- お役立ち記事
- Predictive maintenance using edge AI
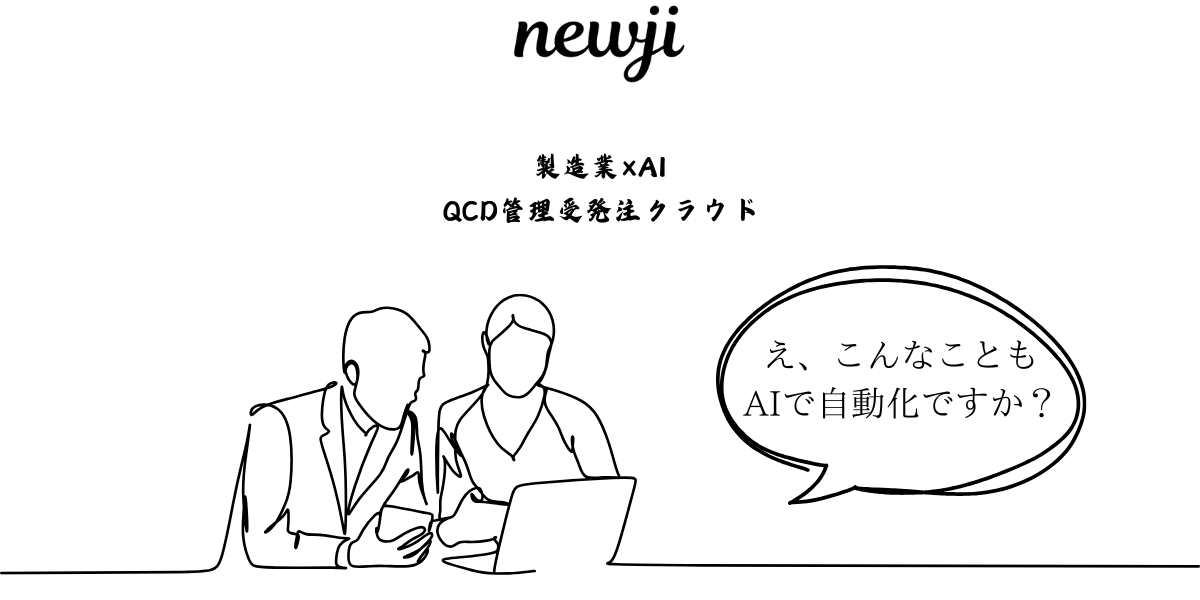
Predictive maintenance using edge AI
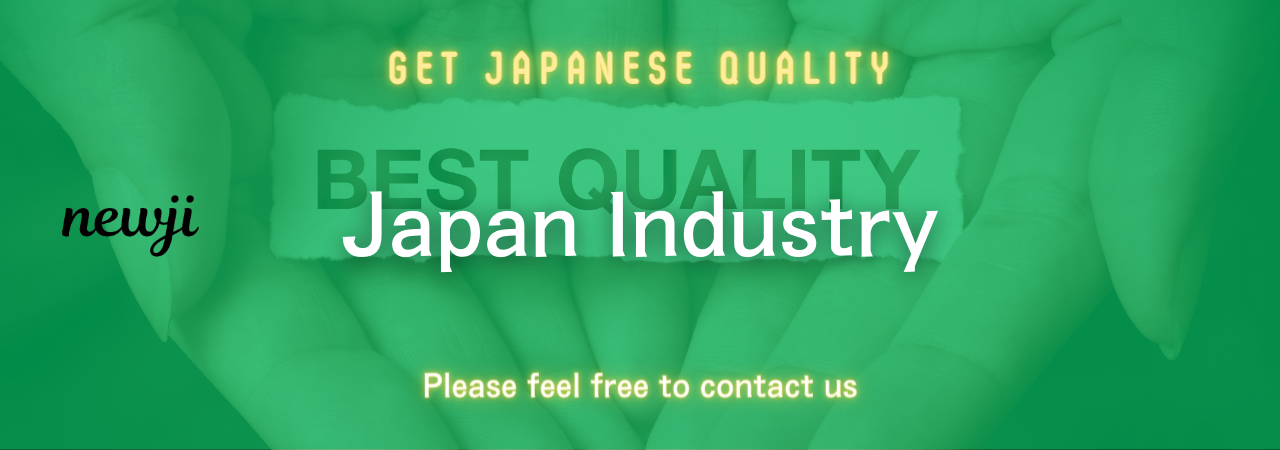
Understanding Predictive Maintenance
Predictive maintenance is a technique that uses data analysis tools and processes to predict when a machine or equipment is likely to fail.
This approach enables businesses to schedule maintenance activities to prevent unexpected breakdowns, which can lead to costly downtimes and repairs.
The goal of predictive maintenance is not just to reduce downtime, but also to extend the life of equipment and improve overall operational efficiency.
