- お役立ち記事
- Application of deep learning (machine learning) to CAE/engineering design and its key points
月間76,176名の
製造業ご担当者様が閲覧しています*
*2025年3月31日現在のGoogle Analyticsのデータより
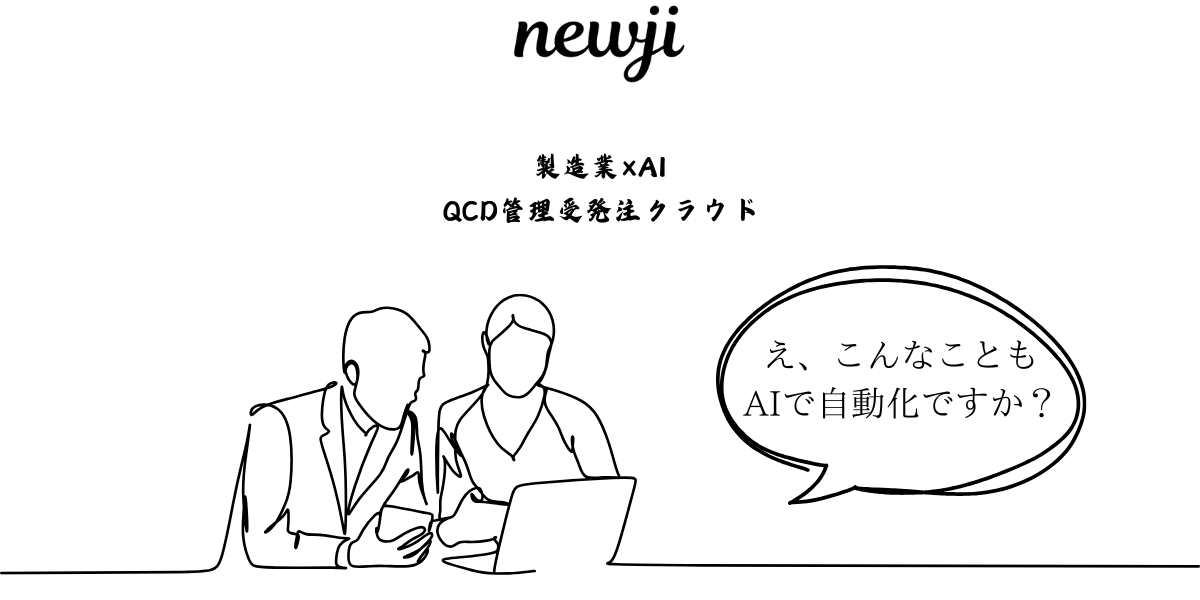
Application of deep learning (machine learning) to CAE/engineering design and its key points
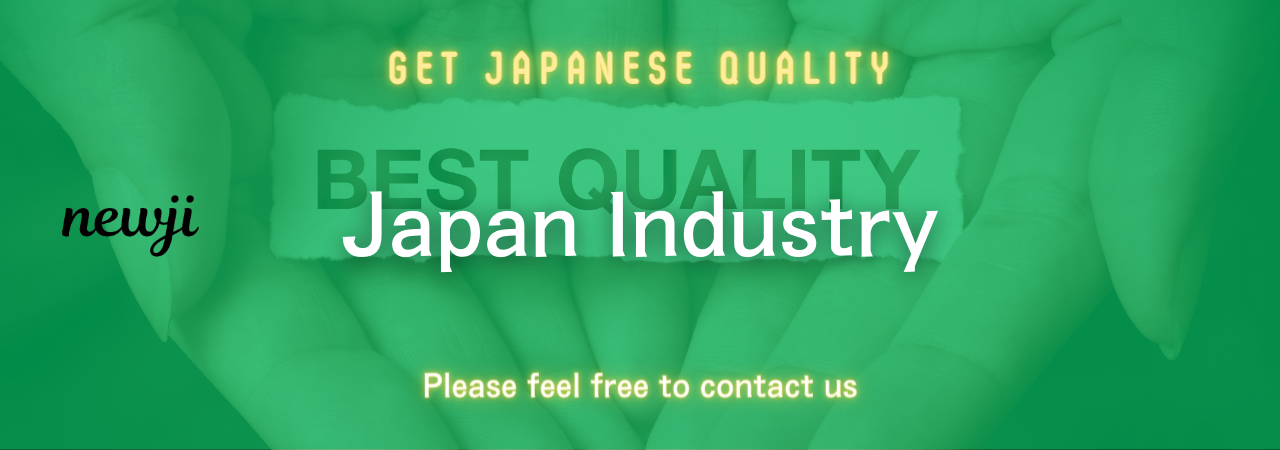
目次
Introduction to Deep Learning and CAE
Deep learning, a subset of machine learning, has increasingly become a cornerstone in advancements within many industries, including Computer-Aided Engineering (CAE) and engineering design.
Its ability to model and predict complex phenomena with remarkable accuracy has opened new avenues for innovation and optimization.
Understanding the application of deep learning to CAE/engineering design can enhance predictive capabilities, reduce computation times, and improve design accuracy.
Let’s explore why this integration is so revolutionary.
The Role of Deep Learning in CAE
CAE involves using software to simulate performance to improve product design.
