- お役立ち記事
- Anomaly detection from sound and vibration using deep learning and application technology to machines
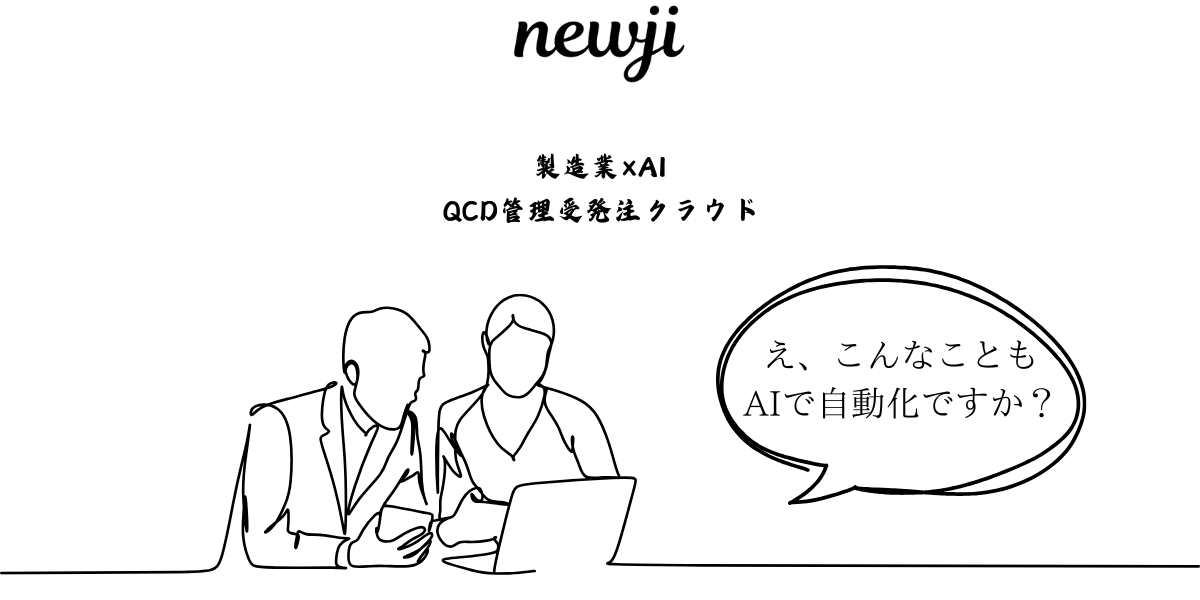
Anomaly detection from sound and vibration using deep learning and application technology to machines
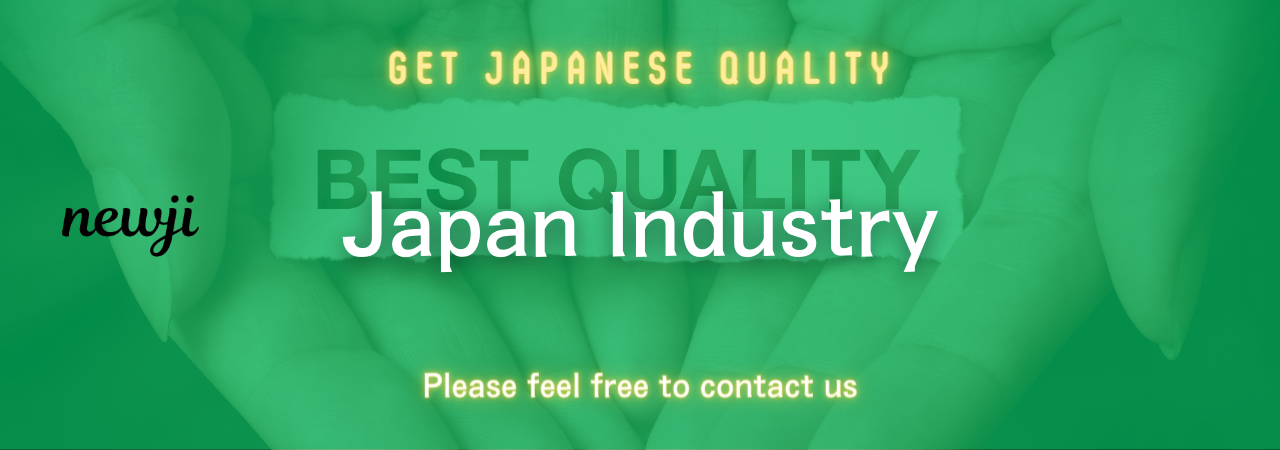
目次
Understanding Anomaly Detection
Anomaly detection refers to the process of identifying patterns in data that do not conform to expected behavior.
These anomalies can indicate crucial information, like potential faults or irregularities that could lead to bigger problems if not addressed.