The Role of Edge AI in Predictive Maintenance
Edge AI refers to the deployment of artificial intelligence solutions at the edge of a network, close to the data source.
In the context of predictive maintenance, edge AI allows for real-time processing and analysis of data generated by machines and equipment.
By processing data locally, edge AI can provide immediate insights and enable instant decision-making.
For example, if a machine starts to show signs of wear, edge AI can instantly alert maintenance teams to take action before a failure occurs.
Advantages of Using Edge AI for Predictive Maintenance
1. **Real-Time Data Processing**: One of the major advantages of edge AI is its ability to process data in real-time.
This means that businesses can react quickly to any warning signs or anomalies detected in the equipment’s performance.
2. **Reduced Latency**: Edge AI significantly reduces the latency associated with sending data to a central server for processing.
This is crucial in situations where every second counts in preventing equipment failure.
3. **Enhanced Data Privacy and Security**: By processing data at the edge and keeping it local, businesses can better protect their sensitive information.
This reduces the risk of data breaches that can occur during data transmission to cloud servers.
4. **Cost Efficiency**: Predictive maintenance enabled by edge AI can lead to significant cost savings.
By predicting failures before they happen, companies avoid unscheduled downtimes and reduce maintenance costs.
Implementing Edge AI in Predictive Maintenance
Implementing edge AI for predictive maintenance involves several key steps:
1. **Data Collection**: The first step is to gather data from various sensors and devices attached to the equipment.
This data includes temperature, vibration, noise levels, and other relevant metrics that can indicate the health of the machine.
2. **Data Preprocessing**: The collected data must be cleaned and preprocessed to ensure accuracy and reliability.
This step involves removing noise and inconsistencies from the data and converting it into a format suitable for analysis.
3. **Model Training and Deployment**: Using machine learning algorithms, a predictive model is trained on historical data to understand patterns and trends associated with equipment failure.
Once trained, this model is deployed at the edge to continuously monitor real-time data feeds.
4. **Continuous Monitoring and Alerts**: The edge AI system continuously analyzes the incoming data and compares it against the model predictions.
If a potential issue is detected, the system can trigger alerts and initiate preemptive maintenance actions.
Challenges in Using Edge AI for Predictive Maintenance
While edge AI offers numerous benefits, its implementation in predictive maintenance does come with challenges:
1. **Integration with Legacy Systems**: Many industries rely on older equipment that may not be equipped with modern sensors or connectivity features.
Integrating edge AI with such legacy systems can be complex and may require additional investments.
2. **Scalability**: As a business grows and expands, so does its infrastructure.
Scaling edge AI solutions to handle a larger network of equipment can be challenging and requires careful planning.
3. **Data Management**: With the large volumes of data generated by IoT devices and sensors, managing and storing this data effectively is crucial.
Businesses must ensure they have robust data management practices in place.
4. **Cost of Edge Devices**: While edge AI reduces operational costs in the long run, the initial investment in edge devices and sensors can be substantial.
Future of Predictive Maintenance with Edge AI
The future of predictive maintenance with edge AI is promising.
As technology continues to evolve, we can expect even more sophisticated AI models and algorithms that provide more accurate predictions.
Moreover, the proliferation of IoT devices will enhance the capabilities of edge AI solutions.
This will enable more precise monitoring and control of equipment across various industries.
Businesses that embrace edge AI for predictive maintenance will likely see significant improvements in efficiency, reduced downtimes, and cost savings.
In conclusion, the integration of edge AI into predictive maintenance represents a forward-thinking approach for industries looking to optimize their operations.
By embracing this technology, companies can ensure their machinery runs smoothly and maintenance efforts are both timely and cost-effective.
資料ダウンロード
QCD調達購買管理クラウド「newji」は、調達購買部門で必要なQCD管理全てを備えた、現場特化型兼クラウド型の今世紀最高の購買管理システムとなります。
ユーザー登録
調達購買業務の効率化だけでなく、システムを導入することで、コスト削減や製品・資材のステータス可視化のほか、属人化していた購買情報の共有化による内部不正防止や統制にも役立ちます。
NEWJI DX
製造業に特化したデジタルトランスフォーメーション(DX)の実現を目指す請負開発型のコンサルティングサービスです。AI、iPaaS、および先端の技術を駆使して、製造プロセスの効率化、業務効率化、チームワーク強化、コスト削減、品質向上を実現します。このサービスは、製造業の課題を深く理解し、それに対する最適なデジタルソリューションを提供することで、企業が持続的な成長とイノベーションを達成できるようサポートします。
オンライン講座
製造業、主に購買・調達部門にお勤めの方々に向けた情報を配信しております。
新任の方やベテランの方、管理職を対象とした幅広いコンテンツをご用意しております。
お問い合わせ
コストダウンが利益に直結する術だと理解していても、なかなか前に進めることができない状況。そんな時は、newjiのコストダウン自動化機能で大きく利益貢献しよう!
(Β版非公開)