- お役立ち記事
- Basic method of point cloud
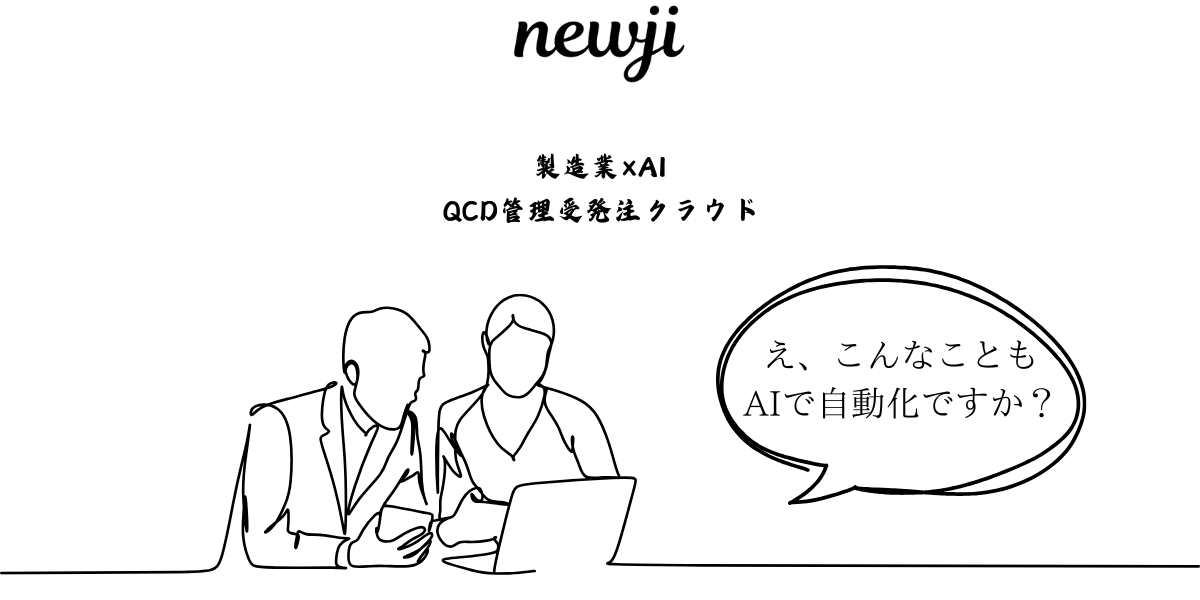
Basic method of point cloud
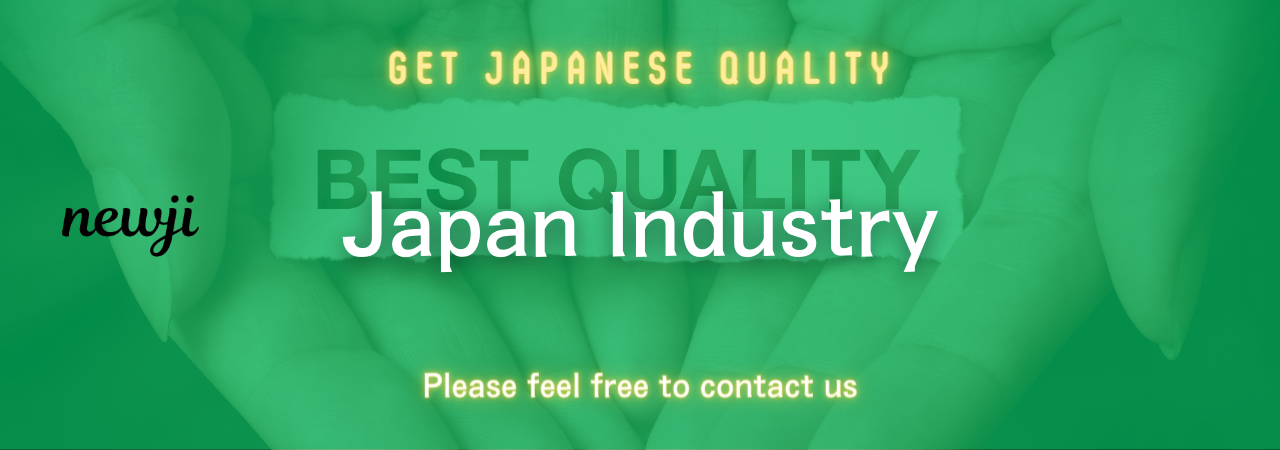
目次
Understanding Point Clouds
Point clouds are a collection of data points defined in a given space and are typically used to represent the external surface of objects.
These points can create detailed representations of real-world environments, which can be beneficial for a range of applications such as 3D modeling, mapping, and virtual reality.
Point clouds are often generated by 3D scanners, including laser scans and photogrammetry, but can also be produced from existing data sources.
