- お役立ち記事
- Inferential statistics and predictive model building
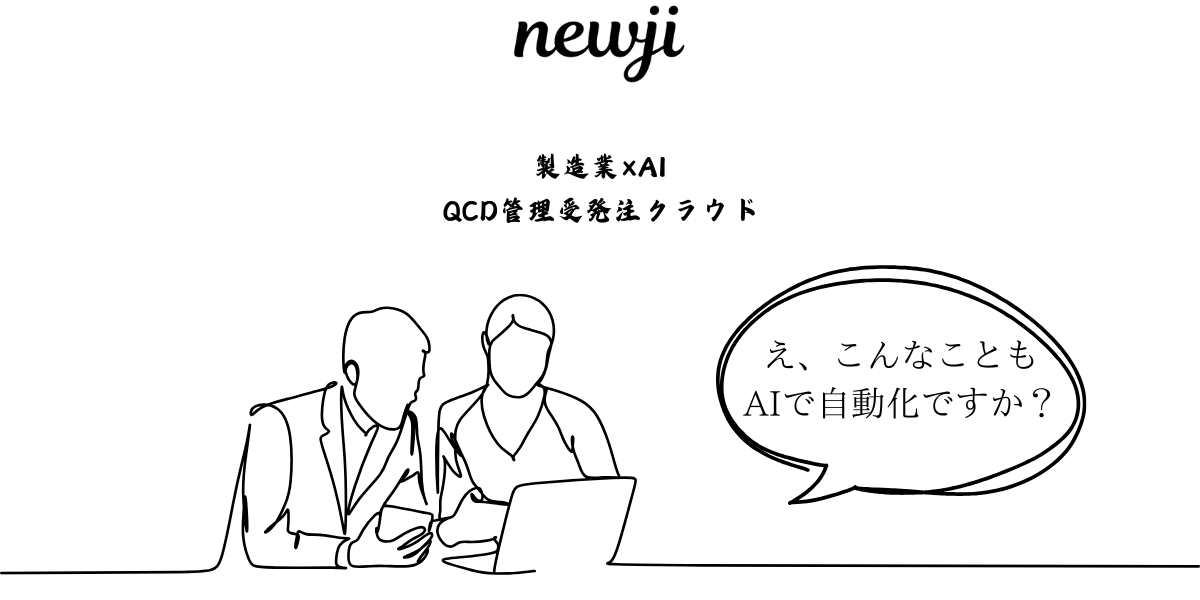
Inferential statistics and predictive model building
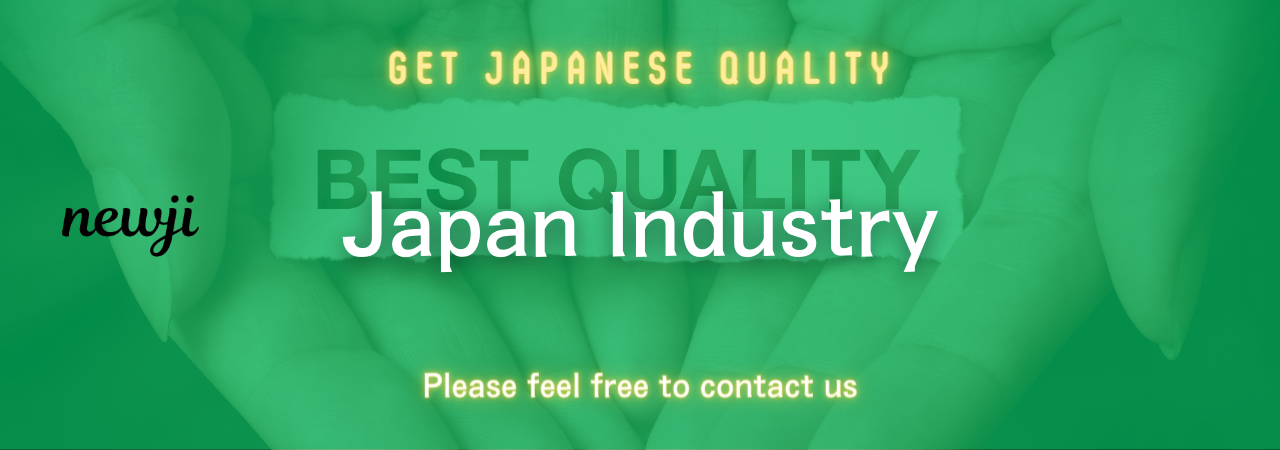
目次
What is Inferential Statistics?
Inferential statistics is a branch of statistics that allows us to make predictions or inferences about a population based on a sample of data drawn from that population.
Unlike descriptive statistics, which aim to summarize the entire dataset, inferential statistics use sample data to estimate broader trends.
This type of statistics helps researchers and analysts determine the probability of characteristics in the population and make decisions based on data analysis.
Key Components of Inferential Statistics
There are several key components that are central to inferential statistics.
First, we have the concept of **samples and populations**.
