- お役立ち記事
- Mechanism of image recognition technology using deep learning, application to object detection, and “Explainable AI” technology
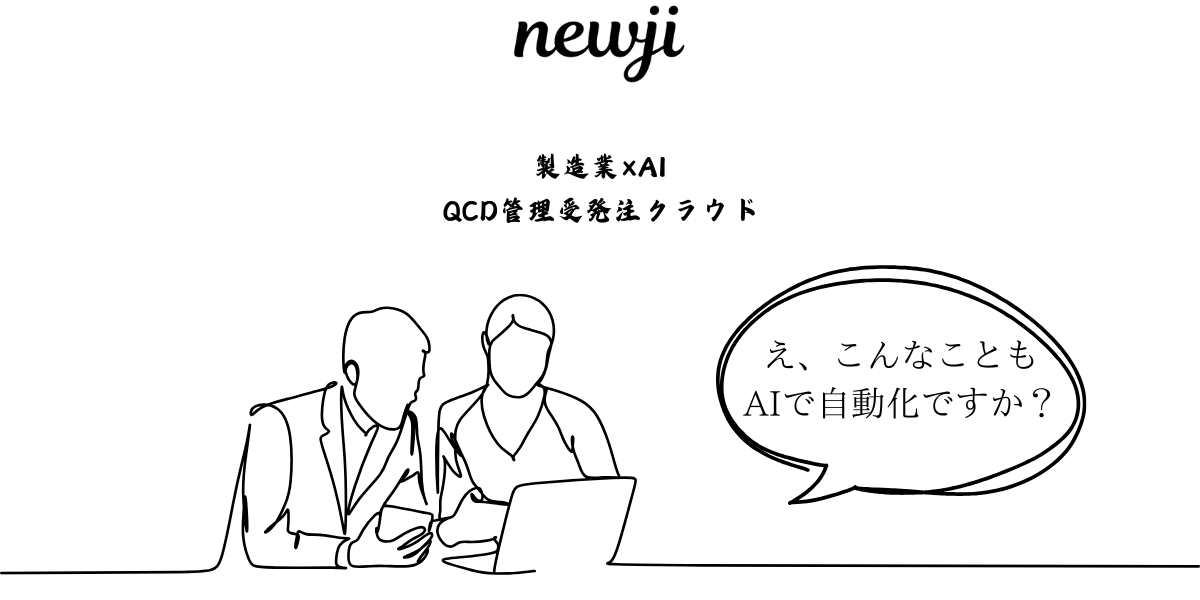
Mechanism of image recognition technology using deep learning, application to object detection, and “Explainable AI” technology
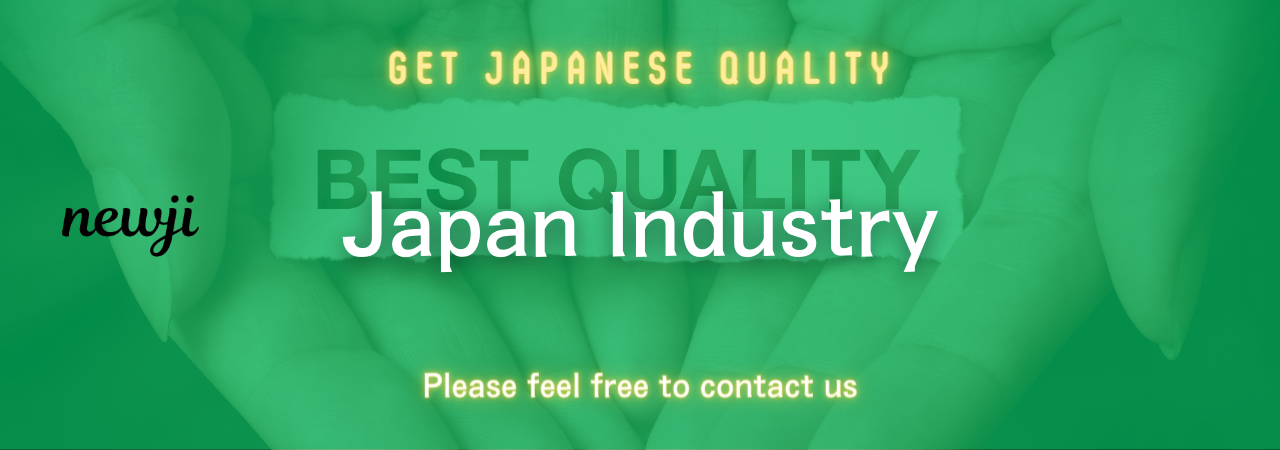
目次
Understanding Image Recognition Technology
Image recognition technology has come a long way, mainly due to advancements in deep learning.
At its core, image recognition involves identifying and processing an image to determine its content.
With the help of machine learning algorithms, computers can now recognize patterns and features within images with impressive accuracy.
