- お役立ち記事
- Basics of classification technology, tips for classification operations, and troubleshooting
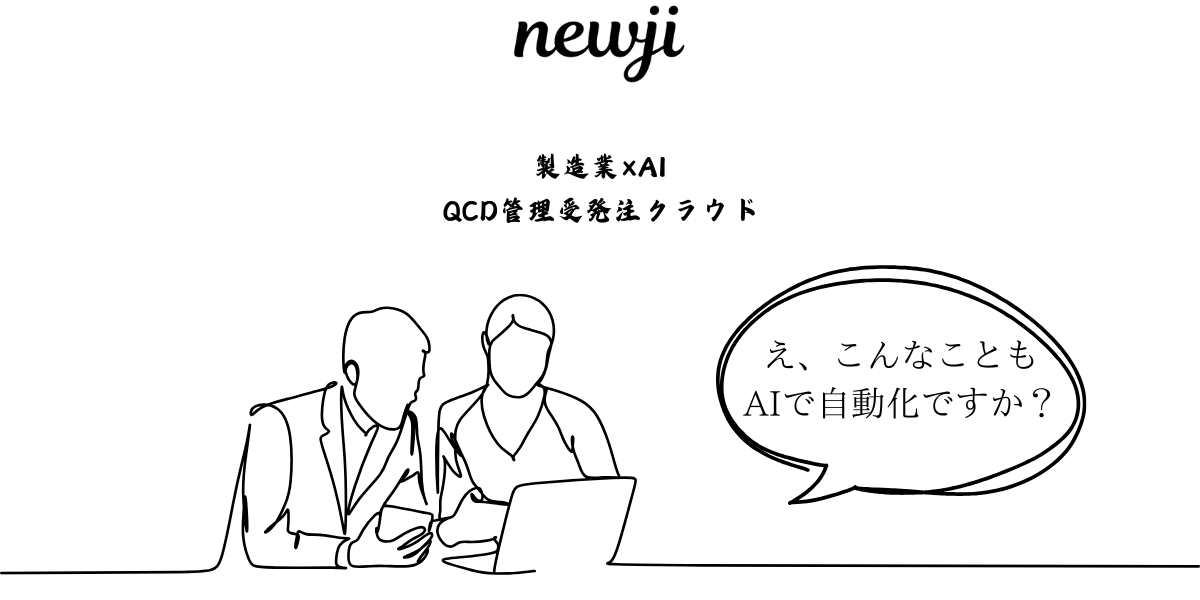
Basics of classification technology, tips for classification operations, and troubleshooting
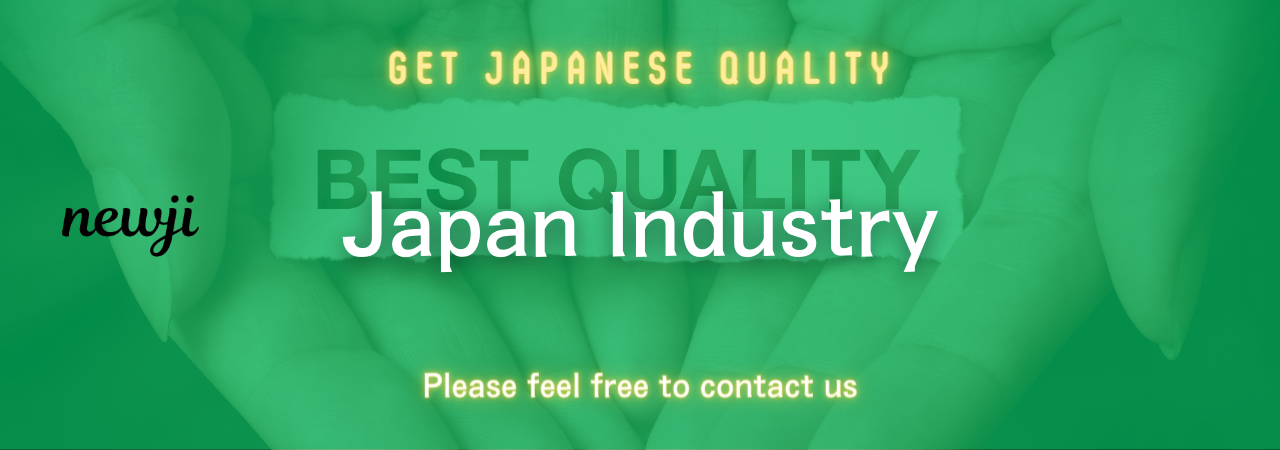
目次
Understanding Classification Technology
Classification technology is a method used to organize data into categories or groups based on specific features or characteristics.
This technology is widely applied in various fields such as machine learning, data analysis, and artificial intelligence.
The purpose of classification is to make data easier to analyze, interpret, and use in real-world applications.
Classification technology utilizes algorithms to identify patterns and trends within datasets.
These algorithms can differentiate between unique features and use them to categorize new data inputs.
By automating the classification process, companies and researchers can save time and resources, all while improving accuracy and reliability.
