- お役立ち記事
- A must-see for new employees in the R&D department! Fundamentals of Bayesian statistics and methods for analyzing experimental data
月間76,176名の
製造業ご担当者様が閲覧しています*
*2025年3月31日現在のGoogle Analyticsのデータより
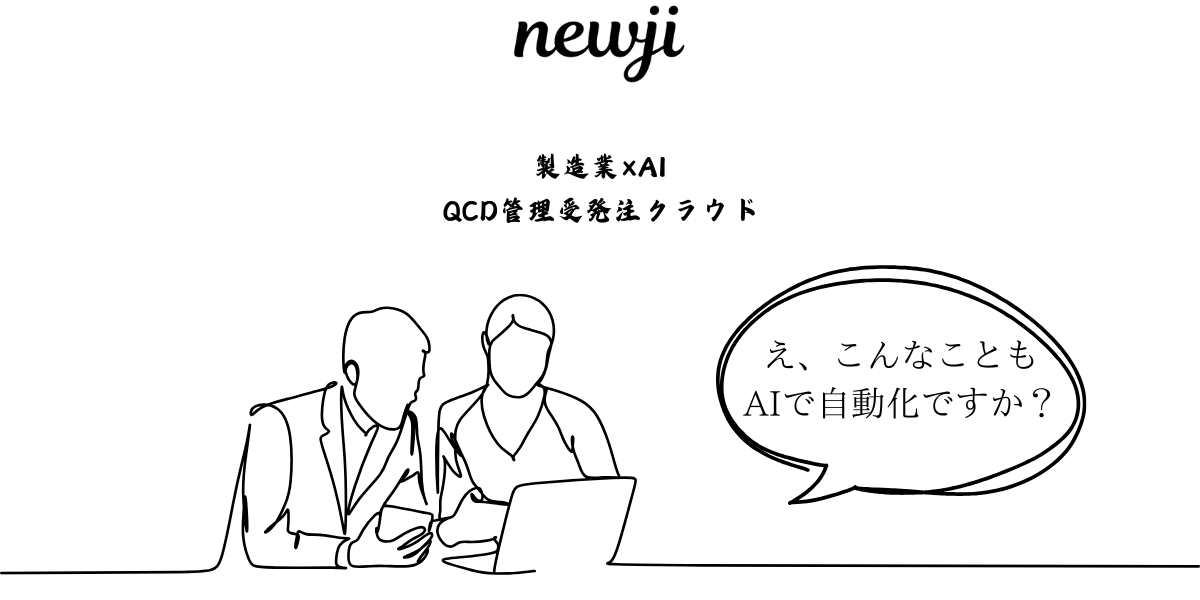
A must-see for new employees in the R&D department! Fundamentals of Bayesian statistics and methods for analyzing experimental data
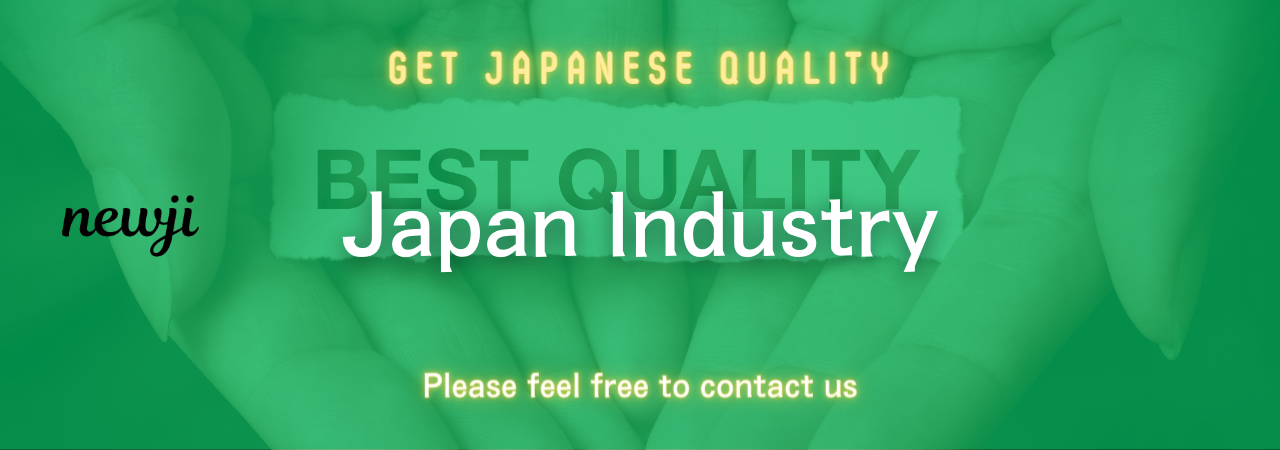
目次
Understanding Bayesian Statistics
Bayesian statistics is a powerful tool used to analyze experimental data, especially in research and development departments.
It offers a flexible approach for incorporating prior knowledge or expert opinions, alongside the evidence presented by the data itself.
To understand Bayesian statistics, it’s essential to grasp two main concepts: prior probability and posterior probability.
Prior probability represents the knowledge or beliefs we have before observing the data.
