- お役立ち記事
- Application of self-position estimation technology in autonomous driving and surrounding environment recognition technology using digital maps
月間76,176名の
製造業ご担当者様が閲覧しています*
*2025年3月31日現在のGoogle Analyticsのデータより
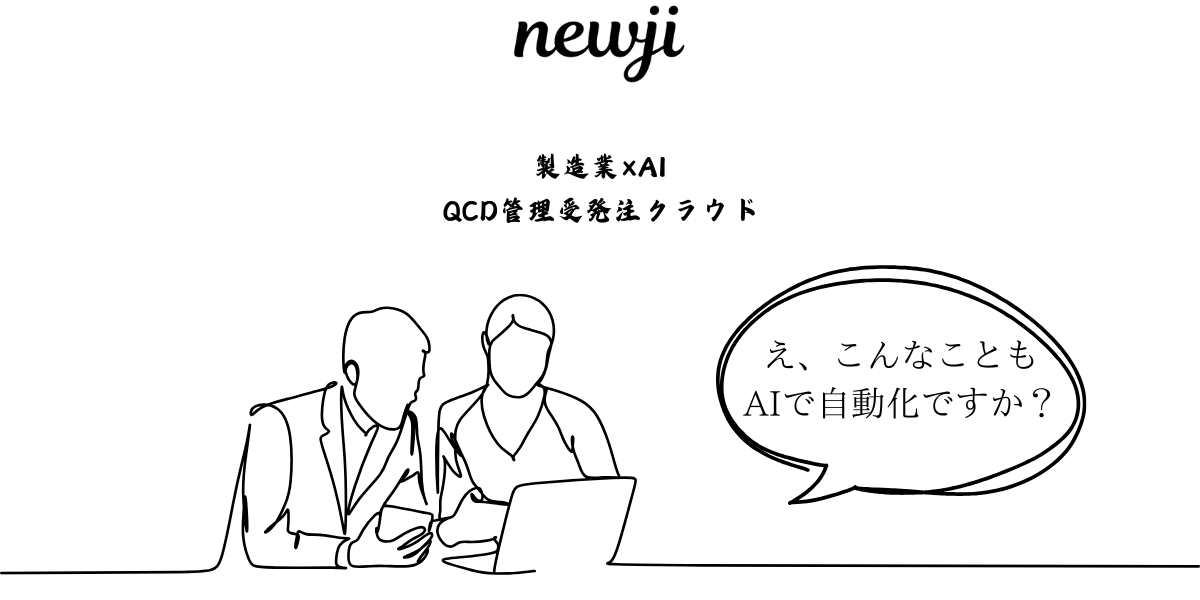
Application of self-position estimation technology in autonomous driving and surrounding environment recognition technology using digital maps
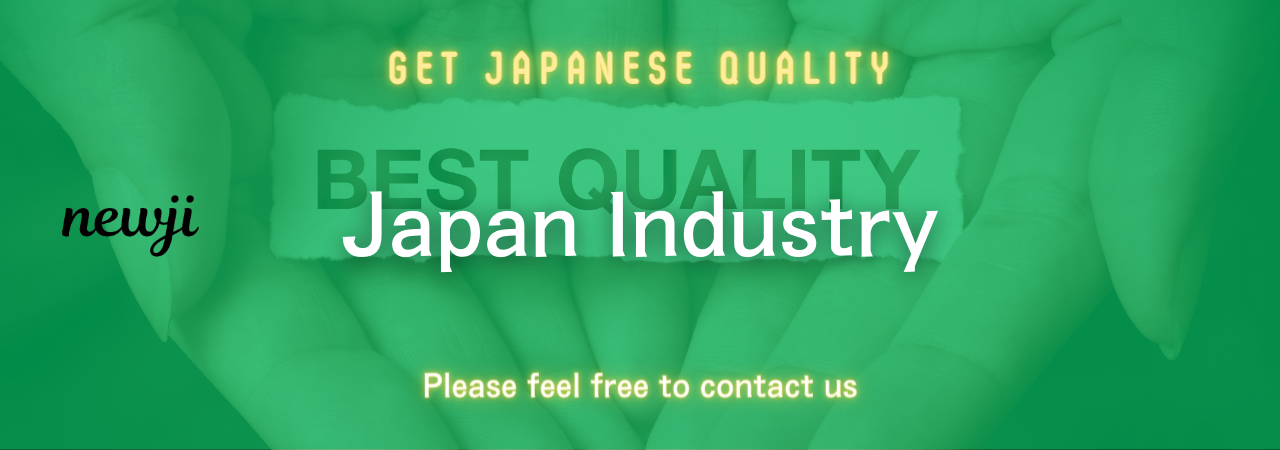
目次
Introduction to Self-Position Estimation Technology
Autonomous driving technology has seen exponential growth in recent years, aiming to revolutionize how we commute.
A critical component of this technology is self-position estimation, which allows vehicles to determine their precise location on the road.
This capability is crucial for enabling vehicles to navigate accurately, avoid obstacles, and follow traffic regulations.
Self-position estimation technology uses a combination of sensors, cameras, and GPS to provide real-time data about the vehicle’s position.
These components work together to create a comprehensive picture of the vehicle’s surroundings and its location within that environment.
By continuously updating this information, autonomous vehicles can make precise adjustments to their trajectory, ensuring a safe and efficient journey.
The Role of Digital Maps in Self-Position Estimation
Digital maps play a pivotal role in the self-position estimation process.
