- お役立ち記事
- A must-see for leaders in the R&D department! How to leverage Bayesian statistics to improve product accuracy
A must-see for leaders in the R&D department! How to leverage Bayesian statistics to improve product accuracy
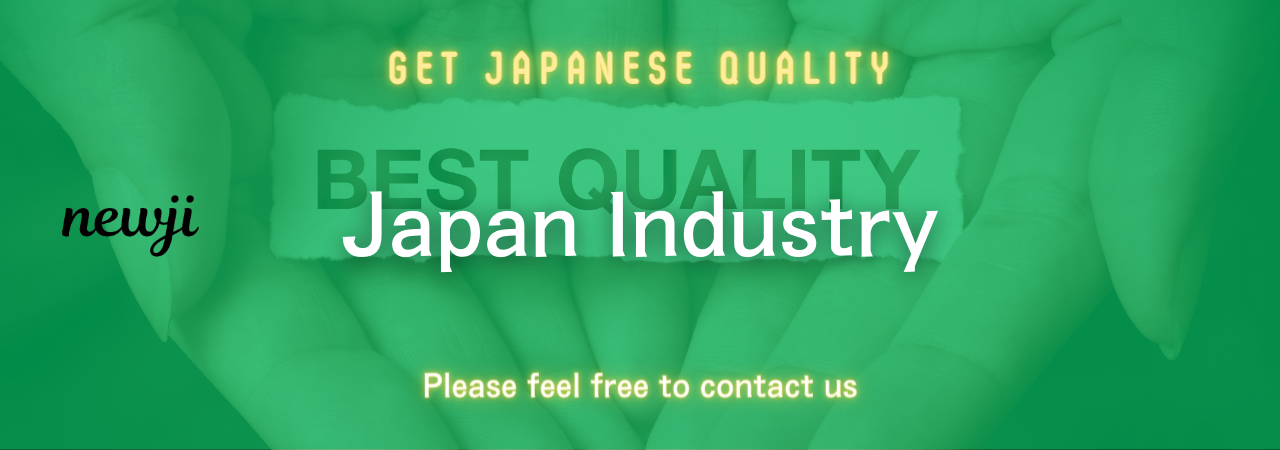
目次
Understanding Bayesian Statistics
Bayesian statistics is a powerful mathematical approach that involves updating the probability of a hypothesis as more evidence or information becomes available.
It serves as a robust framework for dealing with uncertain predictions and complex data analysis, which makes it extremely valuable for research and development (R&D) departments looking to improve product accuracy.
This approach is distinct from traditional statistics, which often involves fixed hypotheses and inference from sampled data.
Bayesian statistics incorporates prior knowledge alongside current evidence, allowing for a more comprehensive probability assessment.
The process revolves around Bayes’ Theorem, a fundamental formula that calculates the conditional probability by inverting the dependence between different conditional probabilities.
Through this, Bayesian methods enable R&D teams to make powerful predictive models and informed decisions despite variability and uncertainty.
Application of Bayesian Statistics in R&D
To leverage Bayesian statistics in R&D, it is essential to understand its practical applications.
R&D departments frequently deal with raw data, ranging from user feedback to sensor readings.
When developing new products or improving existing ones, accurately interpreting this data can lead to significant advancements.
The Bayesian approach facilitates the combination of historical data with new data, ensuring that the predictive models are more dynamic and reliable.
It allows researchers to quantify and incorporate uncertainty directly into the analysis, which is a crucial capability for projects where assumptions change frequently or unforeseen environmental conditions prevail.
Bayesian statistics can aid in:
1. **Product Quality Testing**: By applying Bayesian methods, researchers can determine the probability distribution of various failure modes.
This helps in effectively predicting the life cycle of a product and making necessary design adjustments.
2. **Performance Optimization**: Bayesian models can be used to simulate numerous scenarios, allowing R&D teams to identify the optimal combination of parameters that enhance product performance.
3. **Design of Experiments**: Instead of merely relying on repeated trials, Bayesian statistics can inform better experimental design upfront, reducing cost and time while increasing the reliability of results.
Steps to Implement Bayesian Statistics in R&D
Successfully implementing Bayesian statistics in R&D requires structured steps and strategies. Here’s how leaders can facilitate this process:
1. Cultivate a Statistical Learning Environment
Begin by building a culture that embraces statistical learning and data utilization.
