- お役立ち記事
- Possibility of utilization and co-creation of cell image classification AI technology
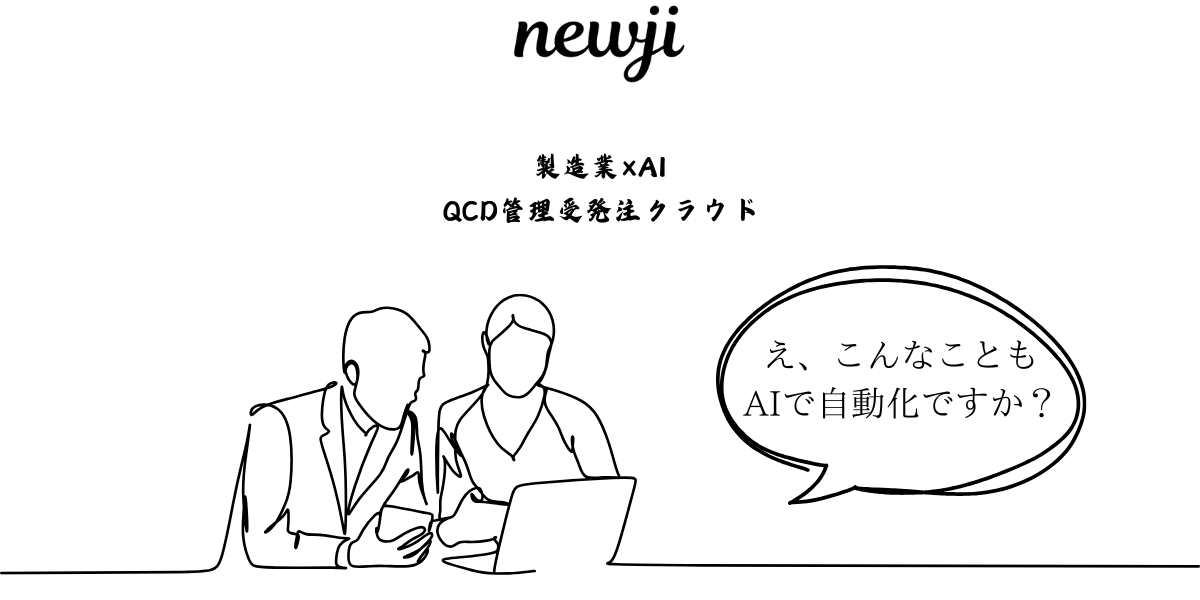
Possibility of utilization and co-creation of cell image classification AI technology
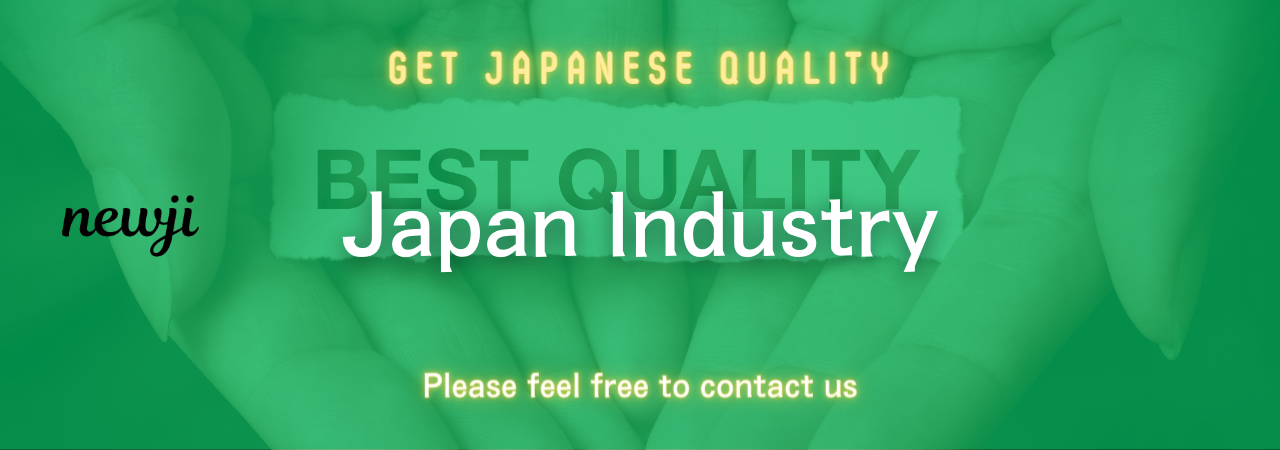
目次
Introduction to Cell Image Classification AI Technology
The advancement of artificial intelligence (AI) technology has opened up numerous opportunities across various fields.
One area that is benefiting significantly from AI is the classification of cell images.
Cell image classification AI technology holds great potential for revolutionizing biological research, medical diagnostics, and pharmaceutical development.
By automating the analysis of cell images, this technology allows scientists and researchers to obtain precise and rapid results.
The Importance of Cell Image Classification
Understanding and analyzing cell images is crucial in numerous scientific and medical disciplines.
Traditionally, this process has been time-consuming and required expert knowledge to ensure accuracy.
AI technology has the potential to minimize the time and effort involved in this process.
It can analyze vast amounts of data at incredible speeds, allowing researchers to focus on more complex tasks rather than spending time on repetitive image analysis.
In the medical field, accurate cell image classification is essential for diagnostics.
