- お役立ち記事
- Basics of reliability data analysis methods and practical points for reliability evaluation
月間77,185名の
製造業ご担当者様が閲覧しています*
*2025年2月28日現在のGoogle Analyticsのデータより
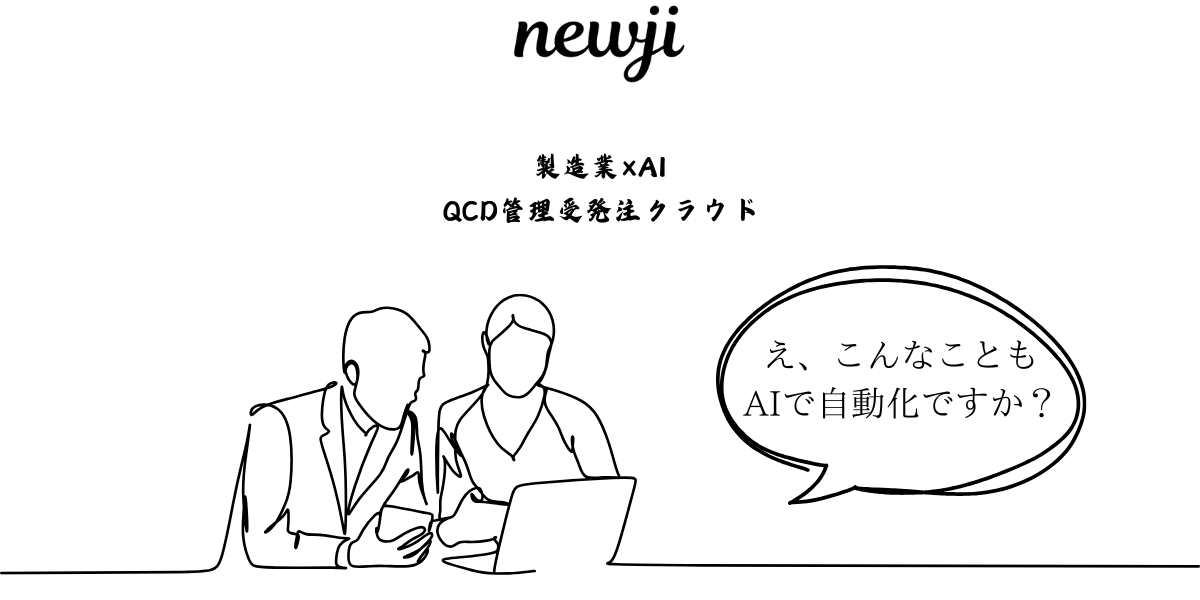
Basics of reliability data analysis methods and practical points for reliability evaluation
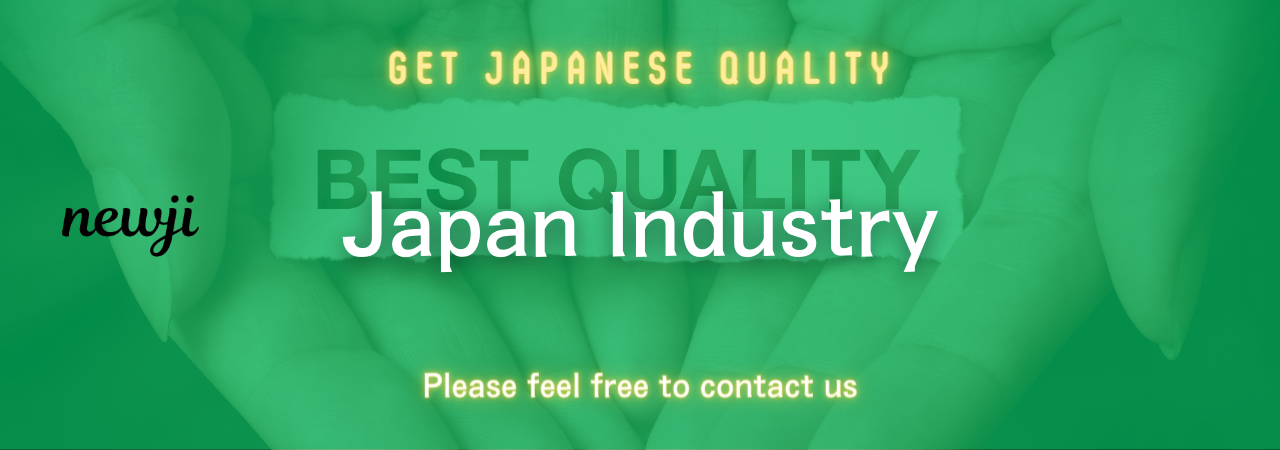
目次
Understanding Reliability Data Analysis
Reliability data analysis is a crucial aspect of assessing the dependability of products and systems.
It involves collecting, analyzing, and interpreting data to determine the lifespan and performance consistency of an item.
Reliability analysis helps engineers and product developers enhance their designs, improve product quality, and reduce the risk of failures.
Why Reliability Analysis Matters
Reliability analysis is paramount in designing products that need to withstand rigorous use over time.
This process ensures that products are trustworthy and perform as expected under various conditions.
Higher reliability can lead to increased customer satisfaction and reduced maintenance costs.
For industries like aerospace, automotive, and healthcare, product failure can lead to catastrophic consequences.
Thus, reliability data analysis is vital for safety and financial reasons as well.
