- お役立ち記事
- Application to self-position estimation technology and surrounding environment recognition technology in autonomous driving
Application to self-position estimation technology and surrounding environment recognition technology in autonomous driving
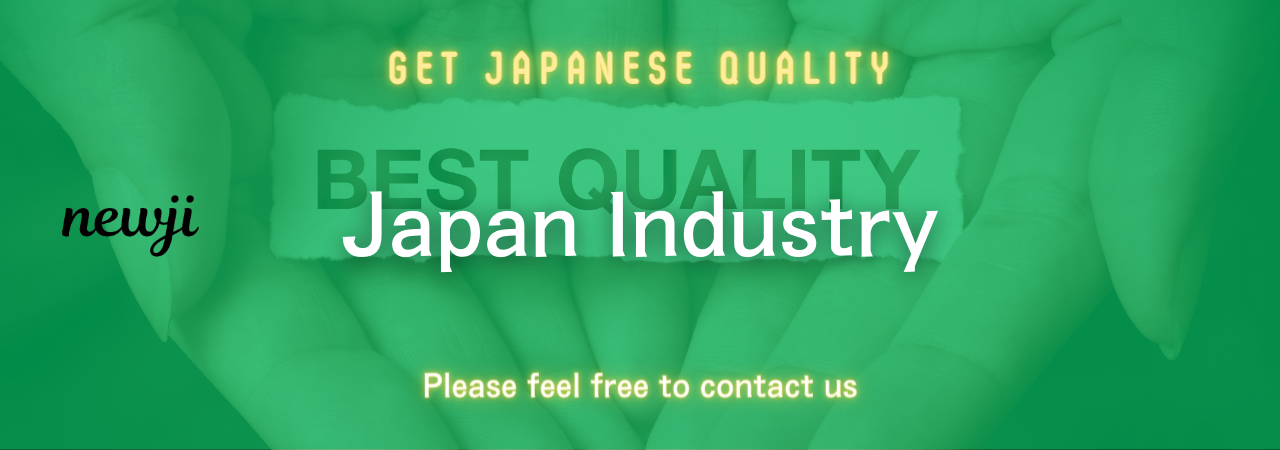
目次
Introduction to Autonomous Driving
Autonomous driving technology has been a hot topic in recent years, as it promises to transform the way we commute, deliver goods, and manage traffic.
A critical aspect of this technology involves the precise estimation of the vehicle’s self-position and the recognition of the surrounding environment.
Both elements are essential to ensure the safety and efficiency of autonomous vehicles.
In this article, we will explore the applications of self-position estimation and environment recognition technologies in the realm of autonomous driving.
Understanding Self-Position Estimation
Self-position estimation refers to the vehicle’s ability to accurately determine its location within a given environment.
This is achieved through a combination of sensors, mapping technologies, and algorithms that work together to provide real-time location data.
Accurate self-position estimation is crucial for autonomous vehicles, as it guides the vehicle’s navigation and decision-making processes.
Role of GPS in Self-Position Estimation
The Global Positioning System (GPS) is one of the primary technologies used for self-position estimation.
GPS satellites provide location data that can pinpoint a vehicle’s position to within a few meters.
However, GPS alone is not always sufficient, particularly in urban environments where signals can be obstructed by tall buildings or tunnels.
This is where additional technologies come into play.
Integration of LiDAR and Cameras
To complement GPS, autonomous vehicles use sensors like LiDAR (Light Detection and Ranging) and cameras.
LiDAR uses laser light to create detailed 3D maps of the vehicle’s surroundings, allowing it to accurately measure distances to nearby objects.
Cameras, on the other hand, provide visual data that can be processed to identify road markings, signs, and other visual cues vital for navigation.
Incorporating Inertial Measurement Units (IMUs)
Inertial Measurement Units (IMUs) are another key component of self-position estimation.
They measure the vehicle’s acceleration and rotation, providing data that helps calculate the vehicle’s movement from a known position.
By integrating IMU data with information from GPS, LiDAR, and cameras, autonomous systems can maintain accurate positional awareness even in challenging conditions.
Recognizing the Surrounding Environment
In addition to knowing their position, autonomous vehicles must understand their surroundings to operate safely.
This involves identifying and interpreting various elements of the environment, such as roads, pedestrians, other vehicles, and obstacles.
Environment recognition leverages advanced technologies and methods to achieve this comprehensive understanding.
Machine Learning and Computer Vision
Machine learning and computer vision play pivotal roles in environment recognition.