Deep learning, a subset of artificial intelligence, mimics the workings of the human brain to process data and create patterns for decision-making.
It uses neural networks with layers (hence the “deep” in deep learning) to analyze various features in images, such as shapes, colors, and textures.
By training on large datasets, these models can discern intricate details, leading to remarkable performance in image recognition tasks.
The Mechanism of Image Recognition with Deep Learning
Deep learning utilizes convolutional neural networks (CNNs), which are particularly effective for image-related tasks.
A CNN typically consists of three primary layers: convolutional layers, pooling layers, and fully connected layers.
Convolutional layers are the first step in processing image data.
They apply filters on the input image, creating feature maps that highlight various attributes like edges or textures.
These filters slide across the image, analyzing small sections at a time, which helps in breaking down complex images into understandable parts.
Pooling layers, usually following convolutional layers, are tasked with down-sampling these feature maps.
Pooling reduces the size, complexity, and computational load by summarizing the presence of features in specific regions.
This ensures the model remains efficient without losing critical information.
Lastly, fully connected layers in the neural network interpret the extracted features and make predictions.
These layers take the pooled feature maps and convert them into a single vector, which is used to classify the image based on learned attributes during training.
Applications in Object Detection
Image recognition doesn’t just stop at recognizing what’s in an image; it extends to detecting and identifying objects within.
Object detection combines image classification and localization to pinpoint where objects are located in an image and what they are.
A significant tool in object detection is the YOLO (You Only Look Once) algorithm.
YOLO can predict multiple bounding boxes and class probabilities for those boxes.
This efficiency comes from treating object detection as a regression problem rather than a classification one, enabling real-time analysis.
Applications of object detection are widespread.
In autonomous vehicles, it’s crucial for detecting pedestrians, other vehicles, and obstacles on the road, ensuring safety.
In retail, automated inventory management systems rely on object detection to monitor stock levels and identify trends.
The technology is also utilized in healthcare, where it assists in analyzing medical imagery, contributing to faster and more accurate diagnosis and treatment.
The Role of Explainable AI
As deep learning models become complex, understanding how they arrive at certain conclusions becomes complicated.
This is where Explainable AI (XAI) comes into play.
Explainable AI is the idea that AI systems should provide human-understandable justifications for their decisions.
It aims to bridge the gap between AI’s complex data processing and human interpretability, ensuring that humans can trust and comprehend AI results.
This transparency is crucial, particularly in sectors like healthcare and finance, where decision-making has significant consequences.
For image recognition, XAI can help demystify why certain images are classified a specific way.
For instance, if a model misidentifies an object, XAI tools can highlight which features led to that misclassification.
This information is invaluable for refining models and making them more robust against errors.
Challenges and Future Directions
Despite the advancements, image recognition and object detection using deep learning face several challenges.
Handling varied lighting conditions, different angles, and unique object orientations can confuse models trained on idealized datasets.
Moreover, concerns about data privacy and ethical use of image recognition technologies also present considerable hurdles.
Researchers are working towards overcoming these issues by developing more versatile models capable of generalizing across diverse environments.
Another promising area is federated learning, where models learn from decentralized data sources, enhancing privacy and reducing the risk of data breaches.
The integration of Explainable AI stands to play an increasingly vital role.
As society becomes more reliant on AI technologies, having transparent and accountable systems will be pivotal for continued public trust and adoption.
In conclusion, the mechanism of image recognition and its application in object detection through deep learning holds great promise.
Explainable AI adds a layer of clarity essential for responsible deployment.
As we advance, refining these technologies and addressing challenges will ensure they contribute positively to various fields, improving efficiency, safety, and understanding in our daily lives.
資料ダウンロード
QCD調達購買管理クラウド「newji」は、調達購買部門で必要なQCD管理全てを備えた、現場特化型兼クラウド型の今世紀最高の購買管理システムとなります。
ユーザー登録
調達購買業務の効率化だけでなく、システムを導入することで、コスト削減や製品・資材のステータス可視化のほか、属人化していた購買情報の共有化による内部不正防止や統制にも役立ちます。
NEWJI DX
製造業に特化したデジタルトランスフォーメーション(DX)の実現を目指す請負開発型のコンサルティングサービスです。AI、iPaaS、および先端の技術を駆使して、製造プロセスの効率化、業務効率化、チームワーク強化、コスト削減、品質向上を実現します。このサービスは、製造業の課題を深く理解し、それに対する最適なデジタルソリューションを提供することで、企業が持続的な成長とイノベーションを達成できるようサポートします。
オンライン講座
製造業、主に購買・調達部門にお勤めの方々に向けた情報を配信しております。
新任の方やベテランの方、管理職を対象とした幅広いコンテンツをご用意しております。
お問い合わせ
コストダウンが利益に直結する術だと理解していても、なかなか前に進めることができない状況。そんな時は、newjiのコストダウン自動化機能で大きく利益貢献しよう!
(Β版非公開)