- お役立ち記事
- AI technology and multimodal machine learning of “language, voice, and images” and its applications
月間76,176名の
製造業ご担当者様が閲覧しています*
*2025年3月31日現在のGoogle Analyticsのデータより
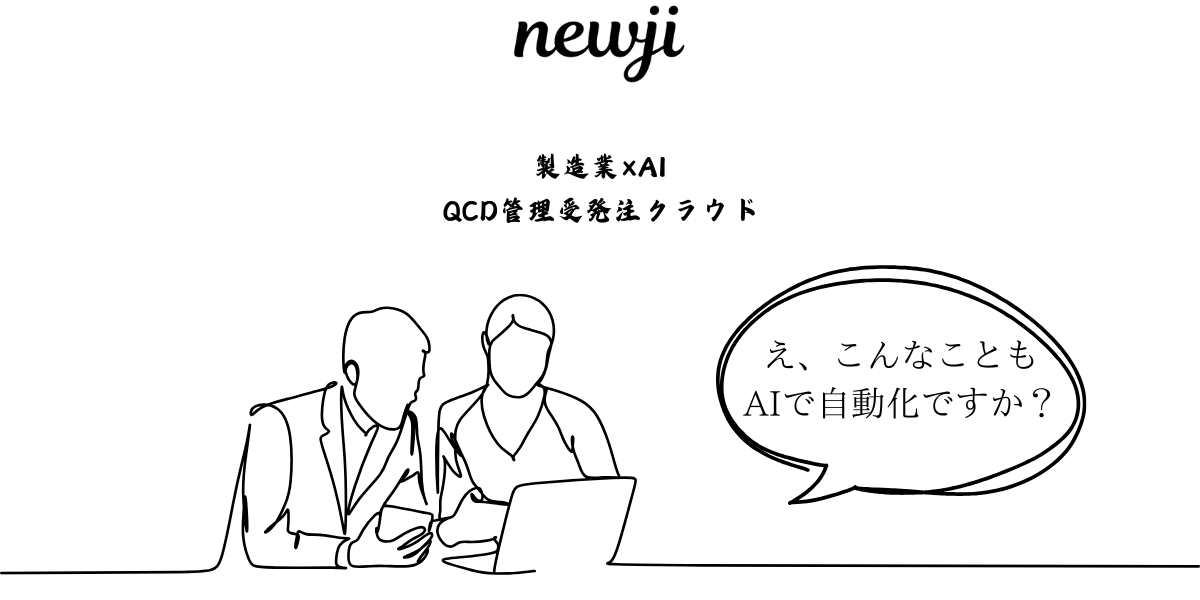
AI technology and multimodal machine learning of “language, voice, and images” and its applications
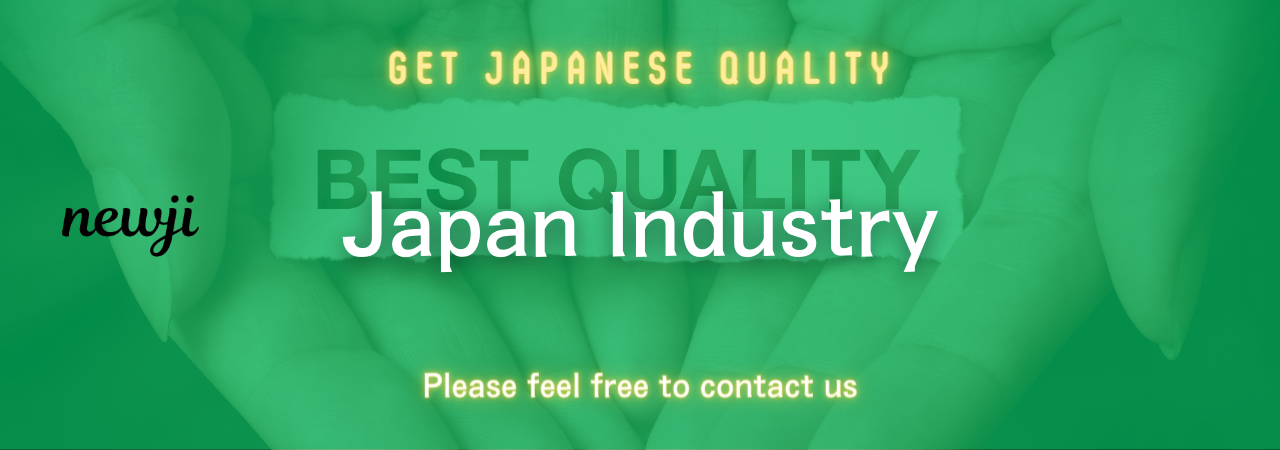
目次
Understanding AI Technology and Multimodal Machine Learning
Artificial Intelligence (AI) has grown exponentially, having a profound impact across various sectors.
One notable advancement in this field is multimodal machine learning, known for its ability to process and integrate multiple data types such as language, voice, and images.
This powerful technology allows machines to interpret information similar to how humans do, and its applications are diverse and far-reaching.
What is Multimodal Machine Learning?
Multimodal machine learning refers to models that can handle and integrate data from different modes or types.
These models are designed to process diverse inputs like text, audio, and visual data simultaneously.
The goal is to unify the interpretation of these various data forms, allowing the machine to make more informed decisions or predictions.
