- お役立ち記事
- A must-see for new employees in the IT management department! How to build a predictive maintenance system using AI and machine learning
A must-see for new employees in the IT management department! How to build a predictive maintenance system using AI and machine learning
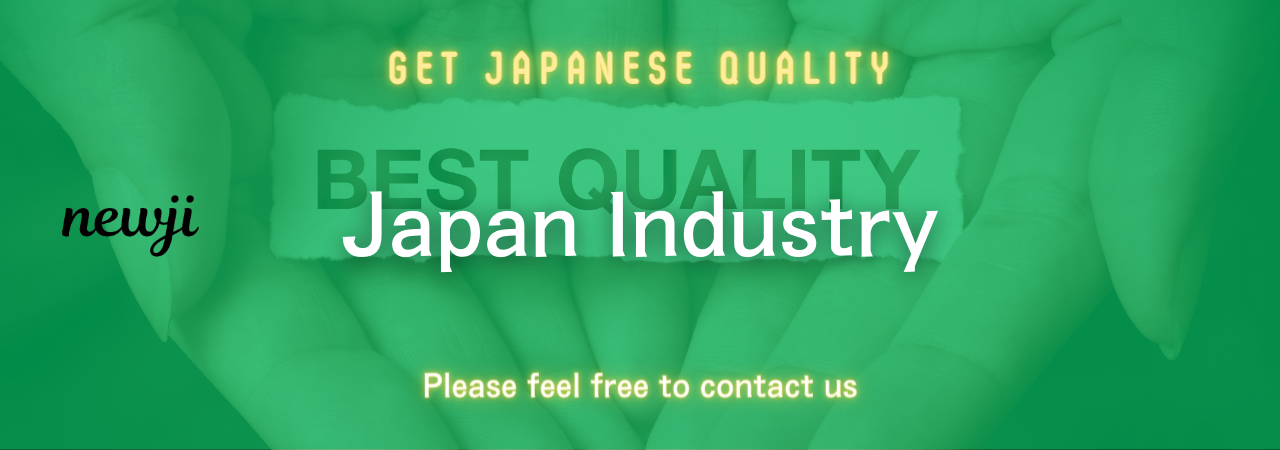
目次
Understanding Predictive Maintenance
Predictive maintenance is a proactive approach that helps predict potential equipment failures before they occur.
This method allows companies to avoid unnecessary downtime, save costs, and improve overall operational efficiency.
Unlike traditional maintenance strategies that rely on time-based schedules, predictive maintenance utilizes data-driven insights to make informed decisions.
Why Predictive Maintenance is Important
For businesses that rely heavily on machinery and equipment, unexpected breakdowns can lead to significant financial losses and disruptions.
By implementing a predictive maintenance system, companies can identify issues early, schedule maintenance during non-peak hours, and extend the life of their equipment.
This approach not only boosts productivity but also enhances safety by reducing the risk of machine-related accidents.
Role of AI and Machine Learning in Predictive Maintenance
Artificial Intelligence (AI) and Machine Learning (ML) are transforming how predictive maintenance is conducted.
These technologies enable the analysis of vast amounts of data collected from equipment sensors and other sources to identify patterns and anomalies.
AI and ML algorithms can predict equipment failures with high accuracy, allowing for timely intervention.
How AI and ML Work Together
AI provides the framework for collecting and processing data, while ML algorithms learn from this data to make predictions.
Machine learning models are trained using historical data to recognize signs of potential failures.
Over time, these models become more accurate and reliable as they analyze new data collected from equipment.
Steps to Build a Predictive Maintenance System
Creating an effective predictive maintenance system involves several key steps.
Data Collection
The first step is collecting relevant data from sensors, logs, and other sources attached to the equipment.
This information serves as the foundation for building predictive models.
It’s important to gather data in real-time to ensure that the system can detect issues promptly.
Data Preprocessing
Once the data is collected, it must be cleaned and preprocessed to remove any inconsistencies or errors.
Data preprocessing involves normalizing, filtering, and transforming data into a format suitable for analysis.
