- お役立ち記事
- Applications of multivariate analysis and product quality improvement that statistical technology departments should know
Applications of multivariate analysis and product quality improvement that statistical technology departments should know
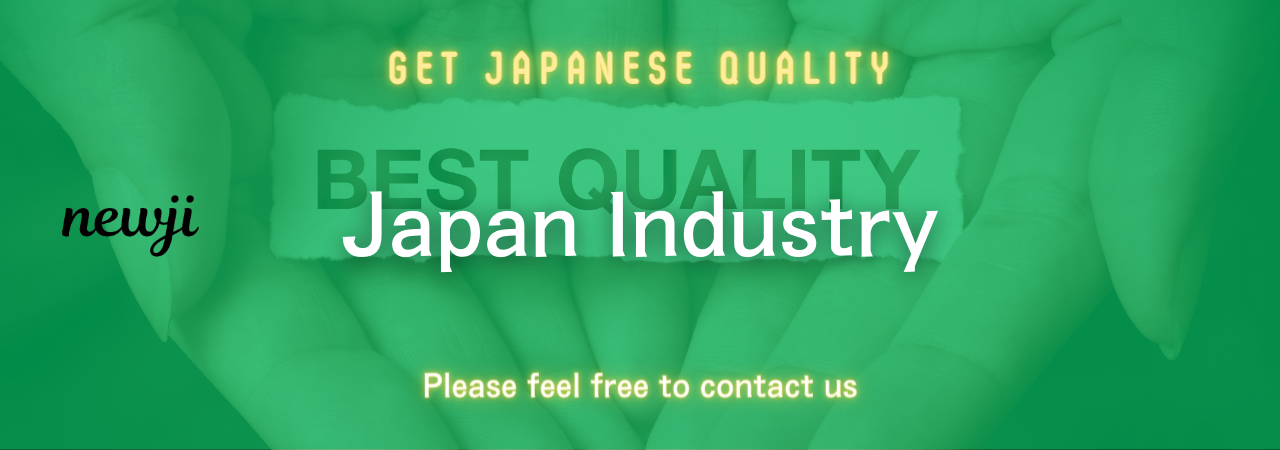
目次
Understanding Multivariate Analysis
Multivariate analysis is a statistical technique that allows us to understand relationships between multiple variables simultaneously.
Unlike univariate analysis, which focuses on one variable at a time, multivariate analysis helps us see the bigger picture by analyzing patterns and relationships in data with multiple dimensions.
In the context of product quality improvement, this technique is invaluable.
It helps identify important factors that influence the quality and performance of a product, enabling companies to make data-driven decisions to enhance quality control processes.
Types of Multivariate Analysis
There are several different types of multivariate analysis techniques, each suited for specific situations and data types.
A few common ones include:
Principal Component Analysis (PCA)
PCA is a method used to reduce the dimensionality of a data set while preserving as much variability as possible.
It does so by transforming the data into a new set of variables, or principal components, which are uncorrelated and ordered by the amount of variance they capture from the data.
This method is particularly useful in simplifying complex data sets and revealing hidden structures by reducing noise.
Cluster Analysis
Cluster analysis is another technique often used in multivariate analysis.
It involves grouping a set of objects in such a way that objects in the same group, or cluster, are more similar to each other than to those in other clusters.
This technique is helpful in market segmentation, image processing, and biological research.
In product quality improvement, it can be used to segment data into groups of similar defect types to identify common failure modes.
Factor Analysis
Factor analysis seeks to identify underlying relationships between variables by modeling latent variables, or factors, which influence the observed measurements.
In product development, factor analysis helps determine the key underlying factors that affect product quality, allowing companies to focus on the most impactful areas for improvement.
Key Applications in Product Quality Improvement
The application of multivariate analysis in product quality improvement is extensive and impactful.
By analyzing multiple variables simultaneously, businesses can make more informed decisions regarding product manufacturing and design.
Identifying Critical Quality Factors
One primary application of multivariate analysis in quality improvement is identifying critical factors that contribute to product defects or variability.
