- お役立ち記事
- Aggregation processing
Aggregation processing
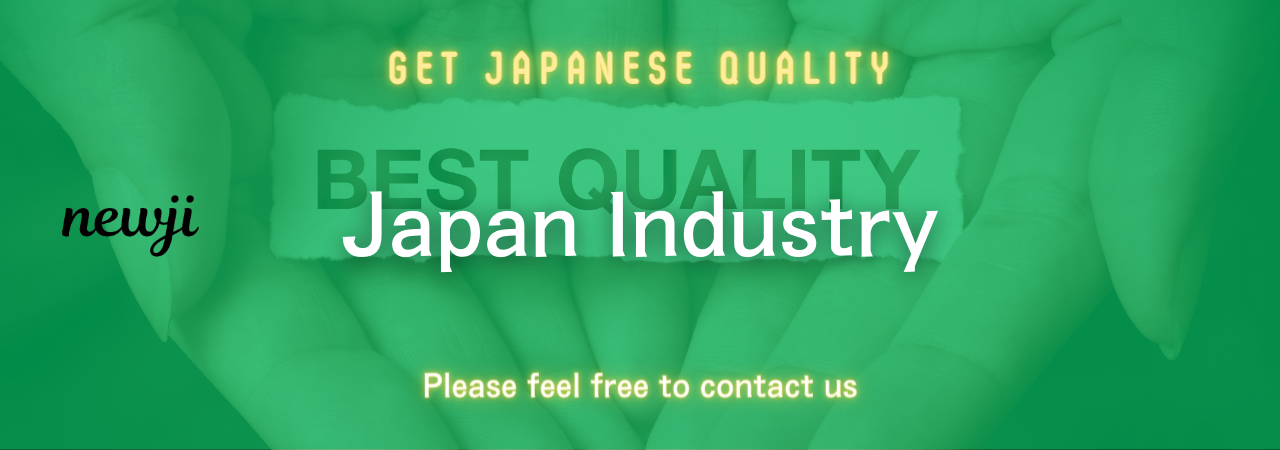
目次
Understanding Aggregation Processing
Aggregation processing is a crucial concept in the world of data management and analysis.
It involves combining multiple pieces of data to create a summary or a consolidated view.
This process is vital for making sense of vast amounts of information and turning it into actionable insights.
What is Aggregation?
Aggregation is a method of processing data by grouping and then performing some kind of operation on these groups to produce an output.
This could involve operations such as summing, averaging, counting, or finding the maximum or minimum values.
Essentially, aggregation helps simplify complex data sets, making them more understandable and easier to analyze.
For instance, if you have sales data for a whole year, aggregating this data by month can give you the total sales for each month.
This is much simpler to analyze than looking at thousands of individual sales records.
Why is Aggregation Processing Important?
Aggregation processing plays a vital role in data analysis for several reasons.
Firstly, it helps reduce data size, making it easier to manage and process.
When you aggregate data, you transform a large dataset into a smaller, more manageable summary.
Secondly, it provides essential insights that can guide decision-making.
By identifying trends and patterns, businesses can make informed decisions that drive growth and efficiency.
Finally, aggregation is foundational for reporting and visualization.
Data aggregated into summaries is often used in dashboards, charts, and graphs, providing a clearer visual picture of complex information.
Types of Aggregation Operations
There are various types of aggregation operations, each serving a specific purpose depending on the information needed.
1. **Sum**: This operation involves adding up all the values in a dataset or group.
For example, calculating the total sales revenue for the past year.
2. **Average**: Also known as the mean, this operation finds the middle value of a dataset by dividing the sum of all the values by the number of values.
This is useful for understanding the typical value in a dataset.
3. **Count**: This operation counts the number of items in a dataset or group.
It is widely used for determining the volume of entries or occurrences.
4. **Maximum and Minimum**: These operations find the highest and lowest values in a dataset, respectively.
They are useful for identifying outliers or extremes in the data.
5. **Median**: The median finds the central value of a dataset when all values are ordered.
This operation is less affected by outliers than the average.
Common Use Cases of Aggregation Processing
Aggregation processing can be applied in various industries and scenarios, each benefiting significantly from its ability to simplify and summarize data.
Business and Finance
Businesses use aggregation to gain insights into their operations.
For example, they might aggregate sales data by region to determine which areas are performing best.
Financial institutions might aggregate transaction data to identify spending habits or detect fraudulent activities.
Marketing and Sales
In marketing, aggregation helps in understanding customer behavior.
Marketers can aggregate customer interactions across various channels to tailor more effective campaigns.
Sales teams might use aggregated data to assess the performance of different products over time.
Healthcare
Healthcare providers aggregate patient data to better understand trends in health conditions across populations.
This information can be crucial for public health decisions and for allocating resources effectively.
Software Development
Developers use aggregation in software analytics to monitor and improve application performance.
By aggregating error logs, teams can identify recurring issues and address them efficiently.
Challenges in Aggregation Processing
While aggregation processing offers various benefits, it also presents certain challenges that must be addressed for optimal results.
Data Quality and Consistency
The results of aggregation are only as good as the data being aggregated.
Inconsistent or poor-quality data can lead to inaccurate conclusions.
Ensuring data accuracy and consistency is essential for reliable aggregation.
Handling Large Volumes of Data
With the explosion of big data, efficiently aggregating large datasets poses a significant challenge.
Organizations need robust tools and techniques to manage and process data at scale.
Choosing the Right Aggregation Level
Determining the appropriate level of aggregation is crucial.
Too much aggregation can obscure important details, while too little can overwhelm users with excessive information.
Conclusion
Aggregation processing is a fundamental technique in data management, providing a way to turn extensive datasets into meaningful insights.
Whether in business, healthcare, or any other field, aggregation helps simplify data analysis, guiding better decision-making.
By understanding and overcoming its challenges, organizations can harness aggregation to unlock the full potential of their data.
資料ダウンロード
QCD調達購買管理クラウド「newji」は、調達購買部門で必要なQCD管理全てを備えた、現場特化型兼クラウド型の今世紀最高の購買管理システムとなります。
ユーザー登録
調達購買業務の効率化だけでなく、システムを導入することで、コスト削減や製品・資材のステータス可視化のほか、属人化していた購買情報の共有化による内部不正防止や統制にも役立ちます。
NEWJI DX
製造業に特化したデジタルトランスフォーメーション(DX)の実現を目指す請負開発型のコンサルティングサービスです。AI、iPaaS、および先端の技術を駆使して、製造プロセスの効率化、業務効率化、チームワーク強化、コスト削減、品質向上を実現します。このサービスは、製造業の課題を深く理解し、それに対する最適なデジタルソリューションを提供することで、企業が持続的な成長とイノベーションを達成できるようサポートします。
オンライン講座
製造業、主に購買・調達部門にお勤めの方々に向けた情報を配信しております。
新任の方やベテランの方、管理職を対象とした幅広いコンテンツをご用意しております。
お問い合わせ
コストダウンが利益に直結する術だと理解していても、なかなか前に進めることができない状況。そんな時は、newjiのコストダウン自動化機能で大きく利益貢献しよう!
(Β版非公開)