- お役立ち記事
- AI predictions and parameters
月間76,176名の
製造業ご担当者様が閲覧しています*
*2025年3月31日現在のGoogle Analyticsのデータより
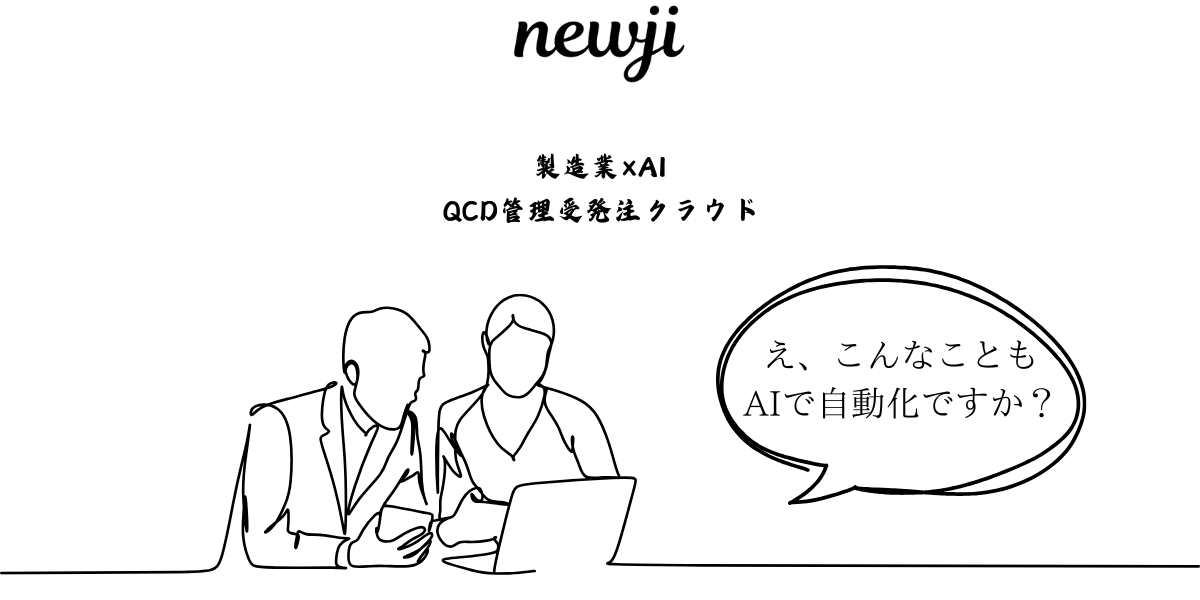
AI predictions and parameters
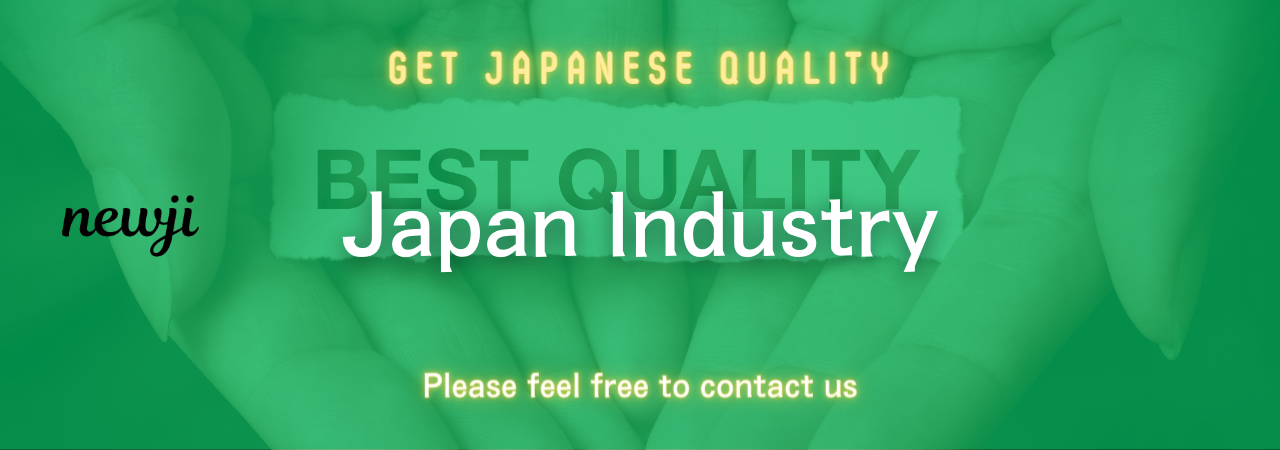
目次
Understanding AI Predictions
Artificial Intelligence (AI) has become a pivotal part of our daily lives, influencing various sectors and transforming them with its capabilities.
