- お役立ち記事
- Application of image recognition technology and deep learning for visual inspection
Application of image recognition technology and deep learning for visual inspection
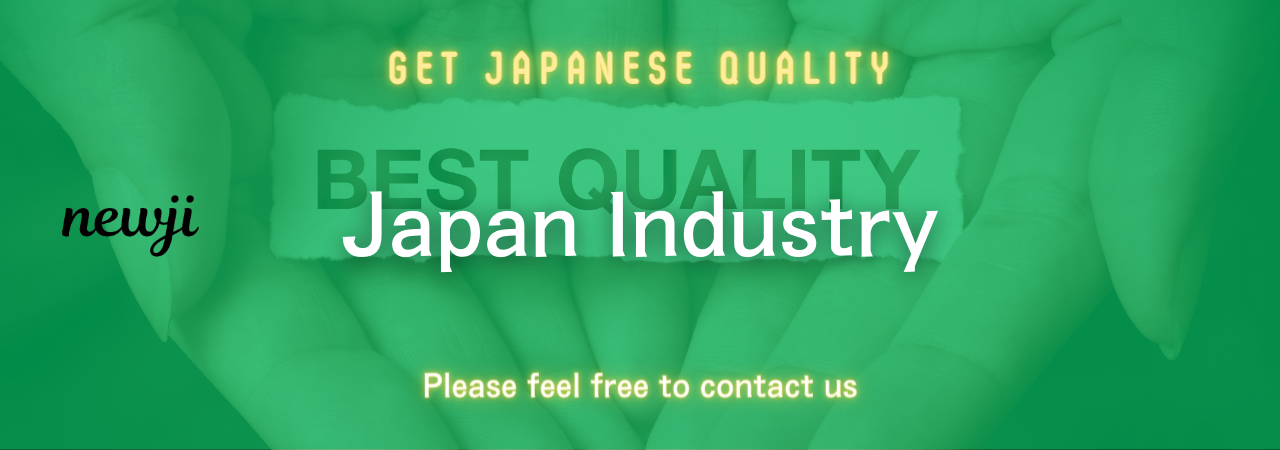
目次
Introduction to Image Recognition Technology
Image recognition technology is revolutionizing various industries by enabling machines to interpret and understand visual information from the world.
This advanced technology allows computers to recognize and process visual data, which can be used for a wide range of applications, from identifying objects and detecting anomalies to automating visual inspection processes.
At the heart of image recognition technology lies the concept of computer vision, a field of artificial intelligence that focuses on enabling machines to interpret and make sense of visual data.
Computer vision employs a combination of image processing, pattern recognition, and machine learning techniques to analyze and understand images and videos.
Deep Learning’s Role in Image Recognition
One of the primary technological advancements driving the progress of image recognition technology is deep learning.
Deep learning, a subset of machine learning, utilizes neural networks with multiple layers (hence the term “deep”) to model and solve complex problems.
These neural networks are designed to imitate the way the human brain processes information.
Convolutional Neural Networks (CNNs) are particularly significant in the field of image recognition.
CNNs are specifically designed to process and analyze structured grid data such as images.
They have multiple layers, each capable of extracting different levels of features, from basic edges and shapes to more complex patterns and objects.
Applications of Deep Learning in Visual Inspection
Deep learning has found extensive applications in visual inspection processes across various industries.
Visual inspection involves assessing products, parts, or components for defects, quality control, and compliance with standards.
By using deep learning algorithms, computers can learn to detect anomalies and defects in images with amazing accuracy, reducing the need for human intervention.
In manufacturing, for instance, deep learning-based visual inspection systems are used to examine products on production lines to ensure they meet quality standards.
These systems can swiftly identify defects such as scratches, dents, and misalignments.
In the automotive industry, image recognition systems can inspect car components, ensuring safety and quality.
Similarly, in the healthcare industry, deep learning models can analyze medical images, such as X-rays and MRIs, to detect abnormalities and diagnose diseases.
This not only speeds up the diagnostic process but also enhances accuracy, assisting medical professionals in making informed decisions.
Challenges and Considerations
While the benefits of image recognition technology and deep learning for visual inspection are substantial, there are challenges that must be addressed.
One of these challenges is the need for large amounts of data to train deep learning models effectively.
High-quality and well-labeled datasets are crucial for developing accurate models.
Another challenge is the computational power required to train these deep networks.
Deep learning models can be highly resource-intensive, requiring sophisticated hardware and significant computational efforts.
However, advancements in cloud computing and specialized hardware like GPUs are helping to mitigate these challenges.
Additionally, there is a need for constant updates and maintenance of the models to ensure their performance and adaptability to new conditions or environments.
The Future of Image Recognition and Visual Inspection
Looking ahead, the integration of image recognition technology and deep learning in visual inspection is expected to continue growing, driven by innovations and technological advancements.
As these technologies evolve, they have the potential to transform industries by enhancing efficiency, accuracy, and productivity.
The development of more sophisticated algorithms and tools will likely lead to even more precise and adaptable visual inspection systems.
These systems could potentially function in dynamic and complex industrial environments, learning and adapting in real-time.
Furthermore, the growing field of edge computing presents opportunities for decentralized processing of data, reducing latency, and enabling image recognition systems to operate more effectively in real-time applications.
Conclusion
The application of image recognition technology and deep learning for visual inspection offers substantial potential benefits across diverse sectors.
By harnessing these technologies, industries can enhance their operational efficiencies, ensure higher-quality standards, and reduce costs associated with manual inspections.
Though challenges remain, ongoing advances in computing power and algorithmic development continue to drive the field forward.
As a result, businesses and organizations can look forward to a future where visual inspection processes are more automated, accurate, and efficient, paving the way for further innovation and growth in the industry.
資料ダウンロード
QCD調達購買管理クラウド「newji」は、調達購買部門で必要なQCD管理全てを備えた、現場特化型兼クラウド型の今世紀最高の購買管理システムとなります。
ユーザー登録
調達購買業務の効率化だけでなく、システムを導入することで、コスト削減や製品・資材のステータス可視化のほか、属人化していた購買情報の共有化による内部不正防止や統制にも役立ちます。
NEWJI DX
製造業に特化したデジタルトランスフォーメーション(DX)の実現を目指す請負開発型のコンサルティングサービスです。AI、iPaaS、および先端の技術を駆使して、製造プロセスの効率化、業務効率化、チームワーク強化、コスト削減、品質向上を実現します。このサービスは、製造業の課題を深く理解し、それに対する最適なデジタルソリューションを提供することで、企業が持続的な成長とイノベーションを達成できるようサポートします。
オンライン講座
製造業、主に購買・調達部門にお勤めの方々に向けた情報を配信しております。
新任の方やベテランの方、管理職を対象とした幅広いコンテンツをご用意しております。
お問い合わせ
コストダウンが利益に直結する術だと理解していても、なかなか前に進めることができない状況。そんな時は、newjiのコストダウン自動化機能で大きく利益貢献しよう!
(Β版非公開)