These technologies enable the system to interpret images captured by cameras or LiDAR and classify objects based on patterns learned through extensive training.
Machine learning models, such as neural networks, are trained on vast datasets to recognize objects ranging from traffic lights to bicycles.
Importance of Semantic Segmentation
Semantic segmentation is a technique used to divide an image into regions based on the objects they contain.
This process assigns labels to each pixel, allowing the autonomous system to distinguish between roads, sidewalks, and other critical features.
Semantic segmentation helps inform decisions, like adjusting speed according to traffic conditions or changing lanes safely.
Role of Radar in Environment Perception
Radar technology is also critical for environment perception, particularly in distinguishing object motion.
Unlike LiDAR and cameras, radar can detect objects irrespective of lighting conditions and environmental factors like fog or rain.
It provides velocity data, which helps predict the movement of surrounding objects, making it indispensable for collision avoidance and safe navigation.
Applications in Autonomous Vehicles
Combining self-position estimation with environment recognition technologies enables an array of applications in autonomous vehicles.
Enhancing Navigation Systems
Self-position estimation and environment recognition technologies significantly enhance the navigation systems of autonomous vehicles.
By accurately understanding their position and surroundings, vehicles can execute precise maneuvers, follow pre-determined routes, and dynamically adjust to traffic conditions.
These capabilities ultimately improve travel efficiency and reduce journey times.
Ensuring Passenger Safety
One of the primary goals of autonomous driving is to enhance passenger safety.
Self-position estimation ensures vehicles stay within their lanes and avoid collisions with stationary objects.
Simultaneously, environment recognition systems detect potential hazards, such as errant pedestrians or upcoming obstacles, prompting preventive actions to mitigate risks.
Facilitating Seamless Interaction with Other Vehicles
Autonomous vehicles can interact seamlessly with other road users when equipped with robust self-position estimation and environment recognition systems.
For instance, vehicle-to-vehicle communication enables them to share positional and environmental data, optimizing traffic flow and minimizing the chances of accidents.
Challenges and Future Directions
While advancements in self-position estimation and environment recognition have been substantial, challenges remain.
These include ensuring reliability in adverse weather, managing large data volumes, and enhancing real-time processing capabilities.
Addressing Adverse Weather Conditions
Ensuring accurate performance during adverse weather conditions, such as heavy rain or snow, is a challenge that requires further innovation.
Systems must be robust enough to handle signal interference and maintain accuracy across diverse environmental conditions.
Data Processing and Storage
Autonomous vehicles generate massive amounts of data from their sensors, posing challenges for processing and storage.
Future advancements will likely focus on optimizing data management and developing efficient algorithms capable of real-time processing.
Ongoing Research and Development
The evolution of autonomous driving systems hinges on ongoing research and development efforts.
As new technologies emerge and existing ones improve, we can expect further enhancements in self-position estimation and environment recognition, pushing us closer to achieving fully autonomous, safe, and efficient transportation.
In conclusion, self-position estimation and environment recognition technologies are at the heart of autonomous driving innovations.
Their applications ensure precise navigation, enhance safety, and enable seamless interaction with the environment, paving the way for the future of transportation.
資料ダウンロード
QCD調達購買管理クラウド「newji」は、調達購買部門で必要なQCD管理全てを備えた、現場特化型兼クラウド型の今世紀最高の購買管理システムとなります。
ユーザー登録
調達購買業務の効率化だけでなく、システムを導入することで、コスト削減や製品・資材のステータス可視化のほか、属人化していた購買情報の共有化による内部不正防止や統制にも役立ちます。
NEWJI DX
製造業に特化したデジタルトランスフォーメーション(DX)の実現を目指す請負開発型のコンサルティングサービスです。AI、iPaaS、および先端の技術を駆使して、製造プロセスの効率化、業務効率化、チームワーク強化、コスト削減、品質向上を実現します。このサービスは、製造業の課題を深く理解し、それに対する最適なデジタルソリューションを提供することで、企業が持続的な成長とイノベーションを達成できるようサポートします。
オンライン講座
製造業、主に購買・調達部門にお勤めの方々に向けた情報を配信しております。
新任の方やベテランの方、管理職を対象とした幅広いコンテンツをご用意しております。
お問い合わせ
コストダウンが利益に直結する術だと理解していても、なかなか前に進めることができない状況。そんな時は、newjiのコストダウン自動化機能で大きく利益貢献しよう!
(Β版非公開)