- お役立ち記事
- Application of sparse modeling to optimization problems and system control
Application of sparse modeling to optimization problems and system control
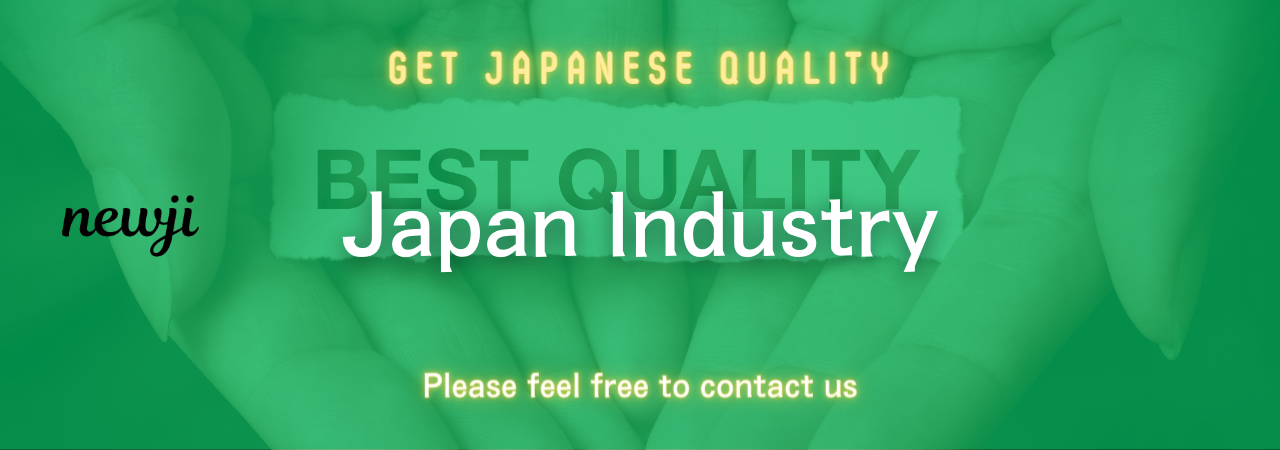
目次
Understanding Sparse Modeling
Sparse modeling is a powerful mathematical technique that simplifies complex data sets.
It focuses on identifying the most significant features within a data structure, which can sometimes contain a large amount of redundant or irrelevant information.
By isolating these key components, sparse modeling helps make predictive models more efficient and effective in various fields such as data analysis, image processing, and system control.
The central idea behind sparse modeling is that many large-scale data or signals can be approximated using only a few non-zero coefficients.
This approach not only reduces the dimensionality of the data but also highlights the most critical elements essential for accurate analysis or control.
By doing so, sparse models can provide more understandable insights and offer computational benefits by decreasing storage and processing requirements.
Optimization Problems in Sparse Modeling
Sparse modeling is particularly relevant in solving optimization problems.
Optimization involves finding the best solution or outcome in a mathematical model, and sparse modeling aids by reducing the complexity of these models.
In optimization problems, we often deal with a vast number of variables, many of which may not significantly impact the final solution.
By applying sparse modeling, we can focus on the variables that matter most, making the solution process more efficient.
Consider the field of machine learning, where sparse modeling is widely used to enhance model performance.
In machine learning, particularly in algorithms like Lasso regression, sparse modeling selects a subset of predictors by imposing a constraint that limits the size of the coefficients.
This not only simplifies the model but also prevents overfitting, leading to a more robust and interpretable model.
Furthermore, sparse modeling plays a crucial role in compressed sensing, a technique aiming to efficiently reconstruct a signal.
This technique uses optimization algorithms to recover a signal from a small number of measurements, which is particularly practical in resource-constrained environments.
System Control and Sparse Modeling
In system control, sparse modeling offers substantial improvements in the development and operation of control systems.
Control systems manage, command, direct, or regulate the behavior of other devices or systems.
Using sparse modeling, engineers can design control systems that respond to actual needs rather than unnecessary complexities, enhancing the precision and stability of these systems.
One primary application is in the design of model predictive control (MPC).
