- お役立ち記事
- Application technology of machine learning for abnormality detection and predictive maintenance and its key points
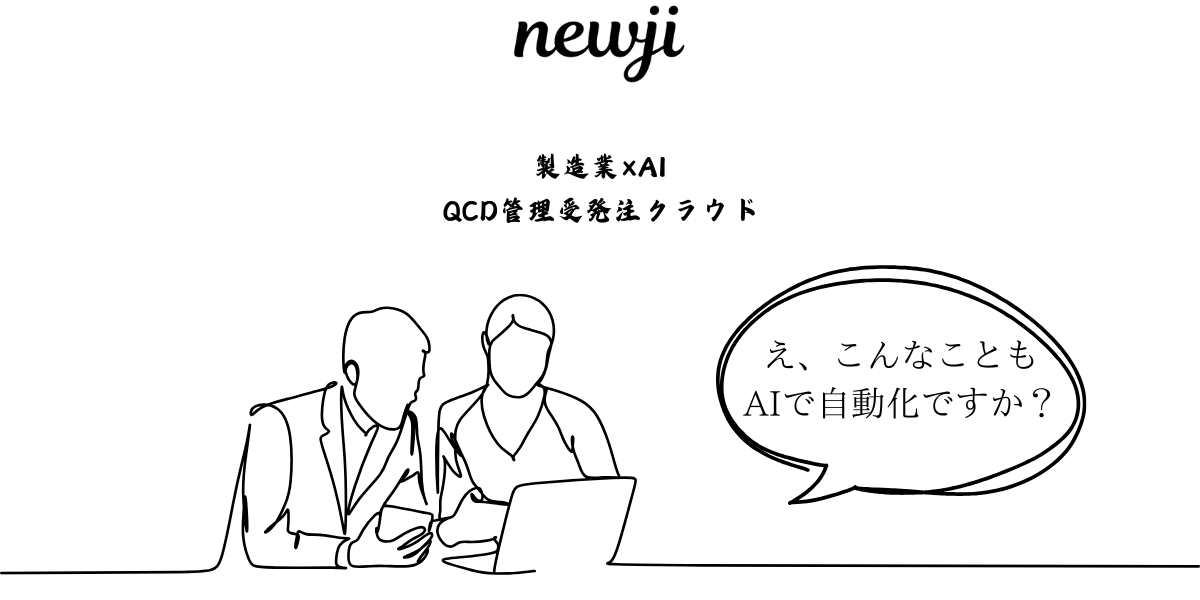
Application technology of machine learning for abnormality detection and predictive maintenance and its key points
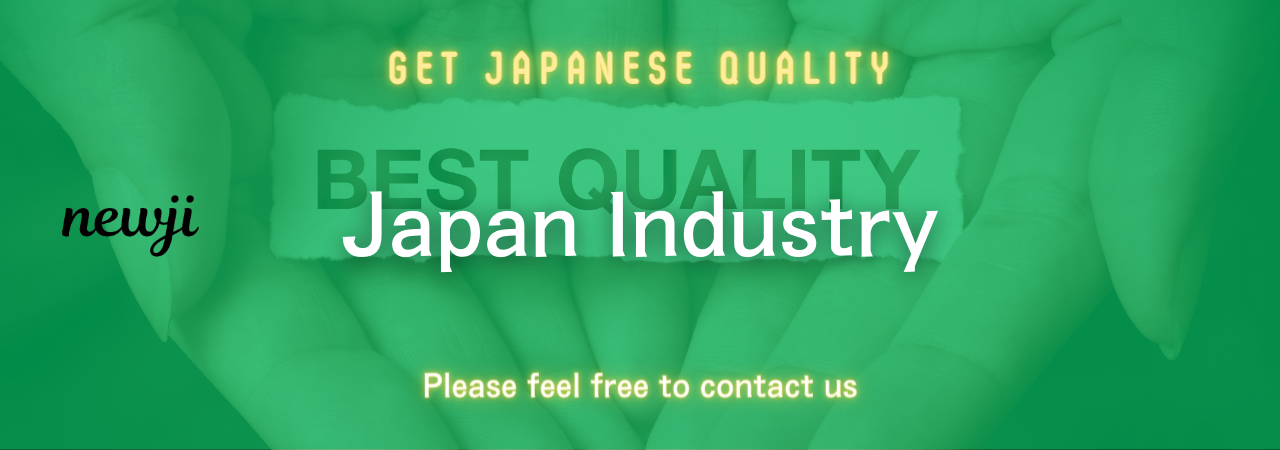
目次
Introduction to Machine Learning in Industrial Settings
Machine learning has revolutionized the way industries operate, particularly in areas like abnormality detection and predictive maintenance.
These applications harness the power of algorithms to process vast amounts of data, identify patterns, and make predictions that can prevent costly downtimes or dangerous failures.
Understanding machine learning’s application in these areas can offer significant improvements in efficiency and safety.
What is Abnormality Detection?
Abnormality detection is the process of identifying unusual patterns that do not conform to expected behavior.
In industrial environments, this could mean detecting fluctuations in equipment performance that might indicate a fault or failure.