It helps in identifying abnormalities within cells that might indicate diseases such as cancer.
By utilizing AI technology, medical professionals can receive assistance in providing more accurate diagnoses, leading to better patient outcomes.
This capability can also significantly enhance the development of personalized medicine, where treatments are tailored to individual patients based on specific cellular abnormalities.
How AI Technology Works in Cell Image Classification
AI algorithms, particularly those in the realm of machine learning, are designed to analyze and classify cell images efficiently.
Machine learning models are trained using vast datasets comprising thousands of cell images.
These models learn to identify different types of cells and distinguish between healthy and diseased cells based on the patterns and features within the images.
Deep learning, a subset of machine learning, often uses convolutional neural networks (CNNs) specifically for image classification tasks.
CNNs can scrutinize cell images layer by layer, detecting subtle features that may not be noticeable to the human eye.
By leveraging GPU-accelerated computing powers, these AI systems can process and analyze images more rapidly than manual methods.
The Advantages of Cell Image Classification AI
One of the primary advantages of utilizing AI technology in cell image classification is enhanced accuracy.
AI algorithms can be trained continuously, improving their accuracy by learning from mistakes and iterating their calculations.
As they encounter more diverse datasets, AI systems become better at recognizing various cell types and conditions.
Additionally, the speed at which AI can process images allows researchers to obtain results almost instantaneously, greatly reducing turnaround time in both research and clinical settings.
AI technology also holds the ability to integrate with other data types.
For example, cell image data can be combined with genetic, proteomic, or clinical data to provide a more comprehensive analysis.
This multi-faceted approach can yield better-informed insights, paving the way for breakthroughs in diagnosis and treatment.
Challenges Facing Cell Image Classification AI
Despite its advantages, cell image classification AI technology still faces several challenges.
Firstly, the need for substantial amounts of high-quality data for training purposes cannot be understated.
Without a robust dataset, the accuracy and reliability of AI models may be compromised.
Secondly, the “black box” nature of some AI systems can be problematic.
Sometimes, AI algorithms make decisions that are difficult to interpret or understand, raising concerns in fields where verification and validation are crucial.
Collaboration between AI experts and domain specialists is necessary to overcome these challenges.
By working together, developers can create AI systems that not only perform well but are also understandable and reliable in practical applications.
Opportunities for Utilization and Co-Creation
The potential applications of cell image classification AI technology are vast, extending beyond research labs and hospitals into areas such as biotechnology, agriculture, and environmental science.
In biotechnology, companies can use AI to enhance drug discovery, high-throughput screening, and even metabolic engineering.
In agriculture, AI could be employed to analyze plant cell images, helping in the development of disease-resistant crops or optimizing the use of fertilizers.
Furthermore, environmental scientists could use this technology to study microorganisms, tracking biodiversity changes and identifying new species.
The concept of co-creation—collaborative innovation—is essential in ensuring that cell image classification technology evolves harmoniously with the needs of various fields.
This means AI developers and target users must collaborate closely from the early stages of development, ensuring that the solutions provided are applicable and impactful.
Case Study: AI in Cell Image Classification
To illustrate the successful utilization of AI in cell image classification, consider a project developed by a renowned research institute.
They implemented an AI-driven system capable of analyzing cancerous cell images, thereby identifying patterns indicative of different cancer types.
Through co-creation, the researchers worked alongside AI experts to develop algorithms that address specific classification challenges they faced in traditional methods.
The result was a system that not only improved diagnostic accuracy significantly but also reduced the time required for comprehensive cell image analysis from hours to mere minutes.
Conclusion
The utilization and co-creation of cell image classification AI technology present tremendous possibilities across multiple disciplines.
As AI continues to become more sophisticated and precise, its potential to transform both scientific research and medical practice is undeniable.
Addressing data quality challenges and fostering collaborative efforts between AI developers and subject-matter experts will be crucial to maximizing the benefits of this technology.
Ultimately, by adopting a collaborative approach, AI technology in cell image classification can become a powerful ally in innovation, delivering insights and solutions that were previously unattainable.
資料ダウンロード
QCD調達購買管理クラウド「newji」は、調達購買部門で必要なQCD管理全てを備えた、現場特化型兼クラウド型の今世紀最高の購買管理システムとなります。
ユーザー登録
調達購買業務の効率化だけでなく、システムを導入することで、コスト削減や製品・資材のステータス可視化のほか、属人化していた購買情報の共有化による内部不正防止や統制にも役立ちます。
NEWJI DX
製造業に特化したデジタルトランスフォーメーション(DX)の実現を目指す請負開発型のコンサルティングサービスです。AI、iPaaS、および先端の技術を駆使して、製造プロセスの効率化、業務効率化、チームワーク強化、コスト削減、品質向上を実現します。このサービスは、製造業の課題を深く理解し、それに対する最適なデジタルソリューションを提供することで、企業が持続的な成長とイノベーションを達成できるようサポートします。
オンライン講座
製造業、主に購買・調達部門にお勤めの方々に向けた情報を配信しております。
新任の方やベテランの方、管理職を対象とした幅広いコンテンツをご用意しております。
お問い合わせ
コストダウンが利益に直結する術だと理解していても、なかなか前に進めることができない状況。そんな時は、newjiのコストダウン自動化機能で大きく利益貢献しよう!
(Β版非公開)