A population refers to the entire group of interest, while a sample is a subset of that population.
Researchers collect data from the sample to make conclusions about the population.
Next, we have **statistical models and estimates**.
These models are mathematical constructs that relate different variables and predict outcomes.
An estimate is a value derived from a sample used to infer or predict a population parameter.
**Hypothesis testing** is another fundamental aspect.
This involves forming a hypothesis, collecting sample data, and determining whether the data supports the hypothesis or requires rejecting it.
Finally, we have **confidence intervals**, which provide a range of values believed to contain the population parameter.
Confidence intervals allow for an understanding of the precision and reliability of an estimate.
The Importance of Inferential Statistics in Predictive Model Building
Predictive model building relies heavily on inferential statistics to identify patterns, make predictions, and refine models for accuracy.
Without inferential statistics, making coveted predictions from historical data would be nearly impossible.
Identifying Relationships
Before building a predictive model, it is crucial to understand relationships between variables in the dataset.
Inferential statistics help identify correlations and significant predictors through statistical tests like ANOVA and regression analysis.
Making Predictions
Using inferential statistics, we can predict future outcomes based on sample data.
Techniques such as regression analysis model the relationships between independent and dependent variables, thus allowing predictions about future behavior or trends.
Model Selection and Evaluation
Selecting the best statistical model is vital to making accurate predictions.
Inferential statistics provide criteria such as p-values and Akaike Information Criterion (AIC) to evaluate model quality and choose the most effective one.
Steps to Build a Predictive Model Using Inferential Statistics
Build predictive models efficiently by following these steps, incorporating inferential statistics into the process.
Step 1: Define the Problem and Gather Data
Start by clearly defining the problem that the predictive model aims to solve or predict.
Then, gather and prepare relevant data, ensuring it represents the population appropriately.
Step 2: Data Cleaning and Preparation
Clean the dataset by removing errors, handling missing values, and ensuring consistency.
Next, preprocess data by normalizing or transforming variables as needed for analysis.
Step 3: Explore Data and Conduct Inferential Analysis
Use exploratory data analysis (EDA) techniques to gain insights and identify patterns within the data.
Conduct inferential statistical analyses to understand relationships and determine significant variables.
Step 4: Select an Appropriate Model
Choose a predictive model based on the problem and data characteristics.
Common models include linear regression, decision trees, and machine learning algorithms.
Step 5: Train the Model
Split the data into training and validation sets.
Use the training data to build the predictive model, allowing the model to learn from the historical patterns.
Step 6: Validate and Test the Model
Validate the model by assessing its performance on the validation dataset.
Use statistical measures like the mean squared error (MSE) or accuracy to evaluate model efficacy.
Step 7: Deploy and Monitor the Model
Once validated, deploy the model for practical application.
Continuously monitor and recalibrate the model to maintain prediction quality over time.
Challenges and Considerations
While inferential statistics are powerful tools for predictive model building, there are challenges and considerations to keep in mind.
Data Quality
The accuracy of predictions depends on the quality of the sample data.
Ensure that data accurately represents the population and is free of biases or errors.
Model Complexity
Complex models can sometimes lead to overfitting, where the model performs well on training data but poorly on new data.
Balance model complexity with simplicity to enhance generalization.
Uncertainty and Variability
All predictive models have inherent uncertainty and variability, which can impact predictions.
Use confidence intervals to express the reliability of prediction estimates.
Ongoing Maintenance
Predictive models are not static and require ongoing updates and maintenance.
Regularly revise models to incorporate new data and reflect changing trends.
In sum, inferential statistics are essential in constructing predictive models that provide valuable insights and forecasts.
By understanding and leveraging these statistical techniques, analysts can make informed decisions that benefit various fields.
資料ダウンロード
QCD調達購買管理クラウド「newji」は、調達購買部門で必要なQCD管理全てを備えた、現場特化型兼クラウド型の今世紀最高の購買管理システムとなります。
ユーザー登録
調達購買業務の効率化だけでなく、システムを導入することで、コスト削減や製品・資材のステータス可視化のほか、属人化していた購買情報の共有化による内部不正防止や統制にも役立ちます。
NEWJI DX
製造業に特化したデジタルトランスフォーメーション(DX)の実現を目指す請負開発型のコンサルティングサービスです。AI、iPaaS、および先端の技術を駆使して、製造プロセスの効率化、業務効率化、チームワーク強化、コスト削減、品質向上を実現します。このサービスは、製造業の課題を深く理解し、それに対する最適なデジタルソリューションを提供することで、企業が持続的な成長とイノベーションを達成できるようサポートします。
オンライン講座
製造業、主に購買・調達部門にお勤めの方々に向けた情報を配信しております。
新任の方やベテランの方、管理職を対象とした幅広いコンテンツをご用意しております。
お問い合わせ
コストダウンが利益に直結する術だと理解していても、なかなか前に進めることができない状況。そんな時は、newjiのコストダウン自動化機能で大きく利益貢献しよう!
(Β版非公開)