Provide training and resources that enable team members to understand the principles and applications of Bayesian analysis.
Leader engagement is crucial here, advocating for the integration of new methodologies and supporting the team through the transition.
2. Identify the Right Tools and Software
Choosing the appropriate tools is critical.
Several statistical software packages can perform Bayesian analysis, such as R, Stan, and PyMC3.
These platforms offer extensive libraries for constructing Bayesian models and running simulations.
Identify the software that aligns with the team’s expertise and the organization’s technical infrastructure.
3. Gathering and Prepping Data
Quality data is the cornerstone of any statistical analysis.
R&D teams should focus on collecting relevant data, cleaning it, and ensuring it’s accurate before analysis begins.
In the Bayesian framework, both data integrity and availability of prior knowledge are vital for effective analysis.
4. Develop Bayesian Models
Start with simple models and gradually scale up to more complex ones as the team gains experience.
Engage statisticians and experts who are adept at Bayesian networks to consult on model development.
It’s important to iteratively refine models as new insights or data emerges.
5. Validating and Testing
Once the models are in place, it is necessary to validate their predictions.
Compare the Bayesian model predictions with real-world outcomes to fine-tune parameters and improve accuracy.
Testing across different scenarios can also help visualize the model’s robustness and adaptability.
Benefits of Using Bayesian Statistics
Bayesian statistics offer several advantages that are particularly beneficial for R&D departments:
– **Improved Flexibility**: Adapt to changes and new information dynamically, refining models whenever new data is collected.
– **Better Decision Making**: By integrating prior information with current data, leaders can make decisions that are both data-driven and contextually informed.
– **Quantifying Uncertainty**: Manage and quantify uncertainty explicitly, providing higher confidence in modeling predictions.
– **Enhanced Communication**: Communicating results and strategies backed by probabilistic reasoning helps align teams and stakeholders better.
Overcoming Challenges
Adopting Bayesian statistics isn’t devoid of challenges.
One significant hurdle is the steep learning curve associated with Bayesian methods, particularly for teams accustomed to classical statistical approaches.
Additionally, Bayesian analysis can often require significant computational resources.
Investing in advanced computational infrastructure or utilizing cloud-based services can alleviate these constraints.
Collaboration is also essential; continuous learning and cross-departmental sharing of insights can bridge knowledge gaps and accelerate adoption.
Conclusion
In an era where data is ubiquitous yet often overwhelming, Bayesian statistics provide a structured approach for R&D leaders to enhance product accuracy through deeper insights and more adaptive models.
By cultivating the necessary environment and infrastructure, implementing the right strategies, and overcoming initial hurdles, organizations can harness the full potential of Bayesian methods, driving innovation and maintaining a competitive edge.
Leaders at the helm of R&D departments should view Bayesian statistics not merely as a tool, but as an integral part of a broader strategy to effectuate innovation and efficiency.
資料ダウンロード
QCD調達購買管理クラウド「newji」は、調達購買部門で必要なQCD管理全てを備えた、現場特化型兼クラウド型の今世紀最高の購買管理システムとなります。
ユーザー登録
調達購買業務の効率化だけでなく、システムを導入することで、コスト削減や製品・資材のステータス可視化のほか、属人化していた購買情報の共有化による内部不正防止や統制にも役立ちます。
NEWJI DX
製造業に特化したデジタルトランスフォーメーション(DX)の実現を目指す請負開発型のコンサルティングサービスです。AI、iPaaS、および先端の技術を駆使して、製造プロセスの効率化、業務効率化、チームワーク強化、コスト削減、品質向上を実現します。このサービスは、製造業の課題を深く理解し、それに対する最適なデジタルソリューションを提供することで、企業が持続的な成長とイノベーションを達成できるようサポートします。
オンライン講座
製造業、主に購買・調達部門にお勤めの方々に向けた情報を配信しております。
新任の方やベテランの方、管理職を対象とした幅広いコンテンツをご用意しております。
お問い合わせ
コストダウンが利益に直結する術だと理解していても、なかなか前に進めることができない状況。そんな時は、newjiのコストダウン自動化機能で大きく利益貢献しよう!
(Β版非公開)