- お役立ち記事
- Application of sensor data analysis and anomaly detection using AI/IoT, and success points of the utilization promotion project
月間76,176名の
製造業ご担当者様が閲覧しています*
*2025年3月31日現在のGoogle Analyticsのデータより
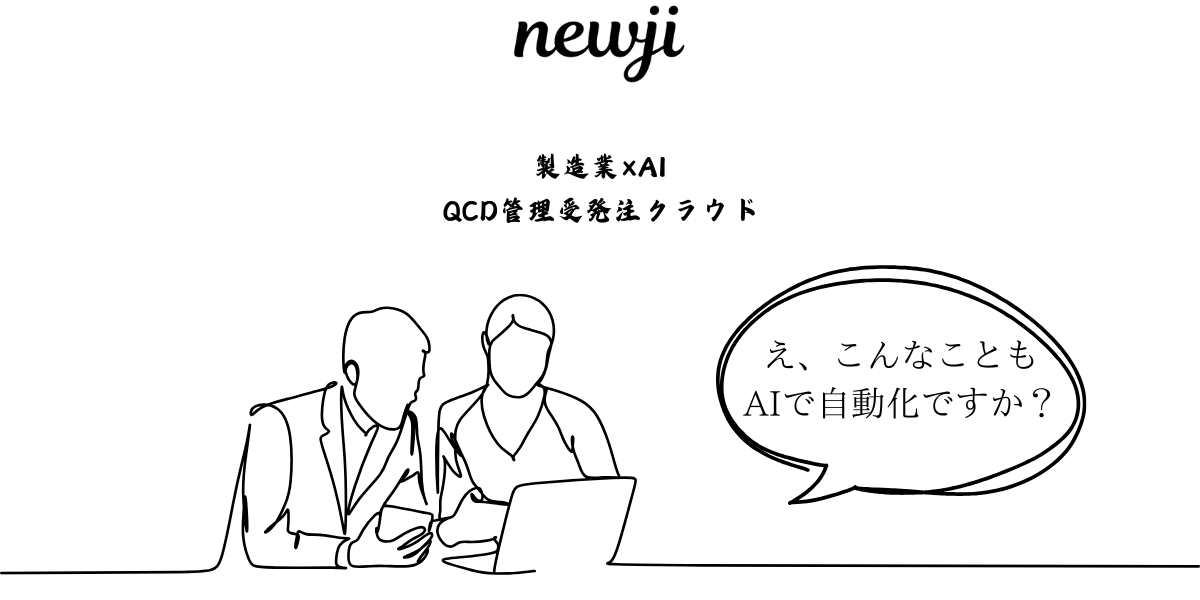
Application of sensor data analysis and anomaly detection using AI/IoT, and success points of the utilization promotion project
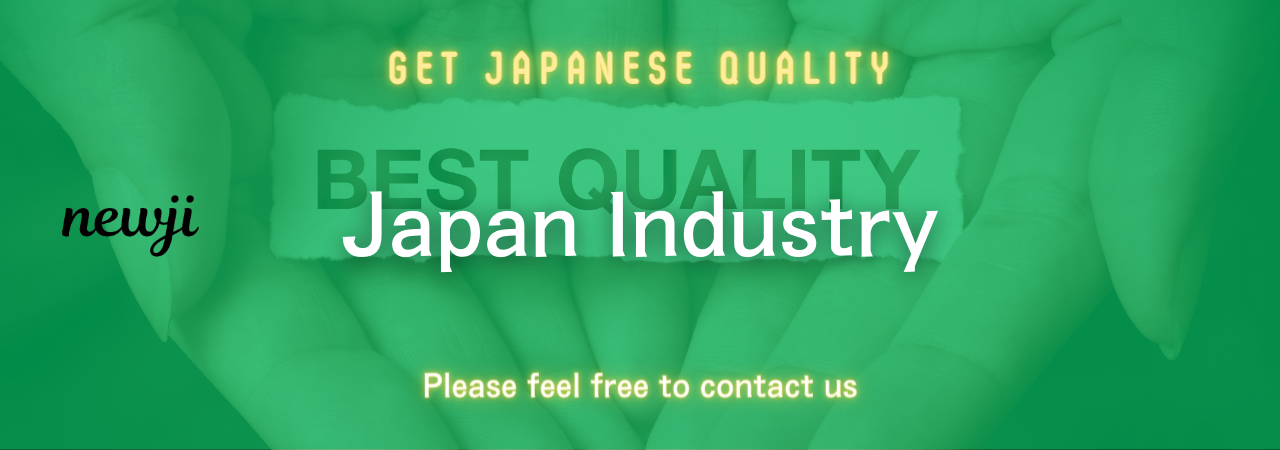
目次
Understanding Sensor Data Analysis
The world is rapidly advancing and with it, the innovative technology of IoT (Internet of Things) has been integrated into our daily lives, businesses, and industries.
One of the most remarkable advancements in this field is the application of sensor data analysis.
Sensors, as part of IoT devices, collect real-time data and transmit it for analysis.
This data, when effectively analyzed using AI, can provide valuable insights, drive decision-making, and power automation processes.