In the context of machines, detecting these anomalies early can significantly reduce downtime and maintenance costs.
The Role of Sound and Vibration in Machines
Machines, by their nature, produce sound and vibration as they operate.
For instance, the hum of a motor, the clang of gears, or the subtle vibrations that a machine emits can tell much about its condition.
Analyzing sound and vibration can provide insights into the mechanical health of machines.
Variations from normal sound and vibration patterns often signal underlying issues that need attention.
Traditional Anomaly Detection Methods
In the past, traditional methods relied heavily on manual inspections and routine maintenance schedules.
Maintenance teams would listen for abnormal sounds or use handheld devices to measure vibrations.
However, these methods can be inconsistent and are often not proactive enough to detect subtle anomalies.
They require skilled technicians and can sometimes result in unnecessary downtime for machines.
Introduction to Deep Learning
Deep learning, a subset of machine learning, involves algorithms known as neural networks inspired by the human brain’s structure and function.
These networks can learn from and make decisions based on vast amounts of data.
Their ability to handle complex patterns makes deep learning particularly suitable for anomaly detection in dynamic environments such as industrial machinery.
Applying Deep Learning to Anomaly Detection
Deep learning can be used to analyze the sound and vibration data from machines.
By training deep learning models on normal operational data, these systems can learn what constitutes a ‘normal’ sound or vibration pattern.
Once trained, they can quickly detect deviations from this baseline, identifying potential anomalies.
Benefits Over Traditional Methods
Deep learning offers numerous advantages over traditional anomaly detection methods.
Firstly, it provides consistent results that do not depend on human interpretation.
Secondly, it can analyze large datasets continuously and in real-time, offering proactive maintenance solutions.
Moreover, it can detect subtle patterns that human inspectors might miss, leading to early detection of potential issues.
Implementing the Technology in Machines
To effectively implement anomaly detection using deep learning in machinery, sensors are essential.
These sensors capture real-time sound and vibration data, sending it to the deep learning models for analysis.
The placement and sensitivity of these sensors are crucial for accurate anomaly detection.
Challenges in Implementation
While the potential for deep learning in anomaly detection is high, there are challenges.
Setting up the necessary infrastructure to capture and process vast amounts of data can be costly.
Moreover, collecting and processing data in noisy environments demands precision to ensure only relevant data is analyzed.
Training the Deep Learning Models
For accurate anomaly detection, the deep learning models must be trained on comprehensive datasets.
These datasets should include a wide range of normal operating conditions and known anomalies to help the model distinguish between normal variations and actual faults.
The training process can take time and requires continual updates to account for changes in operations or new equipment.
The Future of Anomaly Detection in Industry
As deep learning technology advances, its application in anomaly detection will become even more sophisticated.
Anticipated developments include more autonomous systems, capable of not only detecting anomalies but also suggesting corrective actions.
Additionally, advancements in sensor technology will enhance data collection precision, leading to even more accurate detections.
Broader Implications and Applications
The benefits of applying deep learning to anomaly detection extend beyond just the industrial sector.
Potential applications include monitoring infrastructure health, like bridges and buildings, ensuring transport safety by analyzing vehicle performance, and even in healthcare through patient monitoring systems.
Conclusion
Anomaly detection using deep learning from sound and vibration provides significant advantages in ensuring machine reliability and efficiency.
By shifting from reactive to proactive maintenance approaches, industries can reduce costs, minimize downtime, and improve safety.
Though challenges remain, the future of this technology holds impressive potential for widespread application and further innovation.
資料ダウンロード
QCD調達購買管理クラウド「newji」は、調達購買部門で必要なQCD管理全てを備えた、現場特化型兼クラウド型の今世紀最高の購買管理システムとなります。
ユーザー登録
調達購買業務の効率化だけでなく、システムを導入することで、コスト削減や製品・資材のステータス可視化のほか、属人化していた購買情報の共有化による内部不正防止や統制にも役立ちます。
NEWJI DX
製造業に特化したデジタルトランスフォーメーション(DX)の実現を目指す請負開発型のコンサルティングサービスです。AI、iPaaS、および先端の技術を駆使して、製造プロセスの効率化、業務効率化、チームワーク強化、コスト削減、品質向上を実現します。このサービスは、製造業の課題を深く理解し、それに対する最適なデジタルソリューションを提供することで、企業が持続的な成長とイノベーションを達成できるようサポートします。
オンライン講座
製造業、主に購買・調達部門にお勤めの方々に向けた情報を配信しております。
新任の方やベテランの方、管理職を対象とした幅広いコンテンツをご用意しております。
お問い合わせ
コストダウンが利益に直結する術だと理解していても、なかなか前に進めることができない状況。そんな時は、newjiのコストダウン自動化機能で大きく利益貢献しよう!
(Β版非公開)