They provide a detailed layout of the roads, landmarks, and other crucial geographic data that autonomous vehicles use to navigate.
By integrating digital maps with onboard sensors and GPS data, vehicles can correlate their physical position with the mapped environment.
Moreover, digital maps offer additional contextual information, such as traffic signals, road signs, and lane markings.
This information allows autonomous systems to make informed decisions, such as when to change lanes or adjust speed.
The integration of digital maps ensures that the vehicle’s path aligns accurately with the intended route, enhancing both safety and reliability.
Understanding Surrounding Environment Recognition Technology
Surrounding environment recognition technology is a vital aspect of autonomous driving.
It involves the use of various sensors and cameras to detect and interpret the vehicle’s immediate environment.
This technology helps autonomous vehicles identify obstacles, pedestrians, other vehicles, and road conditions.
The primary sensors used in this process include LiDAR (Light Detection and Ranging), radar, and cameras.
LiDAR uses laser beams to create a 3D map of the surroundings, while radar systems detect objects using radio waves.
Cameras provide visual information necessary for identifying road signs, signals, and other vehicles.
Integration of Recognition Technology with Self-Position Estimation
Integrating surrounding environment recognition with self-position estimation is essential for autonomous driving systems.
Together, these technologies enable vehicles to perceive their environment accurately and respond appropriately to changes.
The combination of detailed digital maps and real-time sensor data allows vehicles to compare expected environmental features with observed ones.
When discrepancies arise, autonomous systems can adapt their navigation strategies dynamically.
For example, if a road is unexpectedly blocked, the vehicle can re-route itself efficiently using updated map data.
This fusion of technologies enhances both the precision and safety of autonomous vehicles, ensuring that they can operate smoothly even in complex urban environments.
Challenges in Self-Position Estimation and Environment Recognition
Despite the advancements, there are challenges to implementing effective self-position estimation and surrounding environment recognition.
Urban areas with tall buildings, tunnels, or dense networks of roads can cause GPS signal interference, affecting location accuracy.
In such scenarios, reliance on map data and onboard sensors becomes paramount.
Moreover, environmental conditions like weather or lighting can impact sensor performance.
Fog, rain, or snow can reduce the effectiveness of cameras and LiDAR systems, presenting challenges for accurate environment recognition.
Continuous advancements in sensor technologies are necessary to overcome these limitations.
Overcoming Challenges with Advanced Technologies
To address these challenges, ongoing research and development within the field of autonomous driving are vital.
Techniques such as machine learning and AI can improve data processing and decision-making in real time.
These technologies enable vehicles to learn from new data, adapting to unfamiliar scenarios and improving their performance over time.
Additionally, the development of high-definition maps with up-to-date and detailed information can enhance self-position estimation accuracy.
These maps provide a richer dataset for autonomous vehicles to draw from, reducing the reliance solely on sensor input.
Collaborative efforts from automotive manufacturers, technology companies, and regulatory bodies are essential in creating a robust framework for advancing these technologies.
The Future of Autonomous Driving
The future of autonomous driving promises a world where vehicles operate seamlessly without human intervention.
Self-position estimation and environment recognition are cornerstones for achieving this vision.
As these technologies continue to evolve, we can anticipate more reliable, efficient, and safer transportation systems.
With advancements in technology and increased adoption of autonomous vehicles, cities could transform into highly interconnected environments.
This shift not only optimizes traffic flow but also reduces congestion and emissions, contributing positively to urban living conditions.
The Role of Industry Stakeholders
Industry stakeholders play a crucial role in shaping the future of autonomous driving.
Collaborations between tech companies, car manufacturers, and policymakers are vital in standardizing technologies and regulations.
This cooperation ensures that innovations are aligned with legal and societal norms, facilitating smoother integration into everyday life.
Furthermore, public awareness and understanding of autonomous vehicles need emphasis.
As technology becomes more prevalent, educating communities about its benefits and safety measures is imperative.
In conclusion, the application of self-position estimation and surrounding environment recognition technologies is a transformative force in autonomous driving.
While challenges remain, collaborative efforts and technological advancements continue to pave the way toward a more autonomous future.
This evolution holds the promise of safer, more efficient, and environmentally friendly transportation systems for generations to come.
資料ダウンロード
QCD管理受発注クラウド「newji」は、受発注部門で必要なQCD管理全てを備えた、現場特化型兼クラウド型の今世紀最高の受発注管理システムとなります。
ユーザー登録
受発注業務の効率化だけでなく、システムを導入することで、コスト削減や製品・資材のステータス可視化のほか、属人化していた受発注情報の共有化による内部不正防止や統制にも役立ちます。
NEWJI DX
製造業に特化したデジタルトランスフォーメーション(DX)の実現を目指す請負開発型のコンサルティングサービスです。AI、iPaaS、および先端の技術を駆使して、製造プロセスの効率化、業務効率化、チームワーク強化、コスト削減、品質向上を実現します。このサービスは、製造業の課題を深く理解し、それに対する最適なデジタルソリューションを提供することで、企業が持続的な成長とイノベーションを達成できるようサポートします。
製造業ニュース解説
製造業、主に購買・調達部門にお勤めの方々に向けた情報を配信しております。
新任の方やベテランの方、管理職を対象とした幅広いコンテンツをご用意しております。
お問い合わせ
コストダウンが利益に直結する術だと理解していても、なかなか前に進めることができない状況。そんな時は、newjiのコストダウン自動化機能で大きく利益貢献しよう!
(β版非公開)