- お役立ち記事
- Application and practical points of AI/generation AI technology for abnormality detection and failure prediction in equipment condition monitoring/maintenance
月間76,176名の
製造業ご担当者様が閲覧しています*
*2025年3月31日現在のGoogle Analyticsのデータより
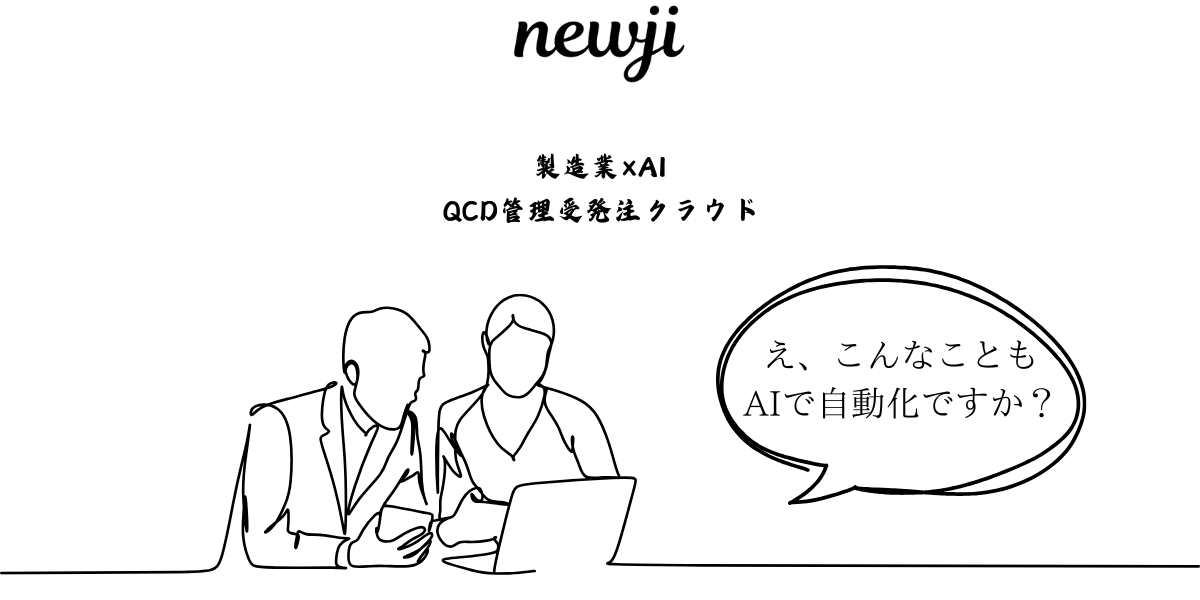
Application and practical points of AI/generation AI technology for abnormality detection and failure prediction in equipment condition monitoring/maintenance
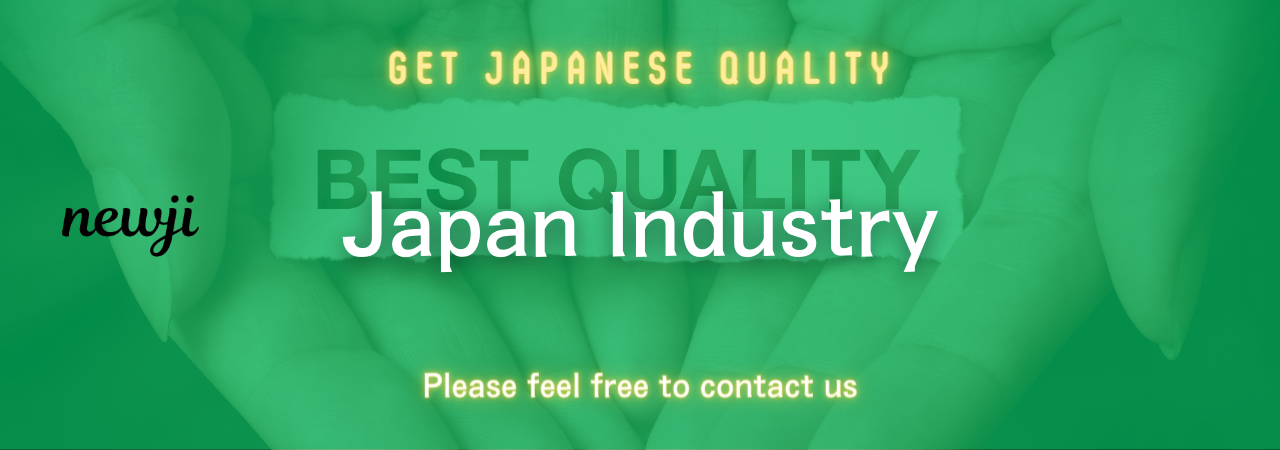
目次
Understanding AI in Equipment Monitoring
Artificial Intelligence (AI) has been revolutionizing various industries with its incredible potential to make processes more efficient and reliable.
One of the fields where AI is making a significant impact is equipment condition monitoring and maintenance.
By using advanced algorithms, AI can help detect abnormalities and predict failures before they occur, minimizing downtime and maintenance costs.
The Role of AI in Abnormality Detection
In the context of equipment condition monitoring, abnormality detection is crucial.
Machines and equipment can deviate from their normal operating patterns due to various reasons such as wear and tear, environmental conditions, or operational errors.
AI technologies, specifically machine learning algorithms, are well-suited for identifying such deviations.
Machine learning models can be trained on historical data from equipment to understand what normal operation looks like.