Traditionally, this process has been computationally intensive, requiring extensive resources and significant time investments.
By integrating deep learning techniques, these simulations can be expedited.
Deep learning algorithms can efficiently process large datasets and identify patterns that would otherwise be incredibly time-consuming for traditional methods to discern.
For instance, in structural analysis, deep learning can predict stress distribution and deformation under various loads, enabling engineers to foresee potential failures or optimizations.
This predictive insight aids in designing more resilient and efficient structures, enhancing safety and performance while cutting down the time and cost involved.
Benefits of Integrating Deep Learning in Engineering Design
Enhancing Predictive Accuracy
Deep learning models are adept at managing and learning from vast amounts of complex data, leading to more accurate simulations and predictions.
This can significantly enhance the reliability of engineering designs.
When equipped with accurate predictions concerning how a product will respond under various conditions, engineers can make informed decisions that can lead to safer and more efficient products.
Reducing Design Cycle Times
The ability of deep learning to expedite simulation processes means that designers can iterate more rapidly.
This is particularly crucial in industries where time-to-market is a critical competitive factor.
By reducing design cycle times, companies can innovate faster and stay ahead in their respective markets.
Optimizing Resource Utilization
Deep learning can also aid in optimizing resource use by accurately predicting which design approaches will meet specifications while minimizing waste.
This is particularly valuable in industries that are resource-intensive, as it can lead to significant cost savings.
Enabling Innovative Designs
The integration of deep learning allows engineers to explore complex, non-linear design spaces that were previously too challenging to navigate purely with traditional methods.
This opens the door to innovative design possibilities that can transform industries.
Key Point Considerations in Applying Deep Learning
Data Quality and Quantity
Deep learning thrives on data.
However, the quality and quantity of data are paramount.
Ensuring data is accurate, comprehensive, and relevant is critical to training effective deep learning models.
A poor dataset can lead engineers to incorrect conclusions, which might counteract the benefits of integrating deep learning.
Computational Resources
While deep learning can reduce computation times, it initially demands significant computational resources, especially during the training phase.
Organizations must assess and allocate the necessary resources to support these processes effectively.
Investments in infrastructure or cloud-computing solutions might be necessary to leverage deep learning efficiently.
Interdisciplinary Expertise
The integration of deep learning into CAE and engineering design requires a synergy of expertise across disciplines.
This includes machine learning specialists, domain experts in engineering, and software developers.
Bringing together these diverse skills ensures that deep learning models are not only technically sound but also practically applicable.
Challenges in Implementing Deep Learning
Despite the numerous advantages, there are challenges associated with integrating deep learning into CAE and engineering design.
The complexity of developing robust deep learning models is significant.
These models not only require careful consideration of the architecture but also hyperparameters need to be tuned to each specific engineering problem.
The dynamic nature of engineering problems often means continuous retraining and modification are necessary to maintain model accuracy.
Moreover, the interpretability of deep learning models remains a concern.
While these models can be highly predictive, understanding exactly how they arrive at a conclusion can be difficult, which could pose issues in industries where explanations are necessary for compliance or client assurance.
The Future of Deep Learning in CAE and Engineering Design
The future is promising for the application of deep learning in CAE and engineering design.
With advancements in algorithm development, computational power, and data availability, the role of deep learning will likely expand even further.
Deep learning could enable real-time simulations, offering instant feedback during the design process, thereby pushing the boundaries of rapid prototyping and iterative testing.
Moreover, as the capability of models to predict and generalize enhances, the adoption in smaller firms that previously couldn’t justify the investment could also increase.
Conclusion
The application of deep learning to CAE and engineering design represents a transformative shift.
It streamlines workflows, empowers more accurate and innovative designs, and provides a competitive edge through quicker market readiness.
While challenges exist, the future of engineering design fortified by deep learning is highly optimistic.
As the technology continues to mature, its integration will become more seamless and integral to the core practices of engineering design, leading to an era where design strategies are more predictive, efficient, and innovative than ever before.
資料ダウンロード
QCD管理受発注クラウド「newji」は、受発注部門で必要なQCD管理全てを備えた、現場特化型兼クラウド型の今世紀最高の受発注管理システムとなります。
ユーザー登録
受発注業務の効率化だけでなく、システムを導入することで、コスト削減や製品・資材のステータス可視化のほか、属人化していた受発注情報の共有化による内部不正防止や統制にも役立ちます。
NEWJI DX
製造業に特化したデジタルトランスフォーメーション(DX)の実現を目指す請負開発型のコンサルティングサービスです。AI、iPaaS、および先端の技術を駆使して、製造プロセスの効率化、業務効率化、チームワーク強化、コスト削減、品質向上を実現します。このサービスは、製造業の課題を深く理解し、それに対する最適なデジタルソリューションを提供することで、企業が持続的な成長とイノベーションを達成できるようサポートします。
製造業ニュース解説
製造業、主に購買・調達部門にお勤めの方々に向けた情報を配信しております。
新任の方やベテランの方、管理職を対象とした幅広いコンテンツをご用意しております。
お問い合わせ
コストダウンが利益に直結する術だと理解していても、なかなか前に進めることができない状況。そんな時は、newjiのコストダウン自動化機能で大きく利益貢献しよう!
(β版非公開)