- お役立ち記事
- Basics of anomaly detection technology using deep learning and application to visual inspection
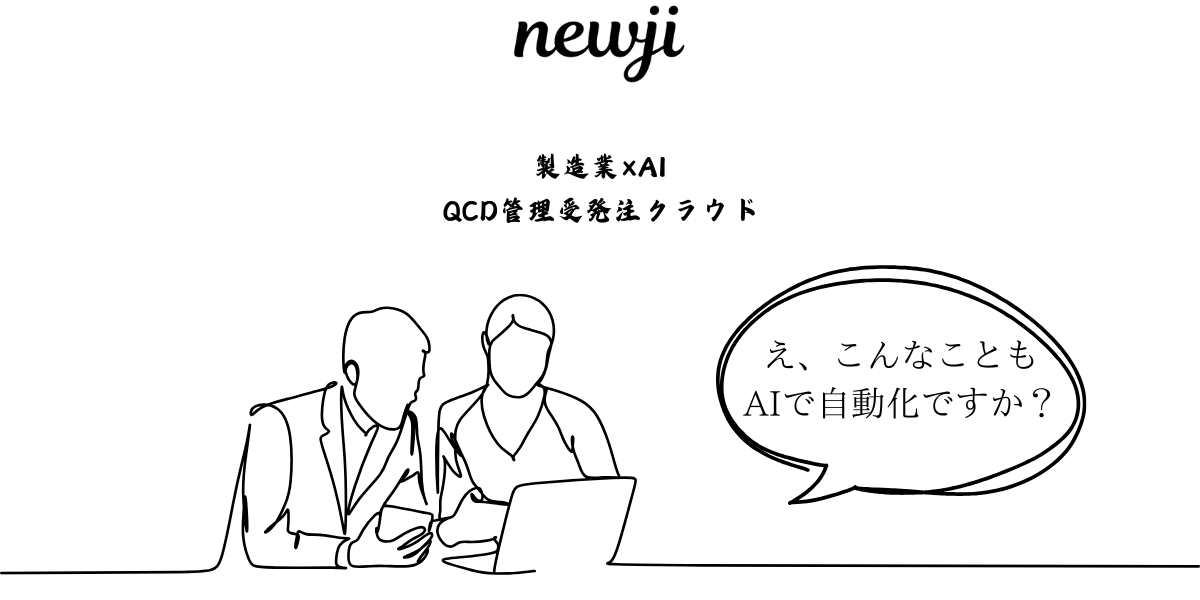
Basics of anomaly detection technology using deep learning and application to visual inspection
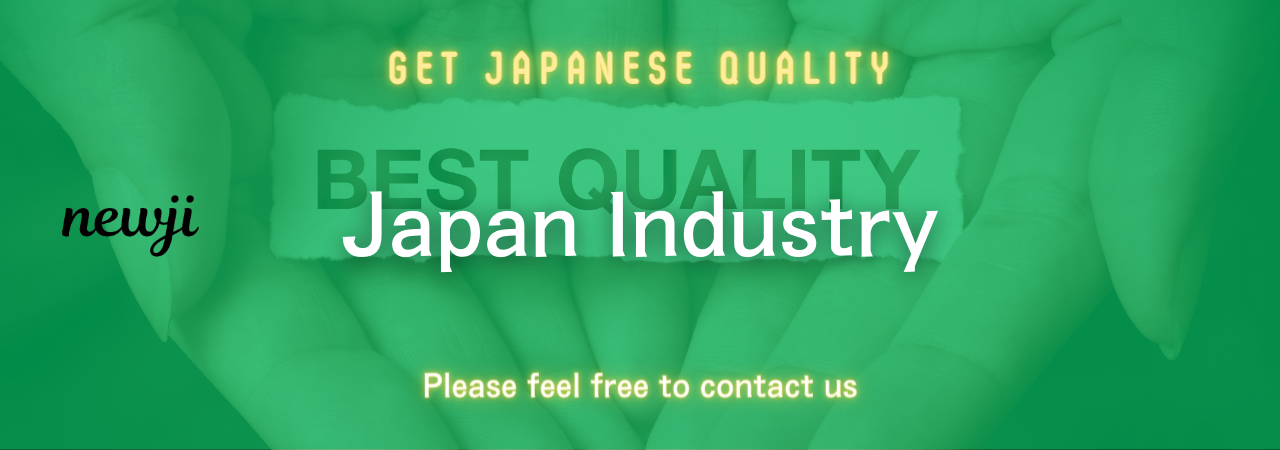
目次
Understanding Anomaly Detection
Anomaly detection is a critical aspect of monitoring and maintaining various systems, ranging from security frameworks to industrial equipment.
In simple terms, anomaly detection refers to the process of identifying patterns or data points that deviate significantly from the expected norm.
