- お役立ち記事
- Application examples of evolutionary calculations that achieve both quality control and productivity improvement
Application examples of evolutionary calculations that achieve both quality control and productivity improvement
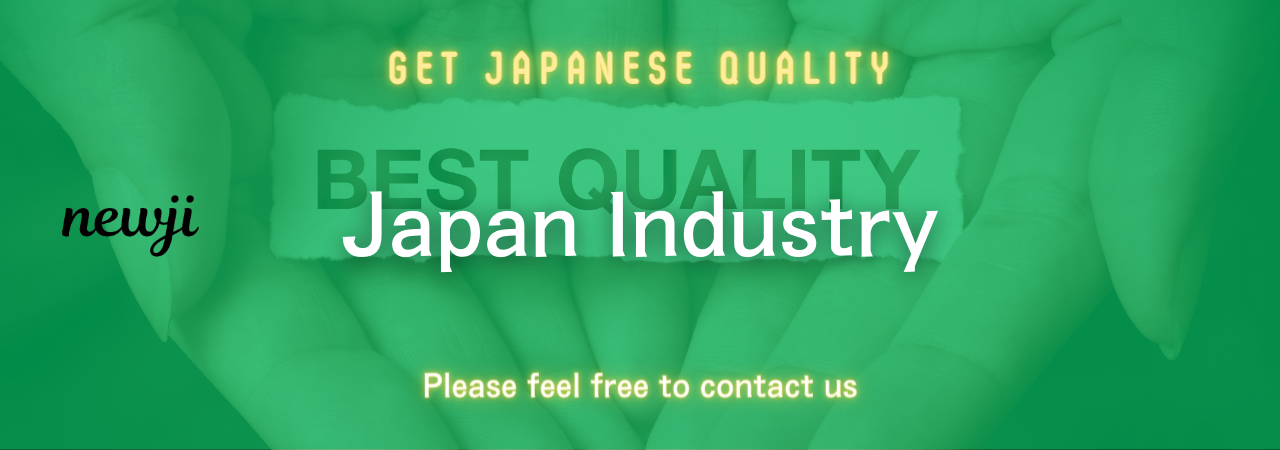
目次
Introduction to Evolutionary Calculations
Evolutionary calculations are an innovative approach in computational science that mimic the process of natural evolution to solve complex problems.
These calculations involve mechanisms such as selection, mutation, and recombination to evolve solutions over generations, ultimately finding optimal or near-optimal solutions.
In the context of modern industries, evolutionary calculations hold the potential to transform quality control and productivity processes significantly.
The Core Principles of Evolutionary Calculations
Evolutionary algorithms are based on Charles Darwin’s theory of natural selection.
They use populations of candidate solutions and apply selection pressure to improve the solutions iteratively.
Selection
Selection is the process where the best-performing solutions from a generation are chosen to create offspring for the next generation.
This ensures that only the most promising solutions continue evolving.
Mutation
Mutation introduces small random changes in the solutions.
It ensures diversity in the population and helps in exploring a broader solution space, which is essential for escaping local optima.
Recombination
Recombination, or crossover, combines different solutions to create new solutions.
It allows the sharing of good features among solutions, potentially leading to better offspring.
Applications in Quality Control
In the realm of quality control, evolutionary calculations can be leveraged to enhance product standards and consistency.
Automating Inspection Processes
Traditional inspection processes can be labor-intensive and subjective.
By using evolutionary algorithms, companies can automate these inspections, reducing human error.
For instance, machine learning models can be evolved using these algorithms to accurately identify defects in manufacturing lines.
Optimizing Test Parameters
Determining the optimal test parameters can be challenging.
Evolutionary calculations can help by evolving different test strategies and identifying the most effective one.
This leads to better utilization of resources and ensures that products meet the required quality specifications.
Enhancing Productivity with Evolutionary Calculations
Applying evolutionary calculations also facilitates notable improvements in productivity.
Process Optimization
Industries can use evolutionary algorithms to optimize their manufacturing processes.
These algorithms can simulate various scenarios and evaluate different configurations to recommend the most efficient process setups.
The results include reduced waste, lower costs, and increased throughput.
Supply Chain Management
Supply chain issues can significantly disrupt productivity.
By employing evolutionary calculations, businesses can manage their supply chains more effectively.
The algorithms can assess various logistics strategies, optimizing routes and schedules for maximum efficiency.
Case Studies Demonstrating Successful Implementations
Several companies have successfully incorporated evolutionary calculations into their workflows.
Automotive Industry
In the automotive industry, evolutionary algorithms have been used to optimize the design of engine components.
These algorithms assess different design variables, reducing development time and costs while ensuring high performance.
Pharmaceutical Industry
Pharmaceutical companies have used evolutionary calculations to speed up the drug discovery process.
By analyzing massive datasets of chemical compounds, these algorithms help identify promising candidates for new drugs, drastically reducing the time needed for research and trials.
Challenges and Considerations
Despite their advantages, implementing evolutionary calculations is not without challenges.
Computational Resources
The computational power required to run large-scale evolutionary calculations can be substantial.
Businesses need to invest in high-performance computing resources, which can be a barrier for smaller enterprises.
Algorithm Complexity
Designing and tuning evolutionary algorithms require expertise.
The complexity of these algorithms means that companies might need to hire specialists or provide extensive training for existing personnel.
Conclusion
The application of evolutionary calculations in achieving both quality control and productivity improvement holds incredible potential for modern industries.
By simulating the process of natural evolution, businesses can find more efficient solutions and adapt to changing market demands swiftly.
While the implementation comes with its set of challenges, the benefits, in the long run, outweigh the initial hurdles.
As technology continues to advance, embracing evolutionary algorithms could be key to maintaining a competitive edge in various sectors.
資料ダウンロード
QCD調達購買管理クラウド「newji」は、調達購買部門で必要なQCD管理全てを備えた、現場特化型兼クラウド型の今世紀最高の購買管理システムとなります。
ユーザー登録
調達購買業務の効率化だけでなく、システムを導入することで、コスト削減や製品・資材のステータス可視化のほか、属人化していた購買情報の共有化による内部不正防止や統制にも役立ちます。
NEWJI DX
製造業に特化したデジタルトランスフォーメーション(DX)の実現を目指す請負開発型のコンサルティングサービスです。AI、iPaaS、および先端の技術を駆使して、製造プロセスの効率化、業務効率化、チームワーク強化、コスト削減、品質向上を実現します。このサービスは、製造業の課題を深く理解し、それに対する最適なデジタルソリューションを提供することで、企業が持続的な成長とイノベーションを達成できるようサポートします。
オンライン講座
製造業、主に購買・調達部門にお勤めの方々に向けた情報を配信しております。
新任の方やベテランの方、管理職を対象とした幅広いコンテンツをご用意しております。
お問い合わせ
コストダウンが利益に直結する術だと理解していても、なかなか前に進めることができない状況。そんな時は、newjiのコストダウン自動化機能で大きく利益貢献しよう!
(Β版非公開)