- お役立ち記事
- Feature extraction technology
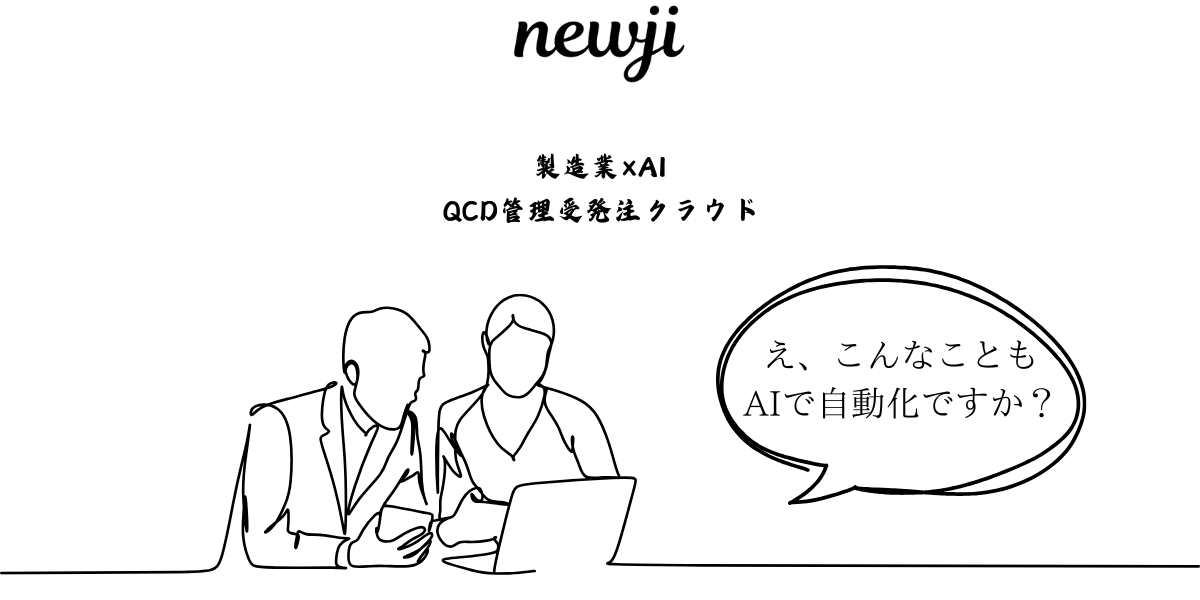
Feature extraction technology
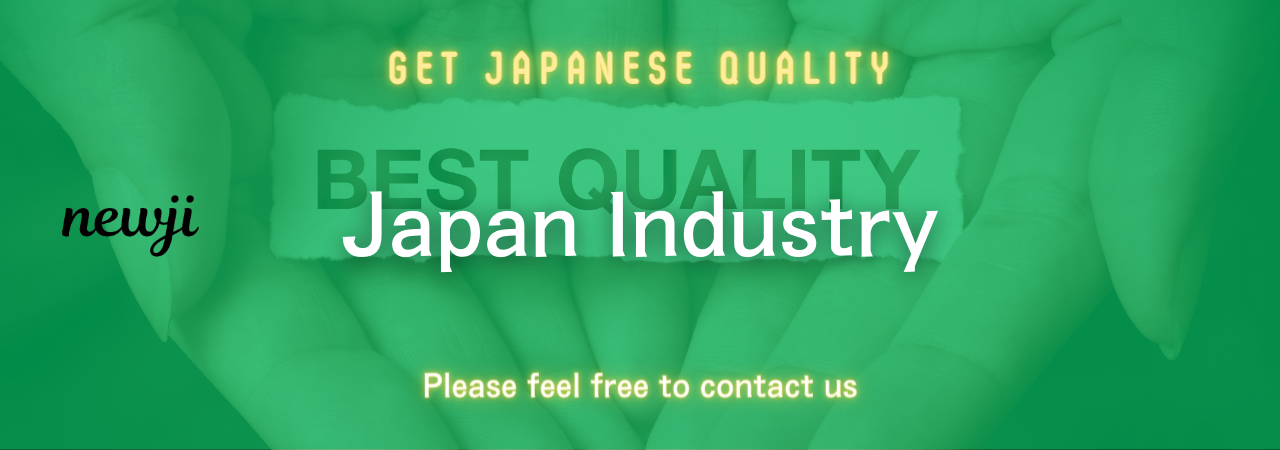
Understanding Feature Extraction Technology
Feature extraction is a critical concept in the realm of data science and machine learning.
It involves the process of transforming raw data into a set of meaningful features that can be used for analysis.
This helps in simplifying the amount of resources needed to describe a large dataset accurately.
In essence, feature extraction constructs combinations of the variables to get around data problems while still describing the data with sufficient accuracy.
Why is Feature Extraction Important?
Feature extraction is vital because it enhances the accuracy and efficiency of data analysis models.
In machine learning, algorithms learn from data.
The better the data, the more insightful the analysis.