Machine learning is particularly adept at this task because it can analyze data continuously and recognize subtle changes before they become significant problems.
The Role of Machine Learning
Machine learning can enhance abnormality detection by learning from historical data.
This involves using algorithms to build a model of what normal operation looks like and then identifying deviations from this model.
For example, in a manufacturing plant, machine learning can monitor vibrations, temperature, or sound levels, and flag any readings that deviate from the norm.
Benefits of Using Machine Learning for Abnormality Detection
The primary benefit of machine learning in abnormality detection is its ability to offer real-time monitoring and analysis.
This means industries can identify and address issues much faster than with traditional methods.
Additionally, machine learning models can improve over time, increasing their accuracy and reducing false alarms.
This leads to more reliable operations and can extend the lifespan of equipment by preventing damage.
Predictive Maintenance and Its Importance
Predictive maintenance uses data-driven analysis to predict when equipment will require maintenance.
Instead of relying on scheduled maintenance, predictive maintenance helps industries perform maintenance only when necessary, based on the current condition of equipment.
This can lead to significant cost savings and improve operational efficiency.
Machine Learning Techniques for Predictive Maintenance
Several machine learning techniques are employed for predictive maintenance, including supervised learning, unsupervised learning, and reinforcement learning.
Supervised learning involves using labeled data to train models, which can then predict future equipment failures.
Unsupervised learning can find hidden patterns in data without prior labeling, which is useful for detecting unknown problems.
Reinforcement learning, while more complex, can optimize maintenance schedules by learning strategies to minimize downtime and repair costs.
Advantages of Machine Learning in Predictive Maintenance
Machine learning enables predictive maintenance to be more accurate and timely.
By analyzing data from previous maintenance events, machine learning models can predict when equipment will fail with greater precision.
This prevents unnecessary maintenance actions, reduces downtime, and often results in substantial cost savings.
Moreover, machine learning can help companies optimize their inventory by forecasting the need for spare parts and other resources.
Key Points for Implementing Machine Learning
Successfully implementing machine learning for abnormality detection and predictive maintenance requires careful planning and execution.
Data Collection and Management
The quality of predictions directly depends on the quality of data.
Hence, it is crucial to set up reliable data collection systems that can capture relevant parameters accurately.
This data should be diverse, covering different aspects of equipment operation, and should be stored in a manner that facilitates easy access and processing.
Model Selection and Training
Choosing the right model depends on the specific needs of an application.
It requires understanding the nature of the problem, the type of data available, and the goals of the analysis.
Training these models necessitates significant computational resources and expertise in machine learning to ensure that they learn effectively and produce reliable predictions.
Continuous Monitoring and Updating
Once implemented, machine learning models require continuous monitoring to ensure they perform as expected.
Data streams should be continuously fed to models, and models themselves may need adjustments or retraining to accommodate new patterns or changes in equipment behavior.
This ongoing process allows models to adapt and maintain their effectiveness over time.
Challenges in Implementation
Despite the benefits, there are several challenges when implementing machine learning in industrial settings.
Integration with Existing Systems
Machine learning models need to integrate seamlessly with existing systems and processes.
This may require significant changes or upgrades in IT infrastructure and collaborating with various departments to align objectives and resources.
Data Security and Privacy
Handling sensitive data poses security risks.
Companies must ensure robust data protection measures are in place to comply with regulations and to safeguard against breaches.
Lack of Expertise
There is often a skills gap in many organizations when it comes to machine learning.
Investing in training or hiring skilled professionals is essential to successfully implement and maintain machine learning applications.
Conclusion
Machine learning offers powerful tools for abnormality detection and predictive maintenance, transforming how industries operate.
While there are challenges in implementation, the benefits far outweigh the hurdles, leading to increased efficiency, reduced costs, and enhanced safety.
By focusing on key aspects such as quality data collection, model selection, and continuous improvement, industries can harness the full potential of machine learning to maintain optimal operations.
資料ダウンロード
QCD調達購買管理クラウド「newji」は、調達購買部門で必要なQCD管理全てを備えた、現場特化型兼クラウド型の今世紀最高の購買管理システムとなります。
ユーザー登録
調達購買業務の効率化だけでなく、システムを導入することで、コスト削減や製品・資材のステータス可視化のほか、属人化していた購買情報の共有化による内部不正防止や統制にも役立ちます。
NEWJI DX
製造業に特化したデジタルトランスフォーメーション(DX)の実現を目指す請負開発型のコンサルティングサービスです。AI、iPaaS、および先端の技術を駆使して、製造プロセスの効率化、業務効率化、チームワーク強化、コスト削減、品質向上を実現します。このサービスは、製造業の課題を深く理解し、それに対する最適なデジタルソリューションを提供することで、企業が持続的な成長とイノベーションを達成できるようサポートします。
オンライン講座
製造業、主に購買・調達部門にお勤めの方々に向けた情報を配信しております。
新任の方やベテランの方、管理職を対象とした幅広いコンテンツをご用意しております。
お問い合わせ
コストダウンが利益に直結する術だと理解していても、なかなか前に進めることができない状況。そんな時は、newjiのコストダウン自動化機能で大きく利益貢献しよう!
(Β版非公開)