- お役立ち記事
- A practical course on the basics of Bayesian statistics and its effective use in data analysis.
月間77,185名の
製造業ご担当者様が閲覧しています*
*2025年2月28日現在のGoogle Analyticsのデータより
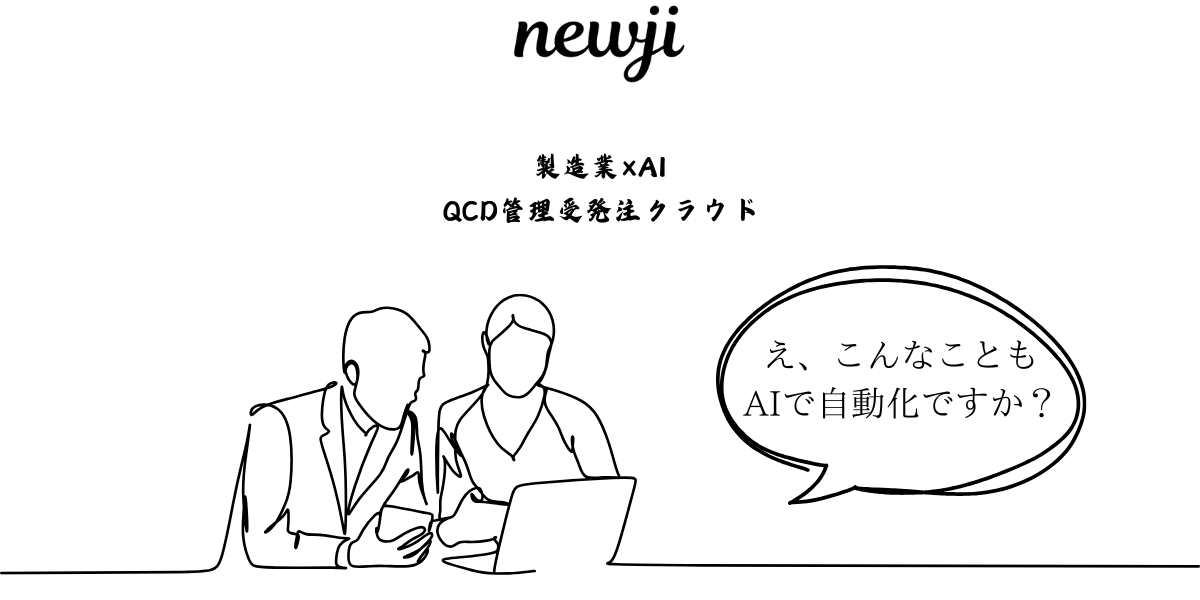
A practical course on the basics of Bayesian statistics and its effective use in data analysis.
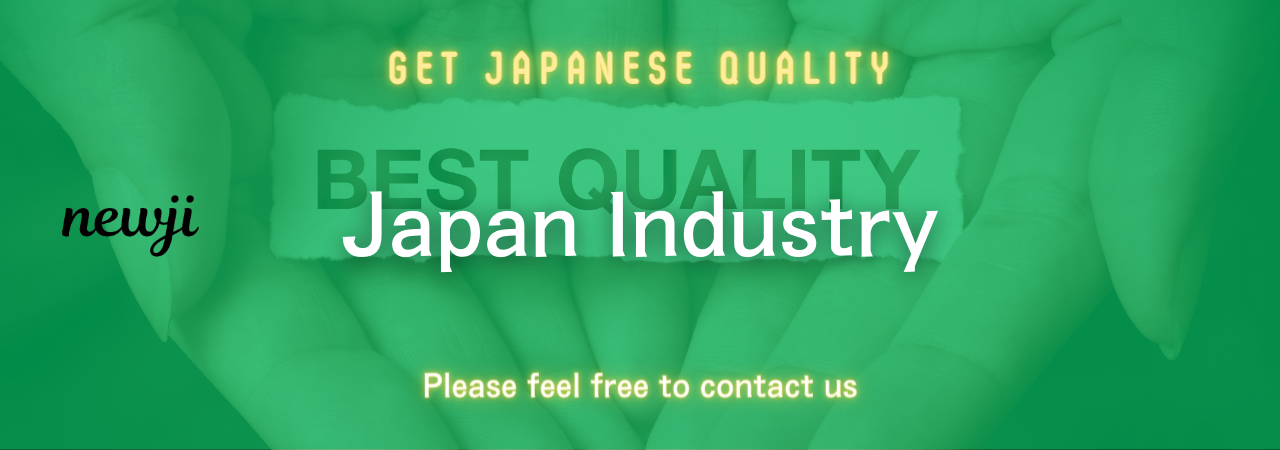
目次
Understanding Bayesian Statistics
Bayesian statistics is a powerful statistical method that has become increasingly popular in the field of data analysis.
Unlike traditional statistical methods, such as frequentist statistics, Bayesian statistics provides a flexible approach to interpreting data by incorporating prior knowledge or beliefs.
This approach allows researchers and analysts to make more informed decisions and predictions based on the available data.
Bayesian statistics is named after the Reverend Thomas Bayes, an 18th-century British mathematician and theologian.
