- お役立ち記事
- SLAM/position estimation technology in autonomous driving and its application to urban autonomous driving
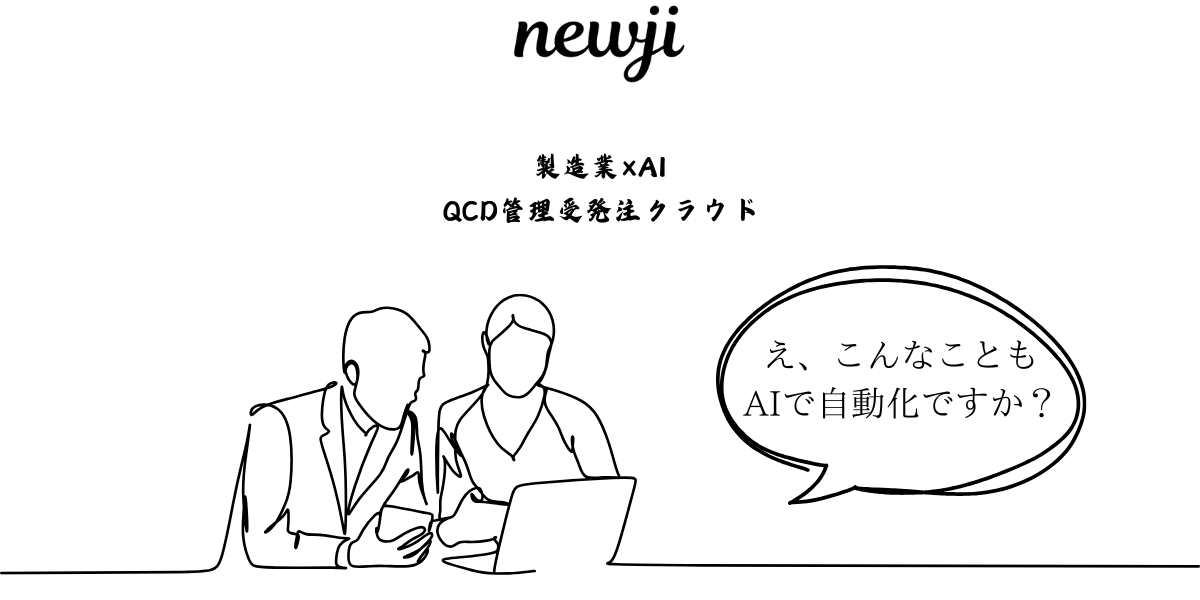
SLAM/position estimation technology in autonomous driving and its application to urban autonomous driving
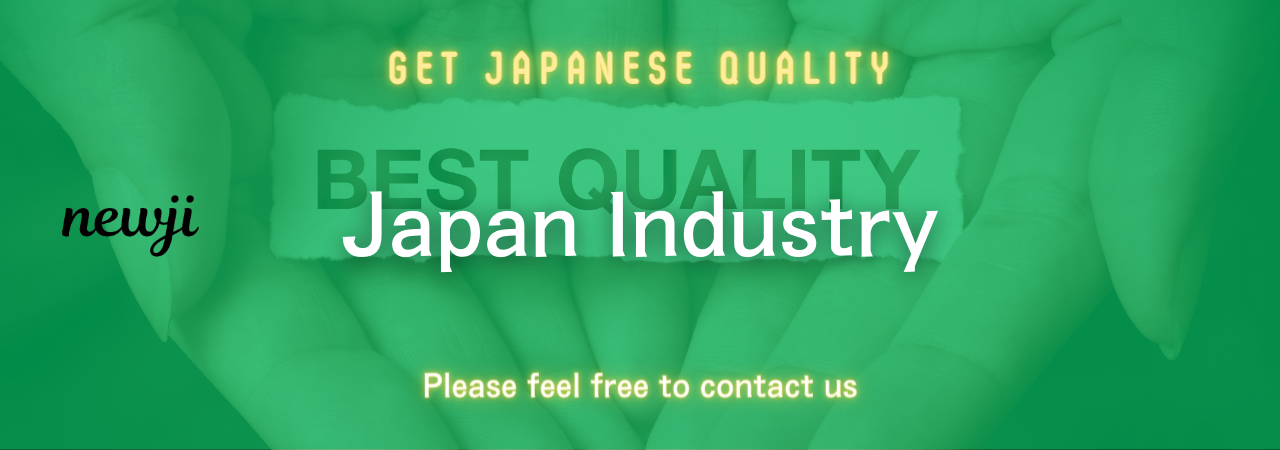
目次
Understanding SLAM Technology in Autonomous Driving
Autonomous driving has been an emerging field in recent years, revolutionizing how we think about transportation.
At the heart of many autonomous systems lies the SLAM (Simultaneous Localization and Mapping) technology.
This is crucial for enabling vehicles to understand their surroundings and determine their positions accurately.
SLAM combines various sensors and algorithms to create an internal map while keeping track of the vehicle’s location.
Let’s explore how this technology works and its application in urban autonomous driving.
How SLAM Technology Works
SLAM is a complex technology that integrates data from multiple sources to establish a map of the surroundings while continuously updating the vehicle’s position.
