- お役立ち記事
- Application of sensor data acquisition and collection technology to abnormality detection using AI
月間76,176名の
製造業ご担当者様が閲覧しています*
*2025年3月31日現在のGoogle Analyticsのデータより
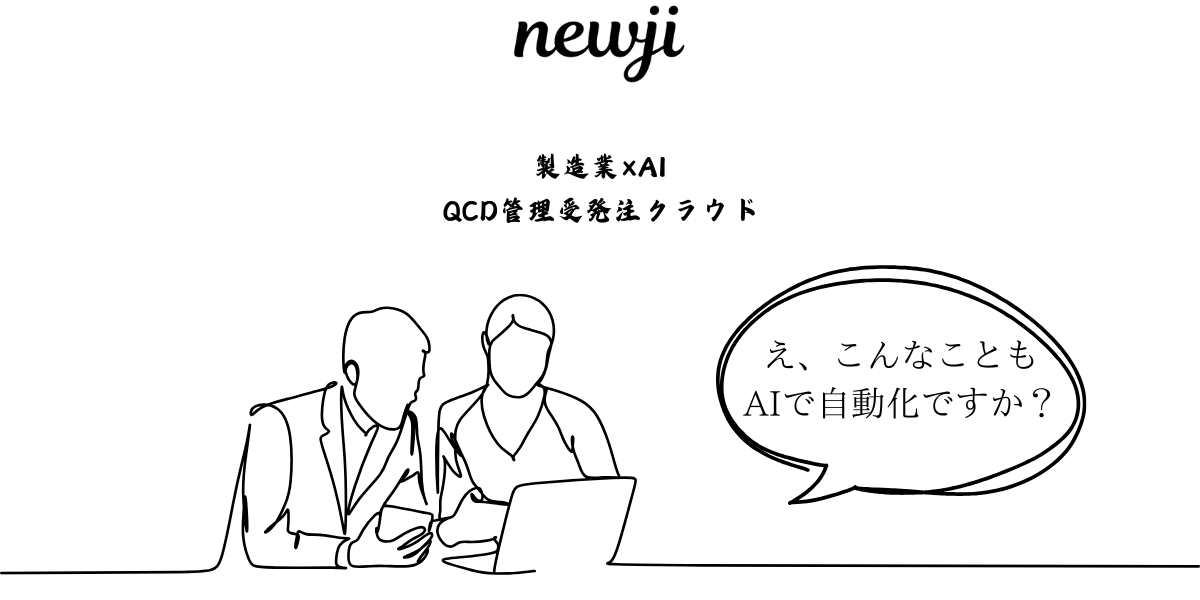
Application of sensor data acquisition and collection technology to abnormality detection using AI
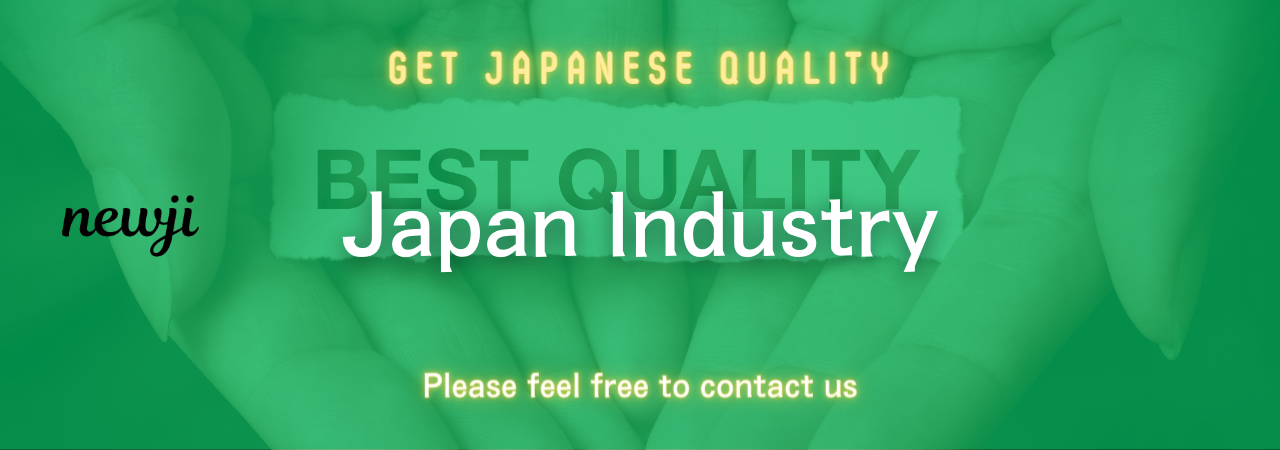
目次
Introduction to Sensor Data Acquisition
In today’s fast-paced world, technology plays an essential role in improving various aspects of our lives, including safety and efficiency in industrial settings.