Bayes developed a theorem that laid the foundation for this statistical approach, known as Bayes’ theorem.
Bayesian statistics uses this theorem to update the probability of a hypothesis as more evidence or data becomes available.
Key Concepts in Bayesian Statistics
To effectively understand and apply Bayesian statistics, it’s essential to grasp its key concepts:
Prior Probability
The prior probability represents our initial beliefs about a parameter or hypothesis before observing any data.
This probability is subjective and can be based on previous studies, expert opinions, or other relevant information.
Likelihood
The likelihood is a measure of how well the observed data supports a particular hypothesis.
It is the probability of the data given a specific parameter value.
In Bayesian analysis, the likelihood is combined with the prior probability to update our beliefs and obtain the posterior probability.
Posterior Probability
The posterior probability is the updated probability of a hypothesis or parameter after considering the observed data.
Bayes’ theorem is used to calculate this probability by combining the prior probability and the likelihood.
The posterior probability represents our revised beliefs about the hypothesis in light of the new data.
Bayesian Inference
Bayesian inference is the process of using Bayes’ theorem to update our beliefs about a hypothesis based on observed data.
It’s a fundamental aspect of Bayesian statistics and allows analysts to make probabilistic statements about parameters or hypotheses.
Advantages of Bayesian Statistics
Bayesian statistics offers several advantages over traditional statistical methods, making it a valuable tool in data analysis:
Incorporation of Prior Knowledge
One of the most significant advantages of Bayesian statistics is its ability to incorporate prior knowledge or beliefs into the analysis.
This feature is particularly useful when data is scarce or when experts have valuable insights that can inform the study.
Flexibility
Bayesian statistics is flexible, allowing analysts to update their probabilities as new data becomes available.
This adaptability makes it suitable for a wide range of applications, from scientific research to business decision-making.
Intuitive Probability Interpretation
Bayesian statistics provides an intuitive way to express uncertainty and make probabilistic statements about parameters or hypotheses.
This approach allows for more transparent conclusions and decision-making.
Applications of Bayesian Statistics
Bayesian statistics has a wide range of applications across various fields, highlighting its versatility:
Machine Learning
In machine learning, Bayesian methods are used for tasks such as regression, classification, and clustering.
Bayesian inference helps improve models by incorporating prior knowledge, leading to more accurate predictions.
Medical Research
Bayesian statistics is frequently used in medical research to evaluate the effectiveness of treatments or interventions.
It allows researchers to combine prior studies and data to draw more reliable conclusions about patient outcomes.
Environmental Science
In environmental science, Bayesian methods are employed to model complex systems and assess the impact of different variables on ecosystems.
This approach helps in understanding environmental changes and making informed policy decisions.
How to Effectively Use Bayesian Statistics
Successfully applying Bayesian statistics in data analysis requires a systematic approach:
Define the Problem
Start by clearly defining the problem you want to address and identifying the parameters of interest.
Determine what prior information or beliefs you have about these parameters.
Select a Prior
Choose an appropriate prior probability distribution based on your prior knowledge or beliefs.
Consider the nature of your data and any relevant expert opinions.
Collect and Analyze Data
Gather relevant data, ensuring it is accurate and representative.
Use Bayesian statistical methods to analyze the data and calculate posterior probabilities.
Interpret and Communicate Results
Interpret the results in the context of your problem, and communicate your findings clearly.
Use visualizations and probabilistic statements to convey the uncertainty and implications of your analysis.
Conclusion
Bayesian statistics is a powerful and flexible tool for data analysis that allows researchers to incorporate prior knowledge and update their beliefs with new data.
Its applications are vast, ranging from machine learning to medical research and environmental science.
By understanding its key concepts and following a systematic approach, analysts can leverage Bayesian statistics to make informed decisions and draw meaningful conclusions from their data.
資料ダウンロード
QCD管理受発注クラウド「newji」は、受発注部門で必要なQCD管理全てを備えた、現場特化型兼クラウド型の今世紀最高の受発注管理システムとなります。
ユーザー登録
受発注業務の効率化だけでなく、システムを導入することで、コスト削減や製品・資材のステータス可視化のほか、属人化していた受発注情報の共有化による内部不正防止や統制にも役立ちます。
NEWJI DX
製造業に特化したデジタルトランスフォーメーション(DX)の実現を目指す請負開発型のコンサルティングサービスです。AI、iPaaS、および先端の技術を駆使して、製造プロセスの効率化、業務効率化、チームワーク強化、コスト削減、品質向上を実現します。このサービスは、製造業の課題を深く理解し、それに対する最適なデジタルソリューションを提供することで、企業が持続的な成長とイノベーションを達成できるようサポートします。
製造業ニュース解説
製造業、主に購買・調達部門にお勤めの方々に向けた情報を配信しております。
新任の方やベテランの方、管理職を対象とした幅広いコンテンツをご用意しております。
お問い合わせ
コストダウンが利益に直結する術だと理解していても、なかなか前に進めることができない状況。そんな時は、newjiのコストダウン自動化機能で大きく利益貢献しよう!
(β版非公開)