The process begins with the collection of data from sensors such as LIDAR, cameras, and GPS.
These sensors gather detailed information about the environment, capturing images, distances, and positions.
Once the data is collected, SLAM algorithms process it to create a map of the surrounding area.
Simultaneously, the algorithms pinpoint the exact position of the vehicle within this map.
This simultaneous process of mapping and localization is critical and allows the autonomous vehicle to adapt to dynamic environments efficiently.
Components of SLAM Systems
There are several key components in SLAM systems that work together to ensure accurate localization and mapping.
Firstly, the environment perception sensors, like radars and cameras, provide real-time context about the vehicle’s surroundings.
Next, the pose estimation component uses mathematical models to calculate the vehicle’s position and orientation.
The mapping component creates a structured map based on the perceived data.
Additionally, data fusion ensures that information from all sensors is combined to enhance accuracy.
The loop closure component helps detect previously visited locations, minimizing errors in the map.
SLAM’s Role in Urban Autonomous Driving
In urban environments, autonomous vehicles face unique challenges that SLAM technology can address.
Cities are dynamic spaces with obstacles like pedestrians, cyclists, and other vehicles.
SLAM helps autonomous vehicles navigate these challenging scenarios smoothly.
Obstacle Detection and Avoidance
One of the critical applications of SLAM in urban driving is obstacle detection and avoidance.
Autonomous vehicles must identify and respond to obstacles promptly to ensure the safety of passengers and other road users.
SLAM facilitates this by continuously updating the vehicle’s map, which includes moving obstacles.
The vehicle’s path planning algorithms can then devise safe navigation paths in real-time, avoiding collisions.
Precise Navigation in Complex Road Networks
Urban areas often have complex road networks with intricate intersections, narrow streets, and various road signs.
SLAM technology aids in precise navigation by providing an accurate and updated map of the environment.
This ensures the autonomous vehicle follows the correct routes and can make informed decisions at junctions and traffic signals.
Enhancing Environmental Understanding
Urban areas are abundant with static and dynamic elements, from buildings and trees to traffic lights and crossing pedestrians.
SLAM enhances a vehicle’s understanding of these elements by maintaining a current map and position log.
This awareness is crucial for the vehicle to interpret and predict the movement of surrounding objects accurately.
Future Prospects of SLAM in Autonomous Driving
As technology evolves, SLAM is expected to become even more integral to autonomous vehicles.
The future may see a seamless integration of SLAM with other advanced technologies such as AI and machine learning, enhancing decision-making capabilities.
Higher processing power and improved algorithms will lead to faster data interpretation, making real-time updates more efficient.
Scalability in Smart Cities
The integration of SLAM into smart city networks is an exciting prospect.
Here, SLAM can contribute to shared databases that update across autonomous vehicles, sharing the most current maps and environmental data.
As smart cities develop, vehicles will be able to communicate with infrastructure and each other, heightening safety and efficiency.
Integration with V2X Communication
Vehicle-to-everything (V2X) communication is another area where SLAM technology can play a pivotal role.
By connecting with V2X systems, SLAM-driven vehicles can exchange information with road infrastructure and other vehicles.
This gives them more robust environmental data and enhances situational awareness, supporting safer urban driving.
Conclusion
SLAM/position estimation technology is a cornerstone of the autonomous driving revolution, especially in urban settings.
Its ability to accurately map environments and localize vehicles is essential for navigating complex city landscapes.
SLAM’s role in obstacle avoidance, precise navigation, and enhanced environmental understanding demonstrates its significance in advancing autonomous driving.
As technology progresses, we can anticipate SLAM’s integration into broader intelligent transportation systems, driving towards a future with safer, more efficient urban transportation.
With continuous innovations and advancements, SLAM will undoubtedly remain at the forefront of autonomous driving solutions.
資料ダウンロード
QCD調達購買管理クラウド「newji」は、調達購買部門で必要なQCD管理全てを備えた、現場特化型兼クラウド型の今世紀最高の購買管理システムとなります。
ユーザー登録
調達購買業務の効率化だけでなく、システムを導入することで、コスト削減や製品・資材のステータス可視化のほか、属人化していた購買情報の共有化による内部不正防止や統制にも役立ちます。
NEWJI DX
製造業に特化したデジタルトランスフォーメーション(DX)の実現を目指す請負開発型のコンサルティングサービスです。AI、iPaaS、および先端の技術を駆使して、製造プロセスの効率化、業務効率化、チームワーク強化、コスト削減、品質向上を実現します。このサービスは、製造業の課題を深く理解し、それに対する最適なデジタルソリューションを提供することで、企業が持続的な成長とイノベーションを達成できるようサポートします。
オンライン講座
製造業、主に購買・調達部門にお勤めの方々に向けた情報を配信しております。
新任の方やベテランの方、管理職を対象とした幅広いコンテンツをご用意しております。
お問い合わせ
コストダウンが利益に直結する術だと理解していても、なかなか前に進めることができない状況。そんな時は、newjiのコストダウン自動化機能で大きく利益貢献しよう!
(Β版非公開)