How Point Clouds Work
Point clouds work by capturing the exact position of points in a 3D space, representing them in a coordinate system that can be analyzed and manipulated digitally.
Each point within the cloud carries specific coordinates – typically described as X, Y, and Z values – which determine its precise location.
This data can then be transformed into a mesh or a solid model, enabling the representation of physical forms digitally.
The density and accuracy of point clouds can vary greatly depending on the technology and method used.
Higher density point clouds offer more detailed representations but require more processing power and storage space.
Applications of Point Clouds
Point clouds have a diverse array of applications due to their ability to provide highly accurate 3D representations.
In architecture and construction, point clouds are valuable for creating as-built models of structures, supporting restoration projects, and ensuring the accuracy of new constructions.
In the field of geography and environmental science, point clouds support topographic mapping and landscape analysis, allowing for the examination of terrain, vegetation, and surface features.
Point clouds are also essential in automotive and aerospace industries for quality control, reverse engineering, and design validation.
Moreover, point clouds enhance virtual reality, augmented reality, and gaming fields by providing realistic environments and experiences.
Creating Point Clouds
The creation of point clouds can occur through different techniques, each with its advantages and limitations.
Laser scanning, a common method, uses laser beams to measure the distance to a surface, capturing millions of points to form a point cloud.
This method provides high accuracy and speed, making it ideal for large-scale projects like cityscapes or complex structures.
Photogrammetry is another technique that uses photography to generate point clouds.
By taking multiple overlapping images of an area or object from different angles, software algorithms identify common points among the photos and calculate their positions.
Lidar sensors, widely used in autonomous vehicles, also produce point clouds by sending out light pulses and measuring the time it takes for them to return from hitting surfaces.
This method is effective in various conditions, including night and adverse weather.
Processing Point Clouds
Once a point cloud is created, the data often requires processing to make it usable.
The processing steps include cleaning, filtering, and converting the raw point cloud data into formats suitable for modeling and analysis.
Cleaning involves removing noise or unwanted data, which is common in scans where points may have been captured outside the desired area.
Filtering reduces the density of the point cloud by eliminating redundant or irrelevant points, optimizing the data for efficient processing.
From there, the processed point cloud can be converted into a mesh model through triangulation, connecting the dots to define surfaces accurately.
Mesh models facilitate more straightforward editing, measurement, and analysis than raw point clouds.
Challenges and Considerations
Despite their capabilities, point clouds present several challenges, notably the management of large data volumes and the need for specialized software and hardware for processing.
Ensuring data accuracy and addressing occlusions – gaps due to inaccessible areas during scanning – are ongoing issues in point cloud creation.
The need for expertise in processing and interpreting point cloud data is also vital, as precision in capturing and manipulating these points determines the quality of the output.
As point cloud technology advances, developments in machine learning and artificial intelligence promise to streamline data processing and enhance flexibility in using point clouds across different sectors.
The Future of Point Cloud Technology
The future of point cloud technology is promising, with significant developments anticipated to enhance accuracy, efficiency, and application scope.
Emerging innovations in sensors and data processing algorithms will likely make real-time point cloud generation and augmented reality applications more attainable.
Increased integration of point clouds with AI and machine learning will improve the automation and accuracy of analyzing complex data, impacting industries such as smart city planning, logistics, and healthcare.
Moreover, as technology progresses, the affordability and accessibility of point cloud-generating devices will continue to rise, expanding their use in small and medium-sized enterprises.
Overall, the foundational methods in point cloud technology are rapidly evolving, opening new possibilities for transforming how the world is measured, understood, and recreated digitally.
資料ダウンロード
QCD調達購買管理クラウド「newji」は、調達購買部門で必要なQCD管理全てを備えた、現場特化型兼クラウド型の今世紀最高の購買管理システムとなります。
ユーザー登録
調達購買業務の効率化だけでなく、システムを導入することで、コスト削減や製品・資材のステータス可視化のほか、属人化していた購買情報の共有化による内部不正防止や統制にも役立ちます。
NEWJI DX
製造業に特化したデジタルトランスフォーメーション(DX)の実現を目指す請負開発型のコンサルティングサービスです。AI、iPaaS、および先端の技術を駆使して、製造プロセスの効率化、業務効率化、チームワーク強化、コスト削減、品質向上を実現します。このサービスは、製造業の課題を深く理解し、それに対する最適なデジタルソリューションを提供することで、企業が持続的な成長とイノベーションを達成できるようサポートします。
オンライン講座
製造業、主に購買・調達部門にお勤めの方々に向けた情報を配信しております。
新任の方やベテランの方、管理職を対象とした幅広いコンテンツをご用意しております。
お問い合わせ
コストダウンが利益に直結する術だと理解していても、なかなか前に進めることができない状況。そんな時は、newjiのコストダウン自動化機能で大きく利益貢献しよう!
(Β版非公開)