Feature extraction focuses on identifying the most relevant attributes from your raw data.
When correctly executed, it reduces data dimensionality and improves the performance of algorithms.
Ultimately, this results in better and faster predictions, which are crucial for decision-making.
How Does Feature Extraction Work?
The process of feature extraction can be divided into several key steps.
Firstly, data collection is necessary.
In this phase, raw data is gathered from various sources, which can include text, images, audio, and other data forms.
Next comes data cleaning.
This involves removing redundant, incomplete, or noisily inconsistent data to enhance its quality.
Once the data is clean, feature extraction begins.
During feature extraction, specific transformations are applied to the raw dataset to create new informative features.
These features allow the data to be represented in a simplified form that is easier for machine learning algorithms to analyze.
Methods to achieve feature extraction include statistical calculations, transforming data into different formats, or using algorithmic techniques like Principal Component Analysis (PCA) and Linear Discriminant Analysis (LDA).
Types of Feature Extraction Techniques
There are numerous techniques for feature extraction, each with unique benefits depending upon the dataset and domain.
Here are some common methods:
Statistical Methods: These involve the use of statistical measures like mean, median, variance, skewness, and kurtosis to derive features from data.
Principal Component Analysis (PCA): PCA helps in reducing the dimensionality of large datasets while preserving as much variability as possible.
It converts the data into new uncorrelated variables called principal components.
Linear Discriminant Analysis (LDA): Like PCA, LDA is used for dimensionality reduction but focuses more on improving the class separability.
Wavelet Transform: Widely used in signal processing, this technique captures both frequency and location information of a signal.
It’s valuable in extracting features from time-series data.
Text Feature Extraction: Techniques such as Term Frequency-Inverse Document Frequency (TF-IDF) and word embeddings like Word2Vec and GloVe convert textual data into numerical formats.
Challenges and Considerations in Feature Extraction
While feature extraction offers significant benefits, it also presents several challenges.
Selecting the right features is critical and often requires expert domain knowledge.
Choosing irrelevant or redundant features can lead to overfitting, where a model performs well on training data but poorly on unseen data.
Feature extraction also demands computational resources.
For large datasets, the process can be time-consuming and computationally expensive.
Moreover, the balance between generalization and specific complexity is delicate.
Overly complex models can memorize rather than learn, while overly simplified models may fail to capture significant patterns.
Hence, finding the right level of abstraction is key.
The Future of Feature Extraction
As data becomes increasingly complex and voluminous with the advent of technologies such as IoT and big data analytics, the need for advanced feature extraction techniques continues to grow.
Artificial Intelligence (AI) and Machine Learning (ML) are expected to drive the evolution of these techniques.
Deep learning approaches, for instance, are already demonstrating success in automating feature extraction.
Convolutional Neural Networks (CNNs) and Recurrent Neural Networks (RNNs) can extract high-level features from images and sequential data, respectively.
Additionally, AutoML, which automates the end-to-end process of applying ML to real-world problems, is likely to further streamline feature extraction.
With these innovations on the horizon, feature extraction technology is poised to become even more sophisticated, reducing the necessity for manual intervention.
Conclusion
Feature extraction technology is indispensable for transforming complex datasets into insightful and actionable data.
It plays a crucial role in ensuring the efficiency and success of machine learning systems.
By simplifying data, identifying key attributes, and enhancing model performance, feature extraction helps in turning raw information into valuable insights.
As technology progresses, we anticipate even more advancements in this field, driven by the need for faster, more accurate, and more automated data processing solutions.
Staying abreast of these developments is crucial for businesses and professionals aiming to leverage data to its fullest potential.
Through continued innovation, feature extraction will undoubtedly help shape the future of data analytics.
資料ダウンロード
QCD調達購買管理クラウド「newji」は、調達購買部門で必要なQCD管理全てを備えた、現場特化型兼クラウド型の今世紀最高の購買管理システムとなります。
ユーザー登録
調達購買業務の効率化だけでなく、システムを導入することで、コスト削減や製品・資材のステータス可視化のほか、属人化していた購買情報の共有化による内部不正防止や統制にも役立ちます。
NEWJI DX
製造業に特化したデジタルトランスフォーメーション(DX)の実現を目指す請負開発型のコンサルティングサービスです。AI、iPaaS、および先端の技術を駆使して、製造プロセスの効率化、業務効率化、チームワーク強化、コスト削減、品質向上を実現します。このサービスは、製造業の課題を深く理解し、それに対する最適なデジタルソリューションを提供することで、企業が持続的な成長とイノベーションを達成できるようサポートします。
オンライン講座
製造業、主に購買・調達部門にお勤めの方々に向けた情報を配信しております。
新任の方やベテランの方、管理職を対象とした幅広いコンテンツをご用意しております。
お問い合わせ
コストダウンが利益に直結する術だと理解していても、なかなか前に進めることができない状況。そんな時は、newjiのコストダウン自動化機能で大きく利益貢献しよう!
(Β版非公開)