Through techniques like PCA and factor analysis, companies can determine which factors exhibit the highest influence on quality.
This information helps prioritize process improvement efforts, focusing on areas that promise significant gains in quality outcomes.
Enhancing Process Optimization
With the insights gained from multivariate analysis, businesses can optimize production processes.
For instance, by conducting a cluster analysis, manufacturers can identify patterns among production batches that exhibit similar quality levels.
These insights help them adjust process parameters, like temperature, time, or pressure, to enhance product consistency.
Quality Monitoring and Control
Multivariate methods allow for real-time quality monitoring and control by using statistical process control (SPC) in a multivariate context.
Unlike traditional SPC, which monitors one quality characteristic at a time, multivariate SPC can monitor several quality characteristics simultaneously.
It provides a more comprehensive view of the production process, ensuring issues are detected early and addressed promptly.
Reducing Waste and Costs
By accurately identifying factors that impact product quality, companies can notably reduce manufacturing defects, waste, and associated costs.
Emphasizing data-driven improvements leads to fewer product recalls, reduced rework, lower warranty claims, and ultimately, increased customer satisfaction and loyalty.
Implementing Multivariate Analysis in Statistical Technology Departments
For organizations that strive to improve product quality through statistical technology, incorporating multivariate analysis can be transformative.
Training and Skill Development
To successfully implement multivariate analysis techniques, statistical technology departments must ensure their team members are well-trained in these methods.
This could involve offering workshops, certification programs, and courses focused on multivariate analysis.
Having skilled analysts on board enhances the ability to derive actionable insights from complex data sets, which are increasingly common in today’s data-driven world.
Utilizing Appropriate Software Tools
Handling large data sets with multiple variables requires robust software solutions capable of efficiently performing multivariate analysis.
Departments should ensure access to specialized software like R, SAS, SPSS, or Python packages that are designed to manage and analyze complex data effectively.
These tools streamline the analytical process and provide visual outputs, making it easier to interpret and communicate findings across teams.
Collaboration and Communication
Effective implementation involves collaboration among cross-functional teams, including design, production, and marketing.
Statistical technology departments should work closely with these teams to understand specific needs and quality objectives, ensuring that multivariate analysis aligns with organizational goals.
Clear communication of findings and recommendations is essential, which may involve producing comprehensive reports and presentations for stakeholders.
Future Implications and Conclusion
The future of product quality improvement is heavily reliant on advanced statistical techniques like multivariate analysis.
As industries adopt newer technologies and data sources continue to grow, the ability to understand complex interdependencies will become even more crucial.
Multivariate analysis offers a roadmap to harnessing these capabilities, leading to superior product quality, efficient processes, and satisfied customers.
Organizations that prioritize multivariate analysis within their statistical technology departments are better positioned to enhance product quality and remain competitive in a fast-evolving market landscape.
資料ダウンロード
QCD調達購買管理クラウド「newji」は、調達購買部門で必要なQCD管理全てを備えた、現場特化型兼クラウド型の今世紀最高の購買管理システムとなります。
ユーザー登録
調達購買業務の効率化だけでなく、システムを導入することで、コスト削減や製品・資材のステータス可視化のほか、属人化していた購買情報の共有化による内部不正防止や統制にも役立ちます。
NEWJI DX
製造業に特化したデジタルトランスフォーメーション(DX)の実現を目指す請負開発型のコンサルティングサービスです。AI、iPaaS、および先端の技術を駆使して、製造プロセスの効率化、業務効率化、チームワーク強化、コスト削減、品質向上を実現します。このサービスは、製造業の課題を深く理解し、それに対する最適なデジタルソリューションを提供することで、企業が持続的な成長とイノベーションを達成できるようサポートします。
オンライン講座
製造業、主に購買・調達部門にお勤めの方々に向けた情報を配信しております。
新任の方やベテランの方、管理職を対象とした幅広いコンテンツをご用意しております。
お問い合わせ
コストダウンが利益に直結する術だと理解していても、なかなか前に進めることができない状況。そんな時は、newjiのコストダウン自動化機能で大きく利益貢献しよう!
(Β版非公開)