One of the core functions of AI is to make predictions based on the data it’s fed, which can be incredibly accurate if managed correctly.
But how does this actually work, and why is it so important?
In simple terms, AI predictions are like educated guesses made by machines.
These predictions are generated through complex algorithms which sift through massive amounts of data to identify patterns or trends.
Once these patterns are recognized, the AI can predict future outcomes or behaviors based on new data it receives.
AI predictions are crucial in areas like weather forecasting, financial market analysis, and healthcare diagnostics because they can provide insights that are often beyond human calculation abilities.
The accuracy of these predictions largely depends on the quality and quantity of the data input, as well as the parameters set during the AI’s training phase.
How AI Predictions Work
The process starts with data collection.
AI requires a significant amount of data to make reliable predictions.
This data is then divided into two sets: one for training the AI model and the other for validating its accuracy.
During the training phase, the AI algorithm processes the training data, learning to identify patterns and relationships between different data points.
This is where parameters come into play.
Parameters are the adjustable parts of the algorithm that define its architecture and capabilities.
They include things like the weights of connections in neural networks or the coefficients in linear regressions.
After training, the AI model is tested with the validation data set to see how well it predicts outcomes.
If the model performs well, it can be put into practice for real-world predictions.
If not, adjustments are made to the parameters or the data set used for training to improve accuracy.
The Importance of Parameters in AI
Parameters are critical in defining how an AI model functions.
Think of them as the settings or dials that can be adjusted to change the output.
There are two main types: hyperparameters and model parameters.
Hyperparameters are the configuration settings used to structure the model before the learning begins.
These can include settings like learning rate, batch size, and the number of layers in a neural network.
On the other hand, model parameters are values determined from the data itself during the training process.
Adjusting these parameters is essential to ensure the AI model is tuned correctly to make accurate predictions.
However, it’s a delicate balance; too many adjustments can lead to overfitting, where the model learns the training data too well but fails to generalize to new data.
Challenges with AI Predictions and Parameters
While AI predictions are powerful, they are not foolproof and come with certain challenges.
One major issue is data quality.
If the data used to train the AI is flawed or biased, the predictions will also be unreliable or skewed.
Another challenge lies in selecting the right parameters.
There are potentially hundreds of parameters to adjust, and finding the right combination is often through trial and error, which can be a time-consuming process.
Moreover, interpreting AI predictions can be difficult.
The algorithms can appear as a “black box,” where the decision-making process is not easily understandable, even to experts.
Lastly, computational resources are a significant challenge.
Training sophisticated AI models with millions of parameters requires substantial processing power and storage, which might not always be available.
The Future of AI Predictions
Despite these challenges, the future of AI predictions is very promising.
As technology advances, so does the ability of AI to learn and predict more accurately.
Researchers are continuously developing new algorithms that require less data and computational power, making AI more accessible to a broader audience.
Additionally, explainable AI (XAI) is gaining traction, aiming to make AI decision processes more transparent and understandable.
Industries are likely to see AI becoming even more ingrained in their processes, helping companies make data-driven decisions with speed and precision.
From predicting consumer behavior to detecting early signs of disease, AI predictions could revolutionize how we approach problems and find solutions.
Conclusion
AI predictions are revolutionizing the way industries operate by providing quick, data-driven insights.
The key to accurate AI predictions lies in high-quality data and well-tuned parameters.
While challenges exist, ongoing advancements in this field indicate a future where AI predictions are both more reliable and interpretable.
These predictions help us make better decisions and prepare for the future with better information than ever before.
資料ダウンロード
QCD管理受発注クラウド「newji」は、受発注部門で必要なQCD管理全てを備えた、現場特化型兼クラウド型の今世紀最高の受発注管理システムとなります。
ユーザー登録
受発注業務の効率化だけでなく、システムを導入することで、コスト削減や製品・資材のステータス可視化のほか、属人化していた受発注情報の共有化による内部不正防止や統制にも役立ちます。
NEWJI DX
製造業に特化したデジタルトランスフォーメーション(DX)の実現を目指す請負開発型のコンサルティングサービスです。AI、iPaaS、および先端の技術を駆使して、製造プロセスの効率化、業務効率化、チームワーク強化、コスト削減、品質向上を実現します。このサービスは、製造業の課題を深く理解し、それに対する最適なデジタルソリューションを提供することで、企業が持続的な成長とイノベーションを達成できるようサポートします。
製造業ニュース解説
製造業、主に購買・調達部門にお勤めの方々に向けた情報を配信しております。
新任の方やベテランの方、管理職を対象とした幅広いコンテンツをご用意しております。
お問い合わせ
コストダウンが利益に直結する術だと理解していても、なかなか前に進めることができない状況。そんな時は、newjiのコストダウン自動化機能で大きく利益貢献しよう!
(β版非公開)