- お役立ち記事
- Fundamentals of data-driven control (FRIT) and application to optimal tuning
月間76,176名の
製造業ご担当者様が閲覧しています*
*2025年3月31日現在のGoogle Analyticsのデータより
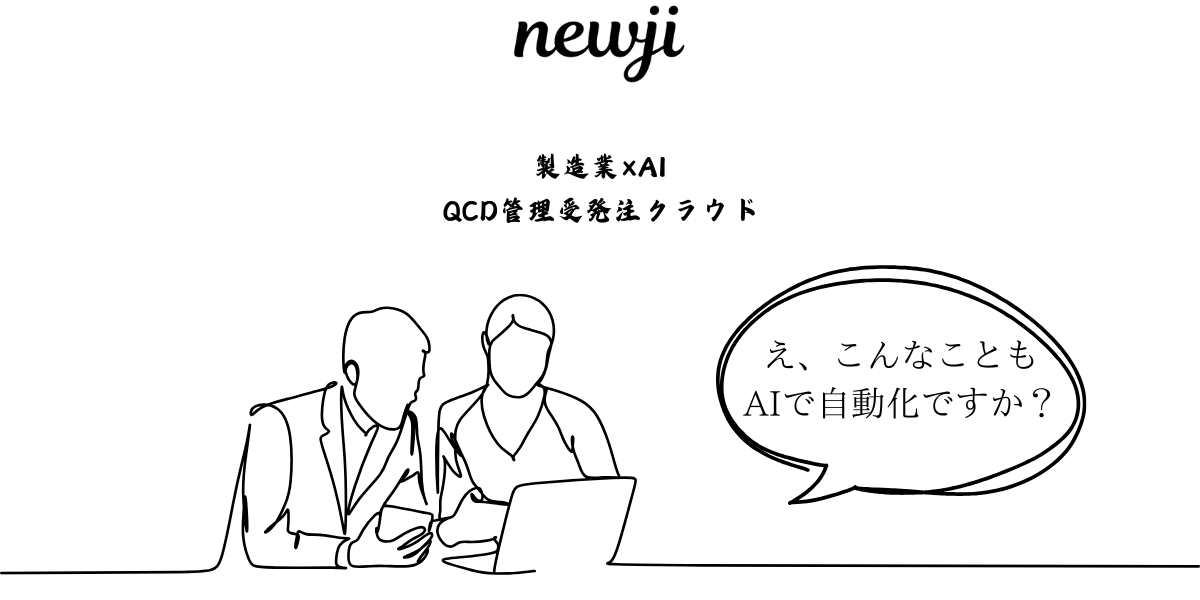
Fundamentals of data-driven control (FRIT) and application to optimal tuning
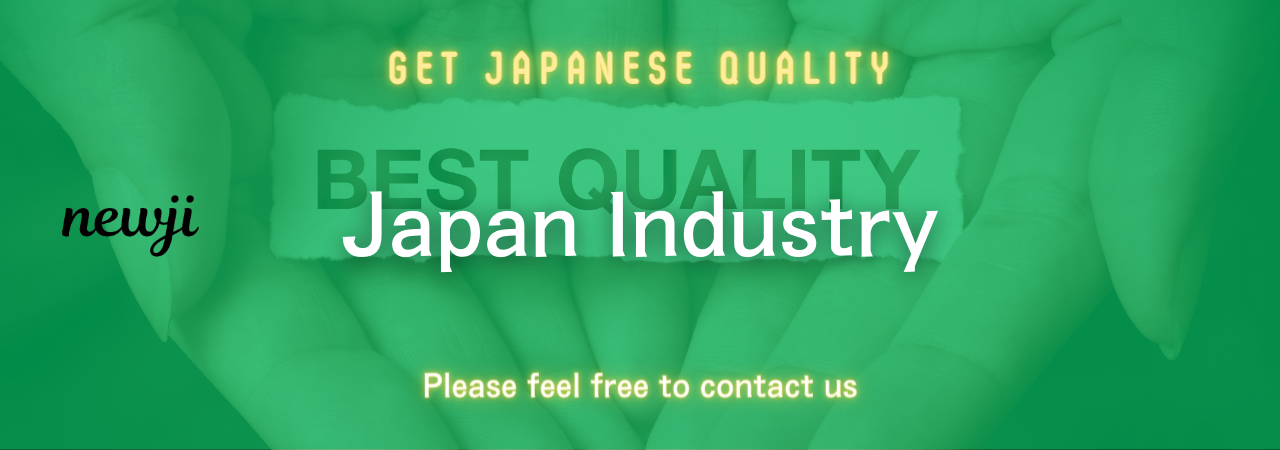
目次
Understanding Data-Driven Control
Data-driven control is an approach that uses data to guide the design and tuning of control systems.