This step is crucial for ensuring the accuracy of predictions.
Feature Engineering
Feature engineering is the process of selecting and transforming input variables to improve the predictive model’s performance.
By identifying the most relevant features, businesses can enhance the model’s predictive power and reduce computation time.
Model Training
In this step, machine learning algorithms are applied to the processed data to train the predictive model.
The model learns to identify patterns associated with equipment failures.
Various algorithms, such as regression, decision trees, and neural networks, can be employed depending on the complexity of the data.
Model Evaluation and Optimization
After training, the model’s performance must be evaluated using test data sets.
Metrics such as accuracy, precision, recall, and F1-score are used to assess model effectiveness.
If the model’s performance is not satisfactory, adjustments are made, which may include tuning hyperparameters or using different algorithms.
Integration with Existing Systems
Once the model is optimized, it can be integrated into the existing IT infrastructure.
Real-time monitoring systems are set up to collect new data and update the predictive model continuously.
This integration allows the maintenance team to receive alerts when potential failures are detected.
Challenges and Considerations
Building a predictive maintenance system is not without challenges.
Data Quality and Volume
High-quality data is essential for accurate predictions.
Data collected from sensors can be noisy or incomplete, making it challenging to build reliable models.
Ensuring that the data is clean and representative of the equipment’s operating conditions is critical.
Algorithm Selection
Choosing the right machine learning algorithms is crucial for performance.
The complexity and nature of the data will determine the algorithm that best suits the predictive maintenance model.
Integration and Scalability
Integrating the predictive maintenance system with existing IT systems requires careful planning.
Furthermore, as the volume of data grows, the system must be scalable to handle increased processing demands without sacrificing performance.
Benefits of Predictive Maintenance
Despite the challenges, the benefits of predictive maintenance are substantial.
Cost Reduction
Predictive maintenance helps reduce costs by minimizing unplanned downtime and optimizing maintenance schedules.
Companies can save on labor and parts expenses by addressing issues before they lead to costly repairs.
Improved Reliability and Efficiency
By accurately predicting equipment failures, businesses can improve the reliability and efficiency of their operations.
This approach ensures that machinery remains operational when needed, maximizing output.
Extended Equipment Life
Regularly maintaining equipment based on predictive insights can extend its lifespan.
This reduces capital expenditures on new machinery and contributes to sustainable business practices.
Conclusion
Building a predictive maintenance system using AI and machine learning is a powerful strategy for enhancing operational efficiency, reducing costs, and extending equipment life.
By following a structured approach to data collection, model training, and system integration, companies can overcome the challenges associated with predictive maintenance.
With ongoing advancements in AI and ML, predictive maintenance systems will continue to evolve, offering even greater accuracy and value for businesses.
資料ダウンロード
QCD調達購買管理クラウド「newji」は、調達購買部門で必要なQCD管理全てを備えた、現場特化型兼クラウド型の今世紀最高の購買管理システムとなります。
ユーザー登録
調達購買業務の効率化だけでなく、システムを導入することで、コスト削減や製品・資材のステータス可視化のほか、属人化していた購買情報の共有化による内部不正防止や統制にも役立ちます。
NEWJI DX
製造業に特化したデジタルトランスフォーメーション(DX)の実現を目指す請負開発型のコンサルティングサービスです。AI、iPaaS、および先端の技術を駆使して、製造プロセスの効率化、業務効率化、チームワーク強化、コスト削減、品質向上を実現します。このサービスは、製造業の課題を深く理解し、それに対する最適なデジタルソリューションを提供することで、企業が持続的な成長とイノベーションを達成できるようサポートします。
オンライン講座
製造業、主に購買・調達部門にお勤めの方々に向けた情報を配信しております。
新任の方やベテランの方、管理職を対象とした幅広いコンテンツをご用意しております。
お問い合わせ
コストダウンが利益に直結する術だと理解していても、なかなか前に進めることができない状況。そんな時は、newjiのコストダウン自動化機能で大きく利益貢献しよう!
(Β版非公開)