These anomalies can indicate anything from potential security breaches to malfunctioning equipment.
In recent years, with the advancement of technology, deep learning has emerged as a powerful tool for improving the accuracy and efficiency of anomaly detection.
The Role of Deep Learning in Anomaly Detection
Deep learning is a subset of machine learning that utilizes neural networks with multiple layers to process and analyze large amounts of complex data.
This makes it particularly effective in identifying patterns that might be too subtle for traditional algorithms to detect.
In the context of anomaly detection, deep learning models can be trained on historical data to learn the normal behavior of a system.
Once trained, these models can then monitor new data and flag any deviations from this learned behavior as potential anomalies.
Applications in Visual Inspection
Anomaly detection using deep learning has found widespread application in the field of visual inspection.
Visual inspection is a quality control process where products are checked for defects or irregularities.
Traditionally, this task has been performed manually by human inspectors.
However, manual inspection can be time-consuming, prone to errors, and inconsistent.
By implementing deep learning models, companies can automate the visual inspection process.
When applied to image data, these models can detect even the smallest defects, such as scratches or misalignments, that might be missed by the human eye.
This not only increases the consistency and reliability of the inspection process but also allows for faster throughput in production lines.
Advantages of Deep Learning in Anomaly Detection
The integration of deep learning into anomaly detection offers several key advantages.
High Accuracy
Deep learning models can achieve high accuracy levels due to their ability to process and analyze vast amounts of data.
This helps in reducing false positives and false negatives, which are common problems in traditional anomaly detection systems.
Adaptability
Deep learning models can adapt and learn from new data, constantly improving their accuracy over time.
This adaptability is critical in environments where normal behavior might change due to evolving conditions or system updates.
Scalability
Deep learning systems can scale effectively to handle increased data volumes, making them well-suited for applications in diverse industries such as healthcare, finance, and manufacturing.
Challenges and Considerations
While deep learning offers significant benefits for anomaly detection and visual inspection, it also presents certain challenges.
Data Requirements
Training a deep learning model requires substantial amounts of labeled data.
Collecting and annotating this data can be resource-intensive and time-consuming.
In cases where anomalies are rare, it is especially challenging to obtain a representative dataset.
Complexity
Deep learning frameworks can be complex to set up and require specialized knowledge for proper implementation.
Organizations must have the necessary expertise in data science and deep learning to effectively deploy these systems.
Compute Resources
Deep learning models typically require significant computational power to train and deploy.
Organizations may need to invest in advanced hardware or cloud computing resources to support their anomaly detection systems.
Future Prospects
The future of anomaly detection using deep learning holds great promise.
Continuous Advancements
As research in deep learning continues to advance, we can expect even more sophisticated models that improve accuracy and efficiency.
These advancements will likely lead to more widespread adoption of deep learning techniques across various industries.
Integration with Other Technologies
We can anticipate the integration of deep learning with other emerging technologies such as IoT (Internet of Things) and edge computing.
This will enable real-time anomaly detection in distributed environments, enhancing the responsiveness and effectiveness of monitoring systems.
Conclusion
Anomaly detection is vital to maintaining the integrity and efficiency of modern systems.
By leveraging deep learning, organizations can significantly enhance their ability to detect and respond to anomalies.
The application of deep learning to visual inspection, in particular, showcases the potential for automation and improved accuracy in quality control processes.
Despite the challenges inherent in implementing deep learning solutions, the benefits are clear.
As technology continues to evolve, we can look forward to even more innovative applications of deep learning in anomaly detection, ensuring safer and more efficient operations across industries.
資料ダウンロード
QCD調達購買管理クラウド「newji」は、調達購買部門で必要なQCD管理全てを備えた、現場特化型兼クラウド型の今世紀最高の購買管理システムとなります。
ユーザー登録
調達購買業務の効率化だけでなく、システムを導入することで、コスト削減や製品・資材のステータス可視化のほか、属人化していた購買情報の共有化による内部不正防止や統制にも役立ちます。
NEWJI DX
製造業に特化したデジタルトランスフォーメーション(DX)の実現を目指す請負開発型のコンサルティングサービスです。AI、iPaaS、および先端の技術を駆使して、製造プロセスの効率化、業務効率化、チームワーク強化、コスト削減、品質向上を実現します。このサービスは、製造業の課題を深く理解し、それに対する最適なデジタルソリューションを提供することで、企業が持続的な成長とイノベーションを達成できるようサポートします。
オンライン講座
製造業、主に購買・調達部門にお勤めの方々に向けた情報を配信しております。
新任の方やベテランの方、管理職を対象とした幅広いコンテンツをご用意しております。
お問い合わせ
コストダウンが利益に直結する術だと理解していても、なかなか前に進めることができない状況。そんな時は、newjiのコストダウン自動化機能で大きく利益貢献しよう!
(Β版非公開)