Data Collection for Reliability Analysis
Effective data collection is the foundation for reliability analysis.
Data can be obtained from several sources, including field failure data, maintenance reports, and lab testing.
Field failure data comes from the actual operational environment.
It provides real-life insights into how products perform over time.
Maintenance reports offer information on when and why repairs occur, while lab testing involves subjecting items to stress to predict failure rates.
Types of Reliability Data
Reliability data can be categorized into three main types: time-to-failure data, event data, and condition data.
Time-to-failure data measures how long an item operates before it fails.
Event data tracks occurrences of events leading to failure, and condition data evaluates the state of product characteristics over time.
Each data type requires specific analysis methods to extract meaningful insights.
Fundamental Reliability Analysis Methods
There are several methods used in reliability data analysis, each with unique applications and benefits.
Descriptive Statistics
Descriptive statistics offer a straightforward way to summarize data.
This method includes statistical measures such as the mean, median, variance, and standard deviation.
Descriptive statistics provide a basic understanding of reliability data by identifying central tendencies and data dispersion.
Life Data Analysis
Life Data Analysis, also known as Weibull Analysis, focuses on time-to-failure data.
It helps estimate the product’s life expectancy and reliability over time.
Engineers use it to determine the probability of failure and identify patterns or trends in failure rates.
This method is particularly useful for predicting future performance.
Survival Analysis
Survival analysis is a powerful tool for examining and modeling the expected duration until an event occurs.
This method is essential in industries where understanding the lifespan of a product is critical.
Survival analysis can incorporate censored data, i.e., incomplete data due to items not yet failing or study end before a failure.
Failure Mode and Effects Analysis (FMEA)
FMEA is a proactive technique to identify potential failure modes and their causes.
It assesses the risk associated with these failures and prioritizes them based on severity, occurrence, and detection.
FMEA helps in preventive maintenance planning and design improvements.
Fault Tree Analysis (FTA)
Fault Tree Analysis is a systematic diagram-based method for identifying the root causes of system failures.
It involves constructing a tree-like diagram to visualize the pathways leading to a failure event.
FTA is invaluable for analyzing complex systems with multiple interconnected components.
Implementing Reliability Analysis
To conduct a successful reliability analysis, one must follow specific steps and best practices.
Define Objectives
Starting with clear objectives is crucial.
Determine what you need from the analysis.
Is it to extend product lifespan, reduce warranty claims, or increase customer trust?
Knowing your goals will guide the analysis process effectively.
Select Appropriate Data
Choose suitable data types and sources based on your analysis objectives.
Ensure that the data is accurate, reliable, and representative of real-world conditions.
Use the Right Tools
Utilize statistical software and tools that support reliability data analysis.
These tools can range from general statistical packages like R and Python to specialized software like ReliaSoft.
Draw Conclusions and Take Action
Analyze the results to draw actionable conclusions.
Identify trends, potential failure points, and areas for improvement in your product or system.
Implement necessary changes in the design, manufacturing, or maintenance processes to enhance reliability.
Practical Points for Reliability Evaluation
Reliability evaluation requires attention to detail and adherence to best practices.
Continuous Monitoring
Implement a system for continuous monitoring of product performance.
This process provides ongoing data collection essential for long-term reliability assessments and quick identification of emerging issues.
Interdisciplinary Collaboration
Combine expertise from engineering, statistics, and quality assurance teams.
Collaborative efforts ensure a comprehensive approach to reliability analysis and evaluation.
Feedback Loop
Establish a feedback loop where reliability data informs design improvements.
A consistent update mechanism helps refine products based on actual field performance.
Customer-Centric Approach
Consider customer feedback as part of the reliability evaluation process.
Understanding customer experiences and requirements can enhance the product design and improve satisfaction.
Conclusion
Reliability data analysis is vital for the success and integrity of products and systems.
By understanding and implementing fundamental analysis methods, organizations can enhance their products’ durability and performance.
Adopting these strategies not only leads to financial savings but also builds stronger customer trust and satisfaction.
With continuous advancements in data analysis techniques, the future of reliability evaluation promises even greater accuracy and effectiveness.
資料ダウンロード
QCD管理受発注クラウド「newji」は、受発注部門で必要なQCD管理全てを備えた、現場特化型兼クラウド型の今世紀最高の受発注管理システムとなります。
ユーザー登録
受発注業務の効率化だけでなく、システムを導入することで、コスト削減や製品・資材のステータス可視化のほか、属人化していた受発注情報の共有化による内部不正防止や統制にも役立ちます。
NEWJI DX
製造業に特化したデジタルトランスフォーメーション(DX)の実現を目指す請負開発型のコンサルティングサービスです。AI、iPaaS、および先端の技術を駆使して、製造プロセスの効率化、業務効率化、チームワーク強化、コスト削減、品質向上を実現します。このサービスは、製造業の課題を深く理解し、それに対する最適なデジタルソリューションを提供することで、企業が持続的な成長とイノベーションを達成できるようサポートします。
製造業ニュース解説
製造業、主に購買・調達部門にお勤めの方々に向けた情報を配信しております。
新任の方やベテランの方、管理職を対象とした幅広いコンテンツをご用意しております。
お問い合わせ
コストダウンが利益に直結する術だと理解していても、なかなか前に進めることができない状況。そんな時は、newjiのコストダウン自動化機能で大きく利益貢献しよう!
(β版非公開)