This method is particularly valuable because it doesn’t solely rely on theoretical models, which can sometimes be inaccurate or too complex for practical applications.
Instead, it draws insights and patterns directly from the data collected from system operations.
This data-centric methodology can significantly enhance the robustness and performance of control systems, especially in environments where system dynamics are complex or not fully understood.
Why Data-Driven Control is Important
One of the primary advantages of data-driven control is its adaptability.
Unlike traditional methods that require precise mathematical models, data-driven techniques can function well even when these models are unavailable or incomplete.
This flexibility allows for better control and optimization in real-world applications, such as in manufacturing, aerospace, and automotive industries.
Moreover, data-driven approaches can accommodate changes in system dynamics over time.
As systems evolve, new data can be used to update control strategies without the need for frequent manual interventions.
This capability results in more efficient and reliable systems management.
FRIT: A Game-Changer in Data-Driven Control
FRIT, which stands for Fundamentals of Data-Driven Control, is a cutting-edge approach that exemplifies the strengths of data-driven control.
The methodology is focused on extracting crucial dynamics from system data to design controllers that are both effective and efficient.
FRIT shines in simplifying the complex problem of system tuning by providing optimization tools that leverage historical and real-time data.
This focus enables engineers to refine their control systems with precision, leading to optimized performance.
The Core Principles of FRIT
One of the critical components of FRIT is the identification of relevant data features that directly affect system performance.
By zeroing in on critical variables, FRIT minimizes the risk of information overload, allowing for cleaner and more actionable insights.
Another principle involves iterative learning.
FRIT often employs iterative algorithms that test various control settings against historical data.
Through this trial and feedback process, the system can identify and implement the settings that produce optimal performance outcomes.
Implementing FRIT for Optimal Tuning
To apply FRIT effectively, data acquisition is key.
This involves collecting comprehensive data from the system, which can include sensor readings, operational logs, and environmental factors.
Once the data is collected, it’s crucial to preprocess it to filter out noise and irrelevant information.
After preprocessing, the next step is model-free analysis.
Rather than relying on pre-defined models, FRIT uses machine learning techniques to find patterns and correlations directly from the data.
These insights form the basis for adjusting control strategies.
Practical Applications of FRIT
In practical terms, FRIT can be used across multiple sectors to enhance system efficiency.
In manufacturing, for example, FRIT can streamline production lines by refining the timing and sequence of operations based on data, thereby decreasing downtime and reducing waste.
In autonomous vehicles, FRIT can improve navigation and safety by learning from sensor data about traffic conditions and driver behavior.
This data can then be used to predict and preemptively adjust control outputs, leading to smoother and more responsive vehicle operation.
Challenges and Considerations
Although FRIT presents numerous advantages, certain challenges must be considered.
Data privacy and security are crucial, as sensitive operational data is often involved.
Implementing robust data governance and protection measures are essential to mitigating these risks.
Furthermore, the quality of the control outputs can be highly sensitive to the quality of input data.
Therefore, ensuring that the data used is accurate and relevant is vital for achieving desired outcomes.
Conclusion
Data-driven control, and FRIT in particular, represents a revolutionary shift in how control systems are designed and optimized.
By leveraging the power of data, FRIT enables systems to be more adaptive, resilient, and efficient.
While challenges exist, the potential benefits far outweigh the risks, making it a crucial area for future advancements in technology and industry operations.
As industries continue to innovate, the role of data-driven solutions like FRIT will undoubtedly grow, offering more opportunities to harness data for smarter, more effective control strategies.
資料ダウンロード
QCD管理受発注クラウド「newji」は、受発注部門で必要なQCD管理全てを備えた、現場特化型兼クラウド型の今世紀最高の受発注管理システムとなります。
ユーザー登録
受発注業務の効率化だけでなく、システムを導入することで、コスト削減や製品・資材のステータス可視化のほか、属人化していた受発注情報の共有化による内部不正防止や統制にも役立ちます。
NEWJI DX
製造業に特化したデジタルトランスフォーメーション(DX)の実現を目指す請負開発型のコンサルティングサービスです。AI、iPaaS、および先端の技術を駆使して、製造プロセスの効率化、業務効率化、チームワーク強化、コスト削減、品質向上を実現します。このサービスは、製造業の課題を深く理解し、それに対する最適なデジタルソリューションを提供することで、企業が持続的な成長とイノベーションを達成できるようサポートします。
製造業ニュース解説
製造業、主に購買・調達部門にお勤めの方々に向けた情報を配信しております。
新任の方やベテランの方、管理職を対象とした幅広いコンテンツをご用意しております。
お問い合わせ
コストダウンが利益に直結する術だと理解していても、なかなか前に進めることができない状況。そんな時は、newjiのコストダウン自動化機能で大きく利益貢献しよう!
(β版非公開)