- お役立ち記事
- Fundamentals of MT systems and machine learning (deep learning) and applications to sensing data analysis and abnormality diagnosis
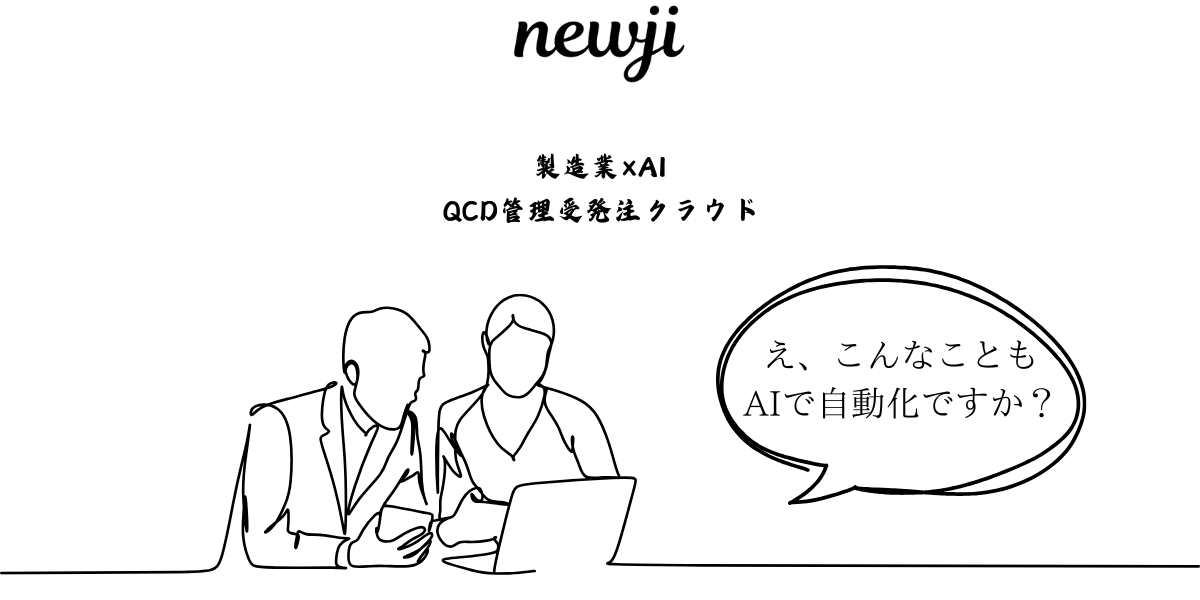
Fundamentals of MT systems and machine learning (deep learning) and applications to sensing data analysis and abnormality diagnosis
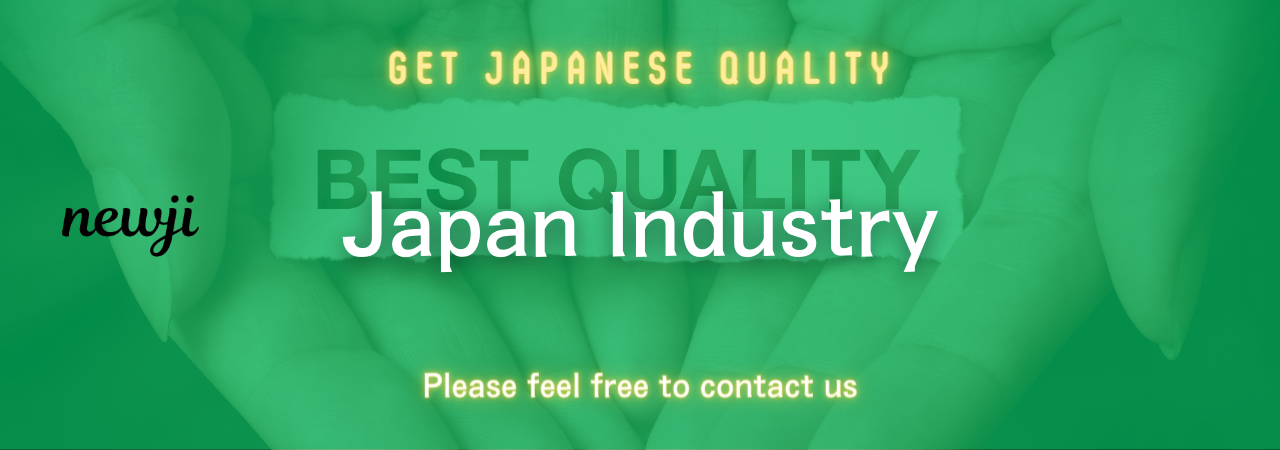
目次
Introduction to Machine Translation Systems and Machine Learning
Machine Translation (MT) systems and machine learning are revolutionizing various industries by automating intricate tasks and enhancing decision-making processes.
MT systems, in particular, utilize artificial intelligence to convert text from one language to another without human intervention.
With the rapid advancement of technology, deep learning—a subset of machine learning—has further catapulted the capabilities of MT systems, expanding their potential applications.
Understanding these systems’ fundamentals and their integration into data analysis and abnormality diagnosis is crucial for leveraging their full potential.
As industries continue to adopt these technologies, it’s essential to grasp how they operate and their applications.
Machine Translation Systems: An Overview
Machine Translation systems first emerged as a means to break language barriers and facilitate communication across different tongues.