The data collected from sensors are diverse, ranging from temperature, humidity, speed, motion, to various other parameters depending on the application.
For example, in a manufacturing setting, sensors might monitor machine performance, detect wear and tear, and ensure operational efficiency.
In smart homes, sensors detect movements, control appliances, and optimize energy usage.
The analysis of this data helps in maximizing efficiencies, reducing downtime, and ensuring safety and compliance.
Anomaly Detection in Sensor Data
As IoT devices proliferate, the amount of data that needs to be analyzed grows exponentially.
This is where AI-based anomaly detection comes into play.
Anomaly detection refers to the process of identifying unusual patterns that do not conform to expected behavior.
These patterns, if left unchecked, can indicate problems such as potential equipment failure, security breaches, or operational inefficiencies.
AI can sift through large datasets at a remarkable speed and accuracy, identifying anomalies that human analysts might overlook.
Machine learning algorithms can be trained to recognize what “normal” looks like for a particular system and alert users when deviations occur.
For instance, in healthcare, anomaly detection might identify deviations in a patient’s vital signs, enabling early intervention and proactive care.
Success in Utilizing AI and IoT Anomaly Detection
Utilizing sensor data analysis and anomaly detection successfully involves several critical steps.
Firstly, it requires a clear understanding of the objectives.
Organizations must identify what they aim to achieve with data analysis and determine the most relevant data types.
Secondly, the quality and volume of data are crucial.
An effective data analytics initiative depends on the integrity and completeness of the data collected.
IoT devices should be strategically placed and maintained to ensure seamless data flow.
Thirdly, leveraging the power of machine learning is essential.
Organizations need to select appropriate algorithms that can learn and adapt over time.
It is equally critical to regularly update and tweak these algorithms as new data becomes available.
Finally, successful projects require collaboration across departments.
Involving stakeholders and encouraging cross-functional teams to collaborate can lead to more innovative solutions and successful outcomes.
Case Studies of Successful Projects
Several notable success stories underscore the potential of applying AI and IoT to sensor data analysis.
In the automotive sector, predictive maintenance has drastically reduced downtime in vehicle fleets.
By constantly monitoring various vehicle parameters, companies like Tesla can predict when a part is likely to fail and intervene before it causes costly disruptions.
Similarly, in agriculture, IoT sensors and AI are optimizing crop yield by precisely monitoring soil conditions and weather parameters.
Projects such as these have shown a significant increase in productivity and cuts in resource usage, benefitting both farmers and the environment.
In the logistics industry, anomaly detection is transforming supply chain management.
Companies like Amazon use real-time data to track parcels, anticipate delays, and optimize routes, ensuring swift and reliable delivery services.
By identifying patterns indicative of delays or disruptions, they can proactively address issues, significantly improving customer satisfaction.
Future Trends in AI and IoT
As technology continues to evolve, the application of AI and IoT is expected to expand into even more sectors.
With the advent of 5G technology, IoT devices will become faster and more efficient, enabling more comprehensive data collection and analysis.
The potential of edge computing is another promising trend.
By processing data closer to the source, edge computing can reduce latency and improve response times, making real-time decisions more feasible.
This will be especially beneficial in time-critical applications such as autonomous driving or remote surgery.
Moreover, advancements in cybersecurity will enhance the safety and reliability of IoT systems, ensuring that data and systems are protected against malicious attacks.
Conclusion
The application of sensor data analysis and anomaly detection through AI and IoT holds immense potential for innovation and efficiency.
When approached with clear objectives, robust data, and a commitment to leveraging the right technology, the benefits can be transformative.
By examining successful case studies and anticipating future trends, businesses can better prepare to harness these technologies for sustained growth and competitive advantage.
It’s clear that as we advance, embracing AI and IoT innovations will be key to staying ahead in an increasingly data-driven world.
資料ダウンロード
QCD管理受発注クラウド「newji」は、受発注部門で必要なQCD管理全てを備えた、現場特化型兼クラウド型の今世紀最高の受発注管理システムとなります。
ユーザー登録
受発注業務の効率化だけでなく、システムを導入することで、コスト削減や製品・資材のステータス可視化のほか、属人化していた受発注情報の共有化による内部不正防止や統制にも役立ちます。
NEWJI DX
製造業に特化したデジタルトランスフォーメーション(DX)の実現を目指す請負開発型のコンサルティングサービスです。AI、iPaaS、および先端の技術を駆使して、製造プロセスの効率化、業務効率化、チームワーク強化、コスト削減、品質向上を実現します。このサービスは、製造業の課題を深く理解し、それに対する最適なデジタルソリューションを提供することで、企業が持続的な成長とイノベーションを達成できるようサポートします。
製造業ニュース解説
製造業、主に購買・調達部門にお勤めの方々に向けた情報を配信しております。
新任の方やベテランの方、管理職を対象とした幅広いコンテンツをご用意しております。
お問い合わせ
コストダウンが利益に直結する術だと理解していても、なかなか前に進めることができない状況。そんな時は、newjiのコストダウン自動化機能で大きく利益貢献しよう!
(β版非公開)