It is the starting point of Bayesian analysis and can be derived from previous studies or expert experience.
Posterior probability, on the other hand, is the updated probability after considering the new data.
This is calculated using Bayes’ Theorem, which mathematically combines the prior with the likelihood of observing the data given a particular hypothesis.
Bayes’ Theorem Explained
Bayes’ Theorem is the foundation of Bayesian statistics.
This theorem is expressed with the following formula:
\[ P(H|E) = \frac{P(E|H) \times P(H)}{P(E)} \]
In this formula, \( P(H|E) \) is the posterior probability, which is the probability of the hypothesis \( H \) given the evidence \( E \).
\( P(E|H) \) is the likelihood, representing the probability of observing the evidence given that the hypothesis is true.
\( P(H) \) is the prior probability of the hypothesis, and \( P(E) \) is the probability of observing the evidence under all possible hypotheses.
Applying Bayesian Statistics in R&D
An R&D department often deals with complex problems and experimental data.
Bayesian statistics offers several advantages for analyzing this data effectively.
First, it allows for incorporating prior knowledge into the analysis.
For example, if a new experiment is based on previous studies, their results can inform the prior probabilities, thus refining the current analysis.
Second, Bayesian methods provide a natural way to update beliefs or hypotheses as more data becomes available.
This dynamic quality is useful in iterative research processes where new data continuously informs the analysis.
Example: Evaluating a New Drug
Consider an R&D department working on a new drug.
Initially, researchers have some prior beliefs about the drug’s effectiveness based on preclinical studies.
As clinical trial data comes in, Bayesian analysis can be used to update these beliefs.
Let’s say the prior probability of the drug being effective is moderate.
After running a small initial trial, the collected data shows promising results.
By applying Bayes’ Theorem, the posterior probability of the drug’s effectiveness increases, supporting the decision to proceed with larger trials.
This iterative update mechanism allows for a more nuanced understanding that evolves with the accumulation of evidence, ensuring that the conclusions drawn remain in line with the latest data.
Methods for Analyzing Data
Various methods can be employed for Bayesian data analysis, each suited for different types of data and research questions.
Here are a few key techniques:
1. Markov Chain Monte Carlo (MCMC)
MCMC is a computational method used to approximate complex posterior distributions.
It involves generating samples from a distribution that simulates the posterior.
These samples help estimate the probability of different hypotheses, offering insights into the most plausible outcomes based on the data.
MCMC is particularly useful when dealing with high-dimensional data or models with multiple parameters, where analytical solutions are difficult to obtain.
2. Bayesian Network Analysis
Bayesian networks are graphical models that represent dependencies among variables through directed edges.
They are highly effective for analyzing multivariate relationships and causal inferences.
In an R&D context, Bayesian networks can model the interconnections between different factors, such as chemical properties and biological responses, thus assisting in identifying key influencers on the outcome.
3. Hierarchical Bayesian Models
These models are beneficial when dealing with data that naturally occurs at different levels of hierarchy, such as nested data structures.
For instance, in clinical trials, data might be structured hierarchically with patients nested within treatment groups.
Hierarchical Bayesian models allow for partial pooling of information across different levels.
This helps in borrowing strength from wider datasets while still accounting for individual variations, leading to more robust and generalized outcomes.
Conclusion: Embracing Bayesian Methods
For new employees in an R&D department, understanding the fundamentals of Bayesian statistics and methods for data analysis is invaluable.
This approach not only provides a framework for incorporating existing knowledge but also enhances the ability to draw informed conclusions from new evidence.
By using Bayesian statistics, researchers can adjust hypotheses dynamically, improving the accuracy and relevance of their findings as more data becomes available.
As you embark on your journey in R&D, keep in mind the flexibility and power Bayesian analysis brings to handling experimental data.
With practice, these techniques will become integral tools in your pursuit of innovative solutions and scientific breakthroughs.
資料ダウンロード
QCD管理受発注クラウド「newji」は、受発注部門で必要なQCD管理全てを備えた、現場特化型兼クラウド型の今世紀最高の受発注管理システムとなります。
ユーザー登録
受発注業務の効率化だけでなく、システムを導入することで、コスト削減や製品・資材のステータス可視化のほか、属人化していた受発注情報の共有化による内部不正防止や統制にも役立ちます。
NEWJI DX
製造業に特化したデジタルトランスフォーメーション(DX)の実現を目指す請負開発型のコンサルティングサービスです。AI、iPaaS、および先端の技術を駆使して、製造プロセスの効率化、業務効率化、チームワーク強化、コスト削減、品質向上を実現します。このサービスは、製造業の課題を深く理解し、それに対する最適なデジタルソリューションを提供することで、企業が持続的な成長とイノベーションを達成できるようサポートします。
製造業ニュース解説
製造業、主に購買・調達部門にお勤めの方々に向けた情報を配信しております。
新任の方やベテランの方、管理職を対象とした幅広いコンテンツをご用意しております。
お問い合わせ
コストダウンが利益に直結する術だと理解していても、なかなか前に進めることができない状況。そんな時は、newjiのコストダウン自動化機能で大きく利益貢献しよう!
(β版非公開)