Early examples of MT systems relied on rule-based approaches, which required extensive linguistic knowledge and manual creation of rules for each language pair.
However, these systems often struggled with contextual nuances and produced translations lacking fluidity and accuracy.
With advancements in computing and the advent of machine learning algorithms, statistical MT systems became the norm.
These systems utilized vast corpora of bilingual text to generate probabilistic models that predicted the best translation for given segments of text.
However, the real game-changer was the introduction of neural machine translation (NMT), which leverages deep learning to deliver translations that are far more fluent and contextually accurate.
Deep Learning: Powering Modern Machine Translation
Deep learning, a subset of machine learning, involves algorithms structured in layers to simulate human brain functions, often referred to as neural networks.
These networks consist of layers of interconnected nodes or “neurons,” which process input data to produce refined outputs.
In the context of MT, deep learning allows for the parsing and translation of language using vast datasets to learn linguistic patterns and contexts.
NMT systems, powered by deep learning, use an encoder-decoder architecture to break down sentences into smaller units, analyze their relationships, and reconstruct them into the target language.
This structure allows the system to comprehend context better than traditional methods, thus offering translations with improved grammar and a more natural flow.
Key Benefits of Deep Learning in MT Systems
– **Improved Contextual Understanding**: Deep learning models better grasp the context of phrases and sentences, reducing errors in translations.
– **Scalability**: With sufficient data, these models can be adapted to numerous languages with minimal changes.
– **Continuous Learning**: NMT systems are capable of continuous improvements, learning from new data to enhance output over time.
Applications in Sensing Data Analysis
Beyond translation, MT systems and deep learning are increasingly being utilized in sensing data analysis, a crucial component of industries such as healthcare, manufacturing, and environmental monitoring.
Sensing technologies collect vast amounts of data through various devices, requiring sophisticated analysis tools to discern meaningful insights.
Deep Learning Techniques in Sensing Data
By applying deep learning, organizations can process and analyze sensor data more efficiently, leveraging the following techniques:
– **Anomaly Detection**: Algorithms identify patterns or deviations in data that could indicate potential issues or malfunctions.
– **Predictive Analytics**: Models forecast future trends and occurrences based on historical data, aiding in strategic planning and operational efficiency.
– **Feature Extraction**: Techniques that automatically identify significant features from raw data, simplifying complex datasets for easier interpretation.
Advantages of Deep Learning in Sensing Data Analysis
– **Automated Processes**: Reduces the need for human intervention, saving time and resources.
– **Enhanced Accuracy**: Delivers precise analyses by recognizing subtle variations in data.
– **Scalability**: Handles large volumes of data without degradation in performance.
Abnormality Diagnosis Using Machine Learning
In workplaces and healthcare settings, abnormality diagnosis is critical to maintaining operational integrity and ensuring patient safety.
Machine learning, with its ability to process and identify irregularities in data, proves invaluable in this domain.
Implementing Machine Learning for Diagnosis
Machine learning models are trained using historical data, learning to recognize patterns indicative of normal and abnormal conditions.
Once trained, these models can quickly and accurately detect deviations from expected norms, prompting timely interventions.
Benefits of Machine Learning in Abnormality Diagnosis
– **Proactive Interventions**: Enables early detection of issues, allowing for swift corrective actions.
– **Enhanced Reliability**: Reduces the possibility of human error in monitoring and diagnostic processes.
– **Cost Transformation**: Reduces associated costs by preventing major breakdowns and ensuring equipment longevity.
Conclusion
The integration of machine translation systems, deep learning, and machine learning into sensing data analysis and abnormality diagnosis represents a significant technological leap.
By leveraging these advancements, industries can enhance their operational efficiencies, make informed decisions, and improve safety across various contexts.
Staying informed about the nuances of these systems empowers organizations to adopt and innovate with confidence, harnessing their full potential for future growth and development.
As the landscape of technology continues to evolve, understanding the fundamentals of MT systems and machine learning will be increasingly valuable.
資料ダウンロード
QCD調達購買管理クラウド「newji」は、調達購買部門で必要なQCD管理全てを備えた、現場特化型兼クラウド型の今世紀最高の購買管理システムとなります。
ユーザー登録
調達購買業務の効率化だけでなく、システムを導入することで、コスト削減や製品・資材のステータス可視化のほか、属人化していた購買情報の共有化による内部不正防止や統制にも役立ちます。
NEWJI DX
製造業に特化したデジタルトランスフォーメーション(DX)の実現を目指す請負開発型のコンサルティングサービスです。AI、iPaaS、および先端の技術を駆使して、製造プロセスの効率化、業務効率化、チームワーク強化、コスト削減、品質向上を実現します。このサービスは、製造業の課題を深く理解し、それに対する最適なデジタルソリューションを提供することで、企業が持続的な成長とイノベーションを達成できるようサポートします。
オンライン講座
製造業、主に購買・調達部門にお勤めの方々に向けた情報を配信しております。
新任の方やベテランの方、管理職を対象とした幅広いコンテンツをご用意しております。
お問い合わせ
コストダウンが利益に直結する術だと理解していても、なかなか前に進めることができない状況。そんな時は、newjiのコストダウン自動化機能で大きく利益貢献しよう!
(Β版非公開)