- お役立ち記事
- Practice of text classification using natural language processing technology and transfer learning using BERT
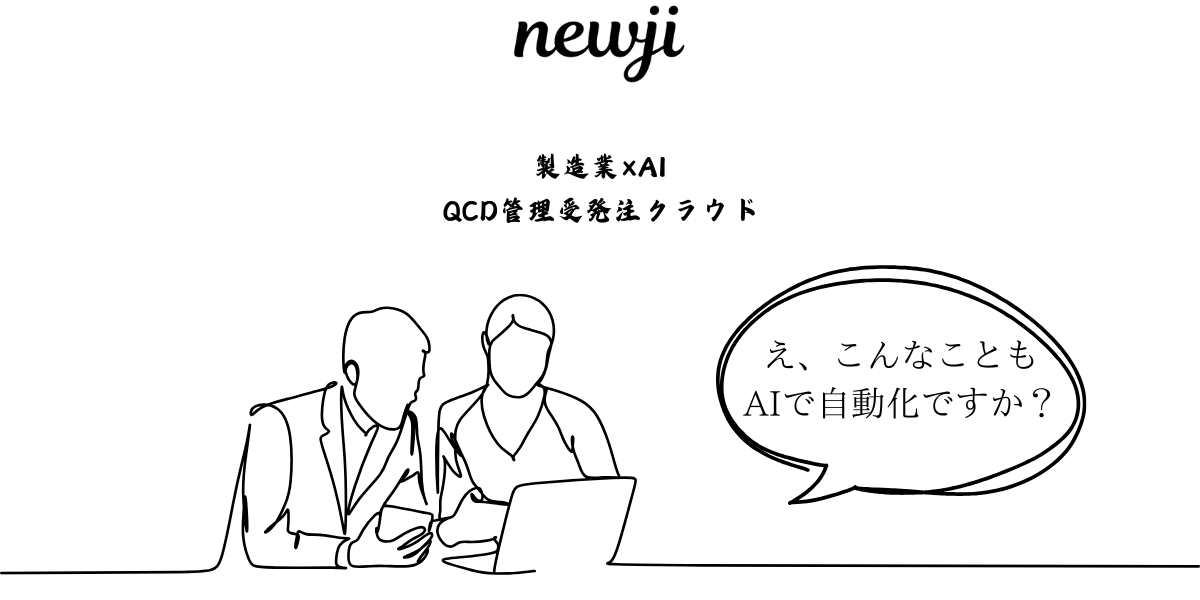
Practice of text classification using natural language processing technology and transfer learning using BERT
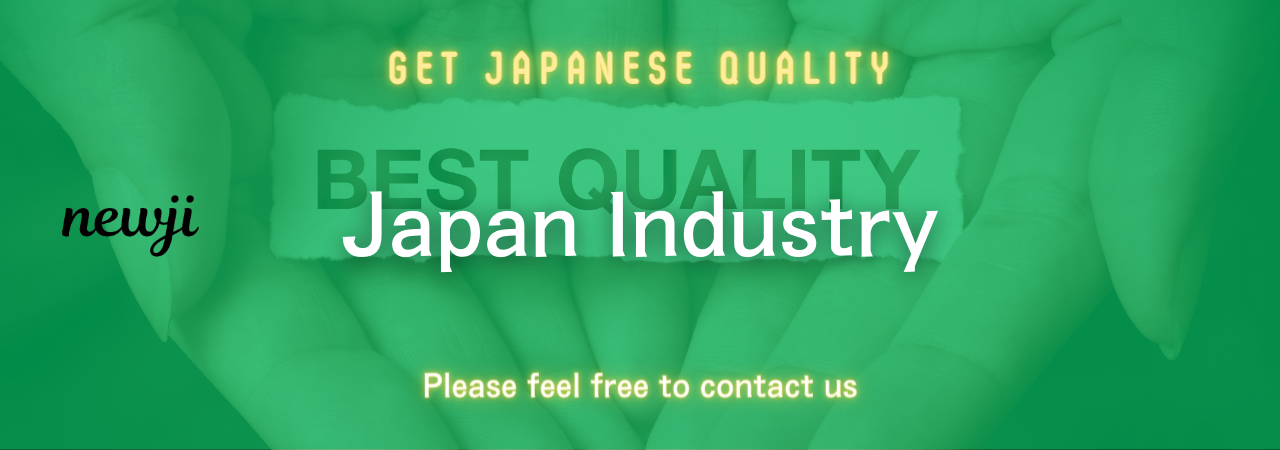
目次
Introduction to Text Classification
Text classification is a fundamental task in natural language processing (NLP) that involves categorizing or labeling text into predefined classes or categories.
Imagine sifting through vast amounts of textual data and being able to accurately sort it into meaningful sections.
That’s the primary goal of text classification—turning a mix of words and sentences into organized, easy-to-understand information.
Why is this important?
Consider the vast sea of data generated every day.
Businesses, researchers, and everyday individuals rely heavily on effective text classification to make sense of this information, whether it be for customer service, sentiment analysis, or sorting through research papers.
Natural Language Processing and Its Role
Natural language processing, or NLP, is a branch of artificial intelligence that focuses on the interaction between computers and humans through natural language.
It’s the technology behind many systems we interact with daily, from voice recognition applications like Siri and Alexa to spam filters and language translation services.
