- お役立ち記事
- AI for Equipment Monitoring and Fault Prediction in Maintenance
AI for Equipment Monitoring and Fault Prediction in Maintenance
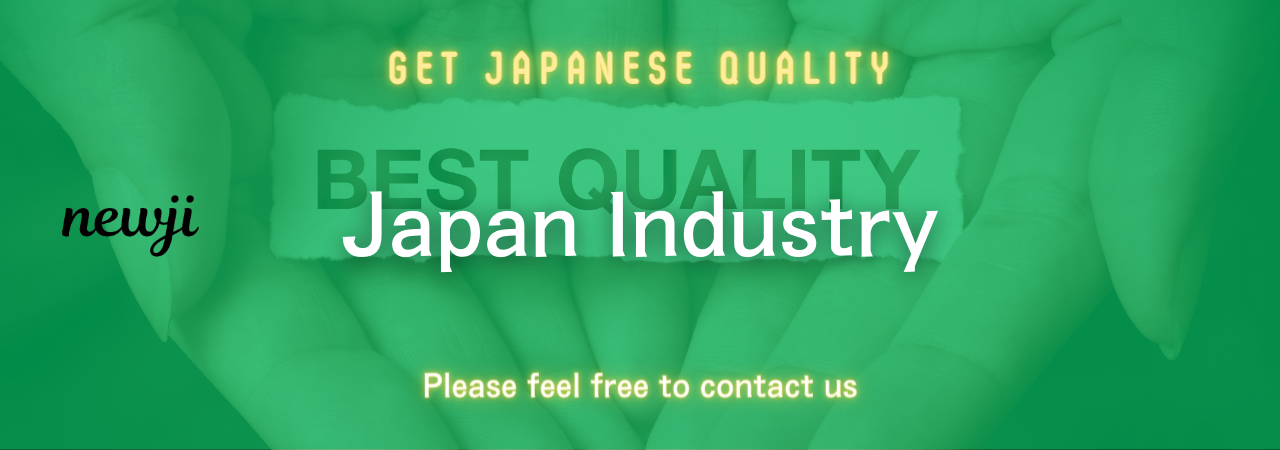
目次
The Importance of AI in Equipment Monitoring
Artificial Intelligence (AI) is revolutionizing industries around the world, and equipment monitoring in maintenance is no exception.
Monitoring the health and efficiency of machinery and equipment is vital for any industry that relies on mechanical operations.
AI offers a more advanced approach to this task, providing precise monitoring capabilities and fault prediction to avoid costly breakdowns.
AI applications in equipment monitoring offer substantial improvements over traditional methods.
With the ability to process vast amounts of data quickly, AI can predict potential faults and maintenance needs before they occur.
This approach saves time, reduces costs, and increases the operational life of machinery.
The Role of Predictive Maintenance
Predictive maintenance is a concept that has been made more feasible and effective through AI technologies.
Instead of reacting to failures, predictive maintenance allows companies to anticipate them.
AI uses data collected from sensors and historical performance information to predict equipment failures before they happen.
This method is not only cost-effective but also significantly reduces downtime.
By identifying potential issues early, companies can plan maintenance schedules during non-peak hours, thus minimizing disruptions in production or service delivery.
How AI Monitors Equipment
AI in equipment monitoring primarily relies on sensors attached to machinery.
These sensors continuously collect data on various parameters such as temperature, vibration, noise, and pressure.
This data is then analyzed by AI algorithms to detect anomalies indicating potential equipment failures.
The beauty of AI lies in its learning capability.
With each dataset, AI systems become more adept at identifying patterns linked to specific faults.
Over time, this results in increasingly accurate predictions and more effective maintenance planning.
Real-Time Data Analysis
One of the greatest advantages of using AI in equipment monitoring is real-time data analysis.
Traditional monitoring techniques might record data over time and provide reports later.
However, AI can process and analyze data as it is generated, offering instant insights into equipment conditions.
Real-time analysis helps in immediately notifying operators about any irregularities.
This instant alert means that potential issues can be addressed as soon as they are detected, preventing further damage and costlier repairs.
AI-Based Fault Prediction
Fault prediction is perhaps the most significant benefit AI brings to the table in equipment monitoring.
By predicting faults, AI helps organizations avoid unexpected breakdowns, thus protecting expensive equipment from severe damage.
The predictive models used by AI are designed based on historical data, examining all variables that could potentially lead to equipment failures.
These models consider everything from operational history to environmental conditions, offering a comprehensive view of potential risks.
Improving Efficiency and Safety
Incorporating AI into equipment monitoring significantly improves operational efficiency and safety.
By ensuring that machinery operates within safe parameters, companies can prevent accidents and create a safer working environment for their employees.
Furthermore, efficient equipment operation reduces energy consumption and wear-and-tear, thereby extending the lifespan of machinery.
This not only reduces maintenance and replacement costs but also supports environmental sustainability by lessening the need for new manufacturing.
Challenges and Solutions
Despite its advantages, implementing AI in equipment monitoring does pose some challenges.
One major challenge is the initial cost of integration, as setting up AI infrastructure requires significant investment in technology and training.
However, the long-term benefits often outweigh the initial costs.
Once implemented, AI systems can lead to significant savings over time, making them a worthy investment for future-proofing equipment management.
Data privacy and security are other concerns that organizations must address.
Sensitive data transmitted by sensors should be secured against potential breaches.
This requires robust cybersecurity measures to protect the integrity and confidentiality of the information collected and processed by AI systems.
The Future of AI in Maintenance
The role of AI in equipment monitoring and fault prediction will only expand as technology continues to advance.
Future developments are likely to bring even more sophisticated monitoring capabilities, including predictive analytics and machine learning enhancements.
The ongoing integration of the Internet of Things (IoT) will further enhance AI’s ability to monitor equipment across multiple locations.
With IoT, equipment can communicate over networks, providing even greater quantities of data for AI to analyze and predict outcomes.
In conclusion, AI represents a transformative force in equipment monitoring and maintenance.
Through efficient fault prediction and real-time data analysis, it offers a proactive approach to managing and maintaining machinery.
As industries continue to embrace this technology, they will enjoy greater operational efficiency, enhanced safety, and longer equipment life cycles.
The future of maintenance is indeed digital, and AI is at the forefront of this revolution.
資料ダウンロード
QCD調達購買管理クラウド「newji」は、調達購買部門で必要なQCD管理全てを備えた、現場特化型兼クラウド型の今世紀最高の購買管理システムとなります。
ユーザー登録
調達購買業務の効率化だけでなく、システムを導入することで、コスト削減や製品・資材のステータス可視化のほか、属人化していた購買情報の共有化による内部不正防止や統制にも役立ちます。
NEWJI DX
製造業に特化したデジタルトランスフォーメーション(DX)の実現を目指す請負開発型のコンサルティングサービスです。AI、iPaaS、および先端の技術を駆使して、製造プロセスの効率化、業務効率化、チームワーク強化、コスト削減、品質向上を実現します。このサービスは、製造業の課題を深く理解し、それに対する最適なデジタルソリューションを提供することで、企業が持続的な成長とイノベーションを達成できるようサポートします。
オンライン講座
製造業、主に購買・調達部門にお勤めの方々に向けた情報を配信しております。
新任の方やベテランの方、管理職を対象とした幅広いコンテンツをご用意しております。
お問い合わせ
コストダウンが利益に直結する術だと理解していても、なかなか前に進めることができない状況。そんな時は、newjiのコストダウン自動化機能で大きく利益貢献しよう!
(Β版非公開)