One of the remarkable advancements in technology is the development of sensor data acquisition techniques combined with AI for the purpose of detecting anomalies.
Sensor data acquisition involves collecting and processing data from different sensor sources.
These sensors can detect physical quantities such as temperature, pressure, movement, and more.
The application of this technology is revolutionizing the field of anomaly detection.
What is Anomaly Detection?
Anomaly detection is a process used to identify patterns in data that do not conform to expected behavior.
This can include outliers, deviations, and errors that may signal malfunctions, fraud, or changes in the environment.
Traditional methods of anomaly detection relied heavily on pre-defined rules and thresholds set by human operators, which often led to inaccuracies.
However, with AI, specifically machine learning algorithms, anomaly detection has become more efficient and accurate.
Machine learning models can ingest large amounts of sensor data, learn from them, and improve over time to better detect anomalies.
The Role of AI in Anomaly Detection
AI plays a crucial role in transforming sensor data into actionable insights for anomaly detection.
Machine learning algorithms can analyze data in real-time, adapt to new scenarios, and provide predictive analytics.
By leveraging AI, systems can automatically detect abnormalities without human intervention, allowing for quicker response times and enhanced safety measures.
Machine Learning Techniques
Some popular machine learning techniques used in anomaly detection include supervised, unsupervised, and semi-supervised learning.
Supervised learning requires labeled datasets and is effective in environments where historical data is available for training.
Unsupervised learning, on the other hand, does not require labeled data and can identify anomalies in data where no prior examples exist.
Semi-supervised learning combines the best of both worlds by using a small amount of labeled data along with a large volume of unlabeled data.
Deep Learning Approaches
Deep learning, a subset of machine learning, involves neural networks with multiple layers.
It is particularly effective in handling large and complex datasets.
Techniques like autoencoders and recurrent neural networks (RNNs) are commonly used in anomaly detection tasks.
Autoencoders learn to compress data by encoding it into a lower-dimensional space and then reconstructing it.
Any significant difference in the reconstruction signifies an anomaly.
Recurrent neural networks are effective in handling time-series data, making them suitable for continuous sensor data that varies over time.
Sensor Technologies Enabling Anomaly Detection
Several sensor technologies are instrumental in enabling effective anomaly detection in various industries.
These technologies help in monitoring conditions and environments for any deviations from the norm.
Types of Sensors
The most common types of sensors used in anomaly detection include:
– Temperature Sensors: Measure temperature changes and detect overheating or undercooling, commonly used in manufacturing and power plants.
– Pressure Sensors: Used to measure liquid or gas pressure, often seen in pipelines and hydraulic systems.
– Motion Sensors: Detect movements and vibrations, widely used in security systems and industrial machinery.
– Proximity Sensors: Evaluate the distance of objects from the sensor, useful in automation and safety applications.
Wireless Sensor Networks
Wireless Sensor Networks (WSNs) consist of distributed sensors that wirelessly communicate with a central gateway.
These networks are advantageous for collecting data in remote and harsh environments where traditional wired systems may fail.