There are several types of classification algorithms.
Some of the most common ones include decision trees, random forests, support vector machines (SVM), and neural networks.
Each algorithm has its own strengths and weaknesses, and the choice of algorithm depends on factors like the size of the dataset, the type of data, and the desired outcome.
Key Components of Classification Operations
To achieve successful classification operations, several key components need to be considered:
1. **Data Preprocessing**: Before diving into classification, it’s crucial to clean and preprocess the data.
This step involves handling missing values, removing duplicates, and normalizing the data for consistency.
Preprocessing ensures that the data is in the best shape for accurate classification.
2. **Feature Selection**: Feature selection is the process of identifying which attributes or variables will contribute the most to the accuracy of the classification model.
By selecting relevant features, you can reduce the dimensionality of the data, making the model more efficient and easier to interpret.
3. **Model Training**: Once you’ve selected your features, it’s time to train your classification model.
During training, the model will learn the distinguishing characteristics of each class in your dataset.
This step is crucial because a well-trained model can accurately categorize new instances.
4. **Model Evaluation**: After training, it’s essential to evaluate the performance of your classification model.
Common evaluation metrics include accuracy, precision, recall, and F1-score.
These metrics help determine how well your model is performing and if it can generalize to new data.
5. **Model Optimization**: Based on the evaluation results, you may need to optimize your model to enhance its performance.
Techniques such as tuning hyperparameters, adjusting algorithm settings, or experimenting with different algorithms can improve the classification results.
Practical Tips for Classification Operations
Here are some practical tips to enhance classification operations:
1. **Start with Simple Models**: Begin with simpler models like logistic regression or decision trees before transitioning to more complex ones.
Simple models are easier to interpret and can provide quick insights into the data.
2. **Use Cross-Validation**: Implement cross-validation techniques to ensure the robustness of your model.
This approach minimizes overfitting and provides a more accurate assessment of your model’s performance.
3. **Leverage Ensemble Methods**: Ensemble methods such as bagging or boosting can enhance classification results by combining the predictions of multiple models.
Techniques like random forests leverage multiple decision trees to achieve higher accuracy and stability.
4. **Balance Sample Imbalance**: Address class imbalance issues by employing techniques like oversampling, undersampling, or synthetic data generation to ensure that your model doesn’t become biased toward the majority class.
5. **Continuous Monitoring and Maintenance**: Classification models need to be continuously monitored and maintained, especially in dynamic environments where data is constantly changing.
Regular updates and retraining may be necessary to maintain high performance.
Troubleshooting Common Classification Problems
Classification operations may encounter a few common problems.
Here are some troubleshooting tips:
1. **Overfitting**: This occurs when a model performs well on training data but fails to generalize to new data.
Solutions include using regularization techniques, pruning decision trees, or employing cross-validation.
2. **Underfitting**: Underfitting happens when a model is too simple to capture the underlying patterns in the data.
In such cases, consider using more complex models, adding more features, or increasing the model complexity.
3. **High Computational Costs**: Large datasets can lead to high computational costs and strain resources.
To mitigate this, use dimensionality reduction techniques like PCA, and explore the feasibility of cloud-based computing solutions.
4. **Data Quality Issues**: Poor data quality can lead to subpar classification performance.
Always ensure rigorous data preprocessing, error correction, and validation processes to support high-quality input data.
5. **Selecting the Wrong Algorithm**: The choice of algorithm can significantly affect classification performance.
Experiment with different algorithms and validate their performance to ensure you’re using the right one for your specific problem.
Conclusion
Classification technology plays a crucial role in organizing and making sense of vast amounts of data.
By understanding the basics, focusing on key components, and implementing practical tips, you can achieve effective classification operations.
Troubleshooting common issues and continuously monitoring your models will further enhance their performance and reliability.
資料ダウンロード
QCD調達購買管理クラウド「newji」は、調達購買部門で必要なQCD管理全てを備えた、現場特化型兼クラウド型の今世紀最高の購買管理システムとなります。
ユーザー登録
調達購買業務の効率化だけでなく、システムを導入することで、コスト削減や製品・資材のステータス可視化のほか、属人化していた購買情報の共有化による内部不正防止や統制にも役立ちます。
NEWJI DX
製造業に特化したデジタルトランスフォーメーション(DX)の実現を目指す請負開発型のコンサルティングサービスです。AI、iPaaS、および先端の技術を駆使して、製造プロセスの効率化、業務効率化、チームワーク強化、コスト削減、品質向上を実現します。このサービスは、製造業の課題を深く理解し、それに対する最適なデジタルソリューションを提供することで、企業が持続的な成長とイノベーションを達成できるようサポートします。
オンライン講座
製造業、主に購買・調達部門にお勤めの方々に向けた情報を配信しております。
新任の方やベテランの方、管理職を対象とした幅広いコンテンツをご用意しております。
お問い合わせ
コストダウンが利益に直結する術だと理解していても、なかなか前に進めることができない状況。そんな時は、newjiのコストダウン自動化機能で大きく利益貢献しよう!
(Β版非公開)