- お役立ち記事
- Fundamentals of sparse modeling and applications to the exploration and development of new materials and substances using materials informatics
月間77,185名の
製造業ご担当者様が閲覧しています*
*2025年2月28日現在のGoogle Analyticsのデータより
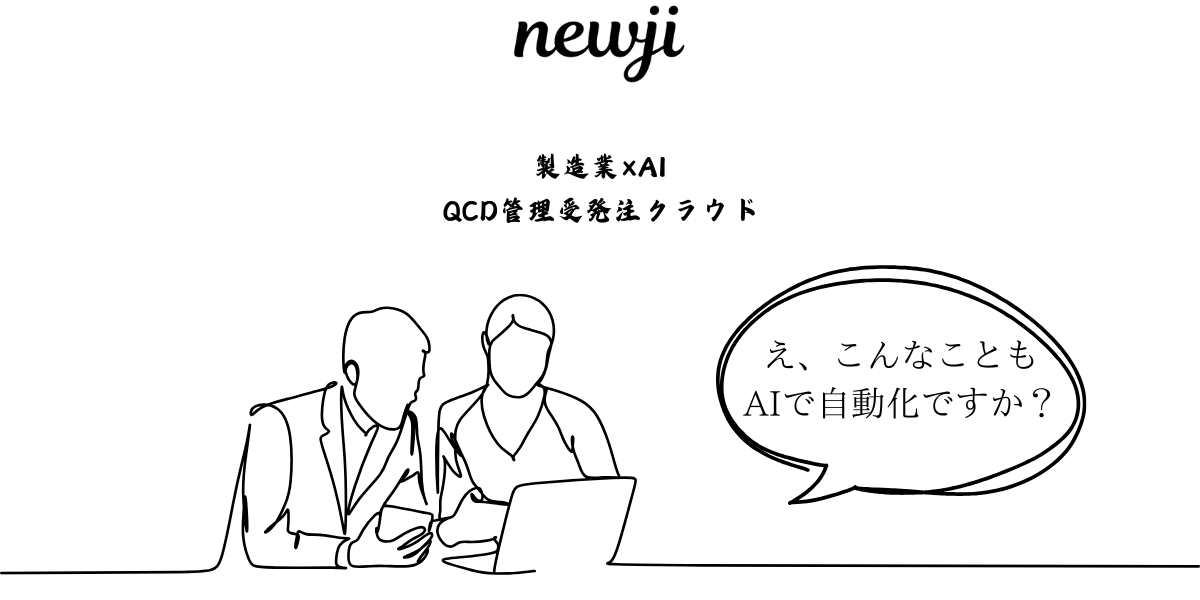
Fundamentals of sparse modeling and applications to the exploration and development of new materials and substances using materials informatics
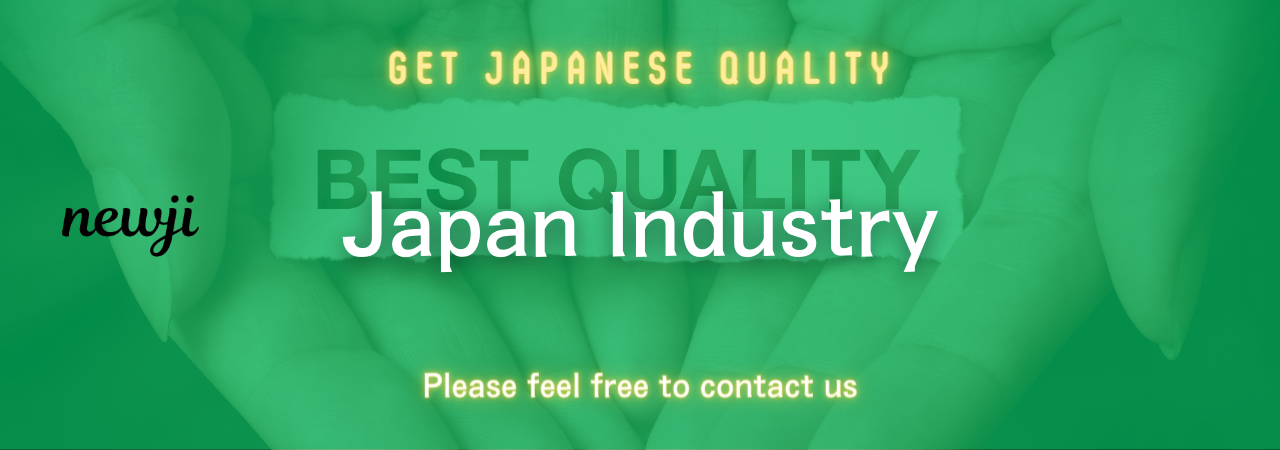
目次
Introduction to Sparse Modeling
Sparse modeling is a powerful mathematical and computational approach designed to find solutions by focusing on simplicity and efficiency.
Unlike traditional methods that may attempt to process all available data, sparse modeling emphasizes identifying the most critical pieces of information.
This technique is particularly valuable in fields like signal processing, image analysis, and statistical data interpretation.
At its core, sparse modeling seeks to represent data in the simplest form possible, using minimal resources while retaining essential information.
This method leverages the assumption that in many real-world datasets, the underlying structure is often simpler than it appears when complete data sets are considered.
For instance, only a few pixels in an image might carry significant information, or just a handful of features in a large dataset might be predictive.