These models can then continuously monitor incoming data from the equipment, such as temperature, vibration, and sound, to detect any deviations from the established norm.
When the AI model identifies an anomaly, it can trigger an alert, allowing maintenance teams to investigate further.
Predictive Maintenance Using AI
Predictive maintenance is another area where AI shines.
Rather than relying on regular maintenance schedules, which can be costly and inefficient, AI allows maintenance to be performed based on the actual condition of the equipment.
This approach can lead to significant savings and extend the life of machinery.
By analyzing historical and real-time data, AI can forecast when a component is likely to fail.
For example, if a particular part is showing a gradual increase in temperature over time, the AI system can predict when it will likely overheat and fail.
This insight allows maintenance to be carried out precisely when needed, rather than on a fixed schedule.
Key AI Technologies for Monitoring and Maintenance
Several AI technologies are employed in equipment monitoring and maintenance to achieve efficient abnormality detection and failure prediction.
Machine Learning
Machine learning, a subset of AI, is a powerful tool in the realm of predictive analytics.
It involves training algorithms to learn from and make predictions based on data.
In maintenance applications, machine learning models can identify patterns that indicate potential equipment failures or abnormalities.
There are various machine learning techniques employed, including supervised, unsupervised, and reinforcement learning, each useful in its own way for different scenarios in equipment monitoring.
Deep Learning
Deep learning, an advanced form of machine learning, uses artificial neural networks to analyze intricate data patterns.
This technology is particularly effective in processing data from sensors that generate complex data streams, such as images or audio signals.
In equipment monitoring, deep learning can enhance the accuracy of anomaly detection and failure prediction by handling large and unstructured datasets, subsequently offering richer insights into equipment health.
Natural Language Processing (NLP)
Natural Language Processing might seem out of place in equipment maintenance, but it plays a crucial role in managing unstructured data like maintenance logs and operator notes.
NLP can analyze textual data to extract information about past failures, repairs, and operational conditions, which can be invaluable in training machine learning models for prediction.
Application and Practical Implementation
The practical application of AI in equipment monitoring requires integrating these technologies into existing systems and workflows effectively.
Data Collection and Integration
The first step in deploying AI for condition monitoring is gathering the right kind of data.
Sensors play a crucial role here, providing real-time data such as temperature, pressure, and vibration, which are essential for AI analytics.
This raw data needs to be integrated into a centralized system for processing.
Data integration ensures that the AI models have access to all relevant information, allowing for more accurate predictions.
Model Development and Training
Developing the right AI model involves selecting the appropriate algorithms and training them with historical and real-time data.
It is important to continuously refine these models to keep them aligned with changing equipment conditions and environmental factors.
Model validation is also critical, where the AI’s predictions are compared against known outcomes to ensure accuracy and reliability in real-world applications.
Implementation and Monitoring
Once trained, AI models are integrated into the monitoring systems where they operate in real-time.
The AI system should be capable of alerting maintenance personnel when an anomaly is detected or when a failure is predicted.
Continuous monitoring of the AI system’s performance is necessary to ensure it remains effective and accurate over time.
Benefits and Challenges
The application of AI in equipment monitoring offers numerous benefits but also presents certain challenges.
Benefits
– **Reduced Downtime:** By predicting failures before they occur, AI helps minimize unexpected equipment downtime.
– **Cost Efficiency:** Predictive maintenance significantly lowers maintenance costs by reducing unnecessary routine maintenance.
– **Extended Equipment Life:** Timely interventions based on accurate predictions can prolong an equipment’s operational life.
Challenges
– **Data Quality:** High-quality data is essential for training accurate AI models. Inadequate or noisy data can lead to less effective predictions.
– **Integration Complexity:** Incorporating AI systems into existing infrastructure can be complex and may require significant changes.
– **Technological Limitations:** AI systems need to be continuously updated to handle new kinds of data and adapt to evolving equipment conditions.
In conclusion, AI technologies bring immense value to equipment condition monitoring and maintenance, offering capabilities for abnormality detection and predictive maintenance that can lead to more efficient and cost-effective operations.
However, successful implementation requires careful planning, robust data management, and ongoing system evaluation to overcome challenges and fully leverage AI’s potential.
資料ダウンロード
QCD管理受発注クラウド「newji」は、受発注部門で必要なQCD管理全てを備えた、現場特化型兼クラウド型の今世紀最高の受発注管理システムとなります。
ユーザー登録
受発注業務の効率化だけでなく、システムを導入することで、コスト削減や製品・資材のステータス可視化のほか、属人化していた受発注情報の共有化による内部不正防止や統制にも役立ちます。
NEWJI DX
製造業に特化したデジタルトランスフォーメーション(DX)の実現を目指す請負開発型のコンサルティングサービスです。AI、iPaaS、および先端の技術を駆使して、製造プロセスの効率化、業務効率化、チームワーク強化、コスト削減、品質向上を実現します。このサービスは、製造業の課題を深く理解し、それに対する最適なデジタルソリューションを提供することで、企業が持続的な成長とイノベーションを達成できるようサポートします。
製造業ニュース解説
製造業、主に購買・調達部門にお勤めの方々に向けた情報を配信しております。
新任の方やベテランの方、管理職を対象とした幅広いコンテンツをご用意しております。
お問い合わせ
コストダウンが利益に直結する術だと理解していても、なかなか前に進めることができない状況。そんな時は、newjiのコストダウン自動化機能で大きく利益貢献しよう!
(β版非公開)