MPC is a type of control system that uses a dynamic model to predict the future state of a process to make decisions.
Sparse modeling can refine the MPC algorithm by narrowing down the model to its most critical components, leading to faster and more accurate predictions.
Moreover, in the realm of electrical power systems, sparse modeling assists in state estimation processes by reducing the computational burden of estimating the state of a power grid.
This reduction is achieved by focusing only on the most crucial grid measurements while still maintaining system reliability and performance.
Another area where sparse modeling makes an impact is in the realm of networked control systems.
These systems rely on communication networks to transmit information and commands between controllers and devices.
Sparse modeling reduces the amount of data that needs to be communicated, enhancing the system’s efficiency and reducing latency.
Benefits of Sparse Modeling in Optimization and Control
The application of sparse modeling to optimization problems and system control brings several advantages.
Firstly, it reduces the computational cost because fewer variables mean fewer calculations, which is particularly beneficial for real-time applications.
This reduction can lead to faster computation times, which is crucial in scenarios demanding speed and efficiency, such as in autonomous vehicle navigation or real-time financial modeling.
Secondly, by focusing on essential information, sparse modeling improves the interpretability of models.
Simplified models are easier to understand and communicate to diverse stakeholders, including those with limited technical backgrounds.
This clarity can facilitate better decision-making and trust in automated systems.
Another significant benefit is the enhancement of the robustness of optimization models and control systems.
Models that rely on fewer predictors are less susceptible to noise and overfitting, increasing their ability to generalize to unseen data and conditions.
This robustness ensures dependable and consistent performance across various scenarios.
Challenges and Future Directions
Despite the clear advantages, sparse modeling also presents challenges.
The primary challenge is determining the optimal level of sparsity—balancing enough data reduction without oversimplifying and losing critical information.
Choosing the right parameters and methods for sparse modeling can be complex and often requires significant expertise.
Another challenge lies in real-world implementation.
Most theories in sparse modeling assume ideal conditions that may not always exist in practical scenarios.
Noise and incomplete data can affect the modeling process, necessitating careful validation and adjustment.
Looking ahead, the intersection of sparse modeling with emerging technologies like artificial intelligence and quantum computing offers exciting prospects.
These advances can provide new tools for optimizing sparse models and applying them to more complex and dynamic systems.
Research in these areas continues to explore novel algorithms and methods to address current limitations and extend the reach of sparse modeling to broader applications.
Conclusion
In conclusion, sparse modeling is a transformative approach that provides remarkable benefits in solving optimization problems and enhancing system control.
By focusing on the most critical components, sparse modeling improves computation efficiency, model interpretability, and system robustness.
Despite challenges, the ongoing development and integration of sparse modeling with cutting-edge technologies promise a bright future in many fields, making it an invaluable tool for researchers and practitioners alike.
資料ダウンロード
QCD調達購買管理クラウド「newji」は、調達購買部門で必要なQCD管理全てを備えた、現場特化型兼クラウド型の今世紀最高の購買管理システムとなります。
ユーザー登録
調達購買業務の効率化だけでなく、システムを導入することで、コスト削減や製品・資材のステータス可視化のほか、属人化していた購買情報の共有化による内部不正防止や統制にも役立ちます。
NEWJI DX
製造業に特化したデジタルトランスフォーメーション(DX)の実現を目指す請負開発型のコンサルティングサービスです。AI、iPaaS、および先端の技術を駆使して、製造プロセスの効率化、業務効率化、チームワーク強化、コスト削減、品質向上を実現します。このサービスは、製造業の課題を深く理解し、それに対する最適なデジタルソリューションを提供することで、企業が持続的な成長とイノベーションを達成できるようサポートします。
オンライン講座
製造業、主に購買・調達部門にお勤めの方々に向けた情報を配信しております。
新任の方やベテランの方、管理職を対象とした幅広いコンテンツをご用意しております。
お問い合わせ
コストダウンが利益に直結する術だと理解していても、なかなか前に進めることができない状況。そんな時は、newjiのコストダウン自動化機能で大きく利益貢献しよう!
(Β版非公開)