For instance, consider a system that can read an article (language), listen to a podcast (voice), and analyze a photograph (images).
Each of these data types provides unique information that contributes to a holistic understanding of the content at hand.
Language Processing in Multimodal AI
Language, in the context of multimodal machine learning, involves processing and understanding written and spoken content.
Natural Language Processing (NLP) is the component of AI that deals with language, enabling machines to comprehend and generate human language in a valuable way.
Technologies like GPT-3 or BERT represent milestones in this domain, allowing applications to perform tasks ranging from translation to sentiment analysis with remarkable accuracy.
By incorporating NLP into multimodal systems, enhancements occur in diverse applications, such as more nuanced chatbot interactions and improved automation in customer support.
Voice Recognition and its Role
Voice recognition is another facet of multimodal machine learning, where AI systems are taught to identify and interpret the human voice.
Voice recognition technology can understand dialects, accents, and even context, making it highly adaptive in real-world settings.
Applications in this field are vast.
From smart assistants like Alexa and Siri learning individual user preferences from voice commands to transcribing voice notes accurately, voice recognition has become a critical component of AI solutions.
By combining voice data with other modalities, more sophisticated and responsive systems are created, enhancing user experience and functionality.
Image Analysis in Multimodal Learning
Image analysis encompasses interpreting and processing visual data.
In the context of multimodal learning, images provide contextual information that text and voice cannot convey alone.
Image recognition technology has evolved significantly, allowing AI to identify objects, faces, and scenes with high precision.
Applications proliferate across various sectors, from healthcare diagnosing medical conditions through scans to security systems leveraging facial recognition for authentication.
Integrating image data with other modalities enriches the understanding of the environment, leading to more intelligent automation and predictive analytics.
Applications of Multimodal Machine Learning
The integration of language, voice, and images has led to groundbreaking applications across different industries.
Healthcare and Multimodal AI
In healthcare, multimodal machine learning assists in accurate diagnosis and patient care management.
AI systems can analyze medical records (language), listen to patient descriptions of symptoms (voice), and evaluate medical imagery (images) to provide comprehensive diagnostic insights.
Such integration leads to improved patient outcomes and streamlined clinical workflows.
Entertainment and Multimodal Experiences
Entertainment industries leverage multimodal AI to create immersive experiences.
Video games and virtual reality platforms integrate voice commands, narrative text, and dynamic graphics, providing users with a seamless interaction experience.
Personalized content recommendations also benefit from these technologies, understanding viewer preferences better by analyzing viewing patterns (images) and reviewing user feedback (language and voice).
Education and Personalized Learning
In education, multimodal machine learning plays a crucial role in developing personalized learning experiences.
Educational tools adapt to individual student needs by analyzing reading comprehension (language), listening skills (voice), and engagement through visual content (images).
This tailored approach enhances learning efficiency and accommodates diverse learning styles.
Retail and Customer Service
Retail sectors deploy multimodal AI to enhance customer service and sales processes.
By integrating chatbots with voice recognition and visual product searches, businesses provide efficient, personalized shopping experiences.
Analyzing customer interactions across these modalities enables creating highly targeted marketing campaigns and improves service delivery.
The Future of Multimodal Machine Learning
The future of multimodal machine learning holds enormous potential.
As technology advances, we can expect even more sophisticated applications that seamlessly blend different data forms to solve complex problems.
As AI systems become more adept at understanding and interpreting multiple modalities, they will exhibit improved situational awareness and deliver more accurate and relevant responses in real-time.
This will likely spur innovations not just in existing applications but also open new realms of possibilities for AI in day-to-day life.
Continuous research and development in multimodal machine learning are critical to overcoming challenges and maximizing its potential benefits.
By fostering collaboration between academia and industry, breakthroughs can accelerate, propelling AI technologies toward more meaningful and impactful use cases.
In conclusion, the integration of language, voice, and images in AI through multimodal machine learning represents a significant leap forward in how machines interact with the world.
Its applications are transforming industries and improving how we live and work, promising a future where AI not only supports but also enriches human experiences in a myriad of ways.
資料ダウンロード
QCD管理受発注クラウド「newji」は、受発注部門で必要なQCD管理全てを備えた、現場特化型兼クラウド型の今世紀最高の受発注管理システムとなります。
ユーザー登録
受発注業務の効率化だけでなく、システムを導入することで、コスト削減や製品・資材のステータス可視化のほか、属人化していた受発注情報の共有化による内部不正防止や統制にも役立ちます。
NEWJI DX
製造業に特化したデジタルトランスフォーメーション(DX)の実現を目指す請負開発型のコンサルティングサービスです。AI、iPaaS、および先端の技術を駆使して、製造プロセスの効率化、業務効率化、チームワーク強化、コスト削減、品質向上を実現します。このサービスは、製造業の課題を深く理解し、それに対する最適なデジタルソリューションを提供することで、企業が持続的な成長とイノベーションを達成できるようサポートします。
製造業ニュース解説
製造業、主に購買・調達部門にお勤めの方々に向けた情報を配信しております。
新任の方やベテランの方、管理職を対象とした幅広いコンテンツをご用意しております。
お問い合わせ
コストダウンが利益に直結する術だと理解していても、なかなか前に進めることができない状況。そんな時は、newjiのコストダウン自動化機能で大きく利益貢献しよう!
(β版非公開)