Basic Concepts of Sparse Modeling
Sparse modeling revolves around a few key concepts, including sparsity, basis representation, and regularization.
Sparsity refers to the use of fewer data points or features to describe a phenomenon.
In practical terms, this means relying on models with fewer parameters, making calculations faster and often more accurate.
Basis representation is another critical principle, where data is expressed in terms of a basis that simplifies its structure.
This could mean transforming data into another domain where its sparse nature becomes apparent, such as using Fourier transforms in signal processing.
Regularization is a technique used in sparse modeling to constrain the solution space, preventing overfitting and ensuring that the model remains generalizable to new data.
This often involves incorporating penalties for complexity into the model’s objective function.
Sparse Modeling in Materials Informatics
Materials informatics is an interdisciplinary field that applies data-driven approaches to the exploration and development of new materials and substances.
Sparse modeling plays a pivotal role in this domain by enabling researchers to efficiently sift through vast datasets to discover patterns and correlations that inform material properties and behaviors.
In materials research and development, experiments and simulations generate an enormous volume of data.
Sparse modeling helps manage this data deluge by highlighting the most relevant factors affecting material performance.
Researchers can then focus on these key features, such as atomic structures or chemical compositions, to accelerate the discovery of novel materials.
Applications in Materials Discovery
One application of sparse modeling in materials informatics is in identifying promising candidate materials for specific applications, such as superconductors or high-strength alloys.
By analyzing the vast parameter space that describes material properties, sparse models can predict which combinations might yield the desired characteristics.
For example, sparse modeling can be used to predict the thermal conductivity of materials by selecting only the most impactful parameters from a larger set of variables.
This approach saves time and resources, allowing researchers to zero in on the most promising candidates for further testing.
Accelerating Computational Simulations
Sparse modeling is also instrumental in speeding up computational simulations central to materials informatics.
Traditional methods require significant computational power and time, especially when modeling complex interactions at the molecular level.
With sparse modeling, simulations are made more efficient by approximating these interactions with fewer, tightly-focused parameters.
This reduction in complexity accelerates simulations without sacrificing accuracy, enabling quicker iteration cycles in materials design.
Benefits of Sparse Modeling
The integration of sparse modeling into the process of exploring and developing new materials offers multiple benefits.
Primarily, it improves efficiency by reducing the computational load, enabling researchers to analyze large datasets and simulate complex systems promptly.
Moreover, sparse modeling enhances interpretability.
By focusing on a reduced set of relevant factors, researchers can better understand the underlying phenomena driving material properties.
This clarity aids in the communication of scientific findings and in the formulation of new hypotheses.
Sparse modeling also facilitates the handling of noise within data, a common issue in experimental science.
By using techniques that prioritize significant features while de-emphasizing random variations, sparse models yield results that are more robust and reliable.
Challenges and Future Directions
Despite its benefits, sparse modeling in materials informatics is not without challenges.
One major hurdle is identifying the optimal level of sparsity, which requires a balance between simplicity and capturing essential data complexities.
Too much simplification might overlook critical features, while insufficient sparsity could undermine the efficiency advantages.
Another challenge lies in the need for interdisciplinary expertise.
Successful application often involves collaboration between material scientists, data scientists, and engineers, all contributing their unique skills to leverage sparse modeling effectively.
Looking ahead, the field is poised for exciting advancements with the integration of machine learning techniques, particularly deep learning, which can offer complementary strengths to sparse modeling.
The continued refinement of algorithms and computational methods will further enhance the precision and applicability of sparse modeling in discovering and developing new materials.
Conclusion
Sparse modeling represents a transformative approach in the realm of materials informatics, providing a means to efficiently navigate complex datasets and accelerate material discovery.
Its ability to enhance computational efficiency and clarity makes it an indispensable tool in modern research and development.
As the field progresses, overcoming current challenges and integrating burgeoning technologies will further unlock sparse modeling’s potential, driving innovations in material science.
In this journey, continued collaboration across disciplines will be key to harnessing sparse modeling’s full capabilities to usher in new breakthroughs in material and substance exploration.
資料ダウンロード
QCD管理受発注クラウド「newji」は、受発注部門で必要なQCD管理全てを備えた、現場特化型兼クラウド型の今世紀最高の受発注管理システムとなります。
ユーザー登録
受発注業務の効率化だけでなく、システムを導入することで、コスト削減や製品・資材のステータス可視化のほか、属人化していた受発注情報の共有化による内部不正防止や統制にも役立ちます。
NEWJI DX
製造業に特化したデジタルトランスフォーメーション(DX)の実現を目指す請負開発型のコンサルティングサービスです。AI、iPaaS、および先端の技術を駆使して、製造プロセスの効率化、業務効率化、チームワーク強化、コスト削減、品質向上を実現します。このサービスは、製造業の課題を深く理解し、それに対する最適なデジタルソリューションを提供することで、企業が持続的な成長とイノベーションを達成できるようサポートします。
製造業ニュース解説
製造業、主に購買・調達部門にお勤めの方々に向けた情報を配信しております。
新任の方やベテランの方、管理職を対象とした幅広いコンテンツをご用意しております。
お問い合わせ
コストダウンが利益に直結する術だと理解していても、なかなか前に進めることができない状況。そんな時は、newjiのコストダウン自動化機能で大きく利益貢献しよう!
(β版非公開)