WSNs enhance anomaly detection by providing real-time data and flexibility to add new sensors as needed.
Internet of Things (IoT)
The IoT has a significant impact on sensor data acquisition and anomaly detection.
IoT devices can continuously monitor processes and send data to cloud-based platforms.
The integration of IoT with AI technologies enables further advancements in predictive maintenance and anomaly detection capabilities.
Applications of Anomaly Detection using AI
Anomaly detection using sensor data and AI is applied in a range of industries, providing immense benefits in diagnostics, forecasting, and preventive measures.
Manufacturing
In manufacturing, machines and assembly lines are equipped with various sensors for monitoring efficiency and safety.
AI-driven anomaly detection can identify potential equipment failures before they cause unplanned downtime.
This approach enhances predictive maintenance strategies and reduces operational costs.
Healthcare
Sensor data from medical devices can be analyzed to detect patient health anomalies.
Wearable sensors continuously collect data on vital signs and activities, enabling timely diagnosis and intervention of health issues.
This leads to improvements in patient care and personalized treatment plans.
Transportation
In the transportation sector, sensors are used in vehicles and infrastructure to monitor conditions and performance.
AI-based anomaly detection can improve traffic management, enhance vehicle safety, and prevent accidents by identifying erratic driving patterns or faulty components dynamically.
Environmental Monitoring
Environmental sensors collect data on air quality, water levels, and weather conditions.
Anomaly detection in this field can warn against natural disasters or pollution outbreaks, ensuring timely responses to protect ecosystems and communities.
Challenges and Future Directions
While sensor data acquisition and AI-driven anomaly detection provide immense opportunities, several challenges persist.
Data Management
The collection and processing of vast amounts of sensor data present challenges in storage and management.
Efficient data handling is necessary to ensure fast processing and accurate anomaly detection without delays.
Privacy Concerns
The use of sensors and AI involves collecting sensitive data, raising privacy and security concerns.
Responsible data handling and adherence to privacy laws and regulations are paramount to maintain user and public trust.
Interoperability
Different sensors and platforms may not always seamlessly integrate, leading to interoperability issues.
Standards and protocols must be developed to ensure diverse sensor systems work together cohesively.
Looking to the future, enhancements in AI algorithms, sensor technologies, and regulatory frameworks will continue to drive the evolution of anomaly detection.
Collaboration between industries, technology providers, and regulatory bodies will be crucial in unlocking the full potential of these advancements.
In conclusion, the application of sensor data acquisition and collection technology to anomaly detection using AI is transforming industries worldwide.
The combination of advanced sensors with AI techniques is making our environments smarter, safer, and more efficient.
Embracing these technologies today will pave the way for enduring future innovations.
資料ダウンロード
QCD管理受発注クラウド「newji」は、受発注部門で必要なQCD管理全てを備えた、現場特化型兼クラウド型の今世紀最高の受発注管理システムとなります。
ユーザー登録
受発注業務の効率化だけでなく、システムを導入することで、コスト削減や製品・資材のステータス可視化のほか、属人化していた受発注情報の共有化による内部不正防止や統制にも役立ちます。
NEWJI DX
製造業に特化したデジタルトランスフォーメーション(DX)の実現を目指す請負開発型のコンサルティングサービスです。AI、iPaaS、および先端の技術を駆使して、製造プロセスの効率化、業務効率化、チームワーク強化、コスト削減、品質向上を実現します。このサービスは、製造業の課題を深く理解し、それに対する最適なデジタルソリューションを提供することで、企業が持続的な成長とイノベーションを達成できるようサポートします。
製造業ニュース解説
製造業、主に購買・調達部門にお勤めの方々に向けた情報を配信しております。
新任の方やベテランの方、管理職を対象とした幅広いコンテンツをご用意しております。
お問い合わせ
コストダウンが利益に直結する術だと理解していても、なかなか前に進めることができない状況。そんな時は、newjiのコストダウン自動化機能で大きく利益貢献しよう!
(β版非公開)