NLP allows computers to read, decipher, understand, and make sense of the human languages in a valuable way.
This is crucial for text classification, as it empowers machines to understand context, sarcasm, sentiment, and various linguistic nuances.
Through NLP, computers can manage tasks such as tokenization (the process of breaking down text into tokens), part-of-speech tagging, and entity recognition, setting the foundation for efficient text classification.
Understanding Transfer Learning
Transfer learning is a machine learning technique where a model developed for a particular task is reused as the starting point for a model on a second task.
In simple terms, it’s like learning to ride a bike and then using some of those skills to learn to ride a motorcycle.
The idea is to utilize knowledge gained from solving one problem, which can then be applied to a different, but related problem.
This approach significantly improves the efficiency and effectiveness of developing models, as it requires less data and computational resources than training a model from scratch.
Transfer learning is particularly effective in NLP because of the vast complexities and nuances within language.
By leveraging pre-trained language models, we can improve the accuracy and performance of tasks related to text classification.
Introducing BERT: Bidirectional Encoder Representations from Transformers
BERT stands for Bidirectional Encoder Representations from Transformers, and it is a NLP model developed by Google in 2018.
BERT revolutionized the way machines understand and process language by introducing a novel method of pre-training language representations.
Unlike previous models that read text input sequentially, BERT reads the entire sequence of words in both directions simultaneously.
This bidirectionality allows it to capture more context and thereby understand the intricacies of language much better.
BERT uses transformers, a kind of NLP architecture, to achieve these impressive results.
Transformers have taken NLP by storm due to their ability to handle the intricacies of language via attention mechanisms, which focus more centrally on the important words and phrases in a sentence.
Practice of Text Classification Using BERT
To start with text classification using BERT, one typically follows these steps:
1. Data Preparation
Firstly, prepare a dataset with text and corresponding labels for the categories you intend to train the model for.
This dataset will guide the BERT model through understanding the differences between various classes.
2. Tokenization
Tokenization is the process of converting the text into a format that BERT understands, typically into tokens or numerical indices.
BERT comes with its tokenizer that handles this process seamlessly and breaks down the text into the required inputs, which include token IDs, segment IDs, and attention masks.
3. Pre-training with BERT
While BERT comes pre-trained, additional pre-training on your domain-specific data helps adapt BERT to the nuances of your specific dataset.
This involves adjusting the BERT model on your prepared data to improve its contextual understanding specific to your task.
4. Fine-tuning
Fine-tuning involves training the pre-trained BERT model on the classification task using your labeled dataset.
This stage is crucial as it allows BERT to make subtle adjustments to the weights learned during pre-training to better classify the specific categories in your task.
5. Evaluation
After fine-tuning, evaluate the model’s performance using a separate set of test data.
This assessment helps ensure that the model can generalize well and hasn’t simply memorized the training data.
Advantages of Using BERT for Text Classification
BERT offers several advantages when used for text classification tasks:
– **Improved Understanding of Context:** Thanks to its bidirectional approach, BERT can pick up on context from both directions, a fact that makes it particularly adept at understanding complex language structures.
– **Fine Grain Attention:** BERT’s attention mechanisms allow it to focus on the important parts of the text, facilitating better prediction and classification accuracy.
– **Transfer Learning Efficiency:** Leveraging transfer learning, BERT can be fine-tuned for specific tasks, reducing the need for vast datasets and long training times.
– **Versatility:** BERT’s architecture is flexible and can be used for a wide range of NLP tasks, from classification to translation and beyond.
Conclusion
Text classification, a key task in natural language processing, becomes significantly more efficient and accurate when utilizing technologies like BERT and transfer learning.
By leveraging the power of BERT, with its advanced contextual understanding and efficient transfer learning capabilities, tasks that once seemed daunting or infeasible become well within reach.
Organizations and researchers are thus empowered to manage and interpret their vast data landscapes with greater precision and insight than ever before.
In a world increasingly driven by data, the importance of deploying effective text classification strategies cannot be overstated.
資料ダウンロード
QCD調達購買管理クラウド「newji」は、調達購買部門で必要なQCD管理全てを備えた、現場特化型兼クラウド型の今世紀最高の購買管理システムとなります。
ユーザー登録
調達購買業務の効率化だけでなく、システムを導入することで、コスト削減や製品・資材のステータス可視化のほか、属人化していた購買情報の共有化による内部不正防止や統制にも役立ちます。
NEWJI DX
製造業に特化したデジタルトランスフォーメーション(DX)の実現を目指す請負開発型のコンサルティングサービスです。AI、iPaaS、および先端の技術を駆使して、製造プロセスの効率化、業務効率化、チームワーク強化、コスト削減、品質向上を実現します。このサービスは、製造業の課題を深く理解し、それに対する最適なデジタルソリューションを提供することで、企業が持続的な成長とイノベーションを達成できるようサポートします。
オンライン講座
製造業、主に購買・調達部門にお勤めの方々に向けた情報を配信しております。
新任の方やベテランの方、管理職を対象とした幅広いコンテンツをご用意しております。
お問い合わせ
コストダウンが利益に直結する術だと理解していても、なかなか前に進めることができない状況。そんな時は、newjiのコストダウン自動化機能で大きく利益貢献しよう!
(Β版非公開)