- お役立ち記事
- Basics and applications of Bayesian statistics that new employees in the R&D department should learn
月間76,176名の
製造業ご担当者様が閲覧しています*
*2025年3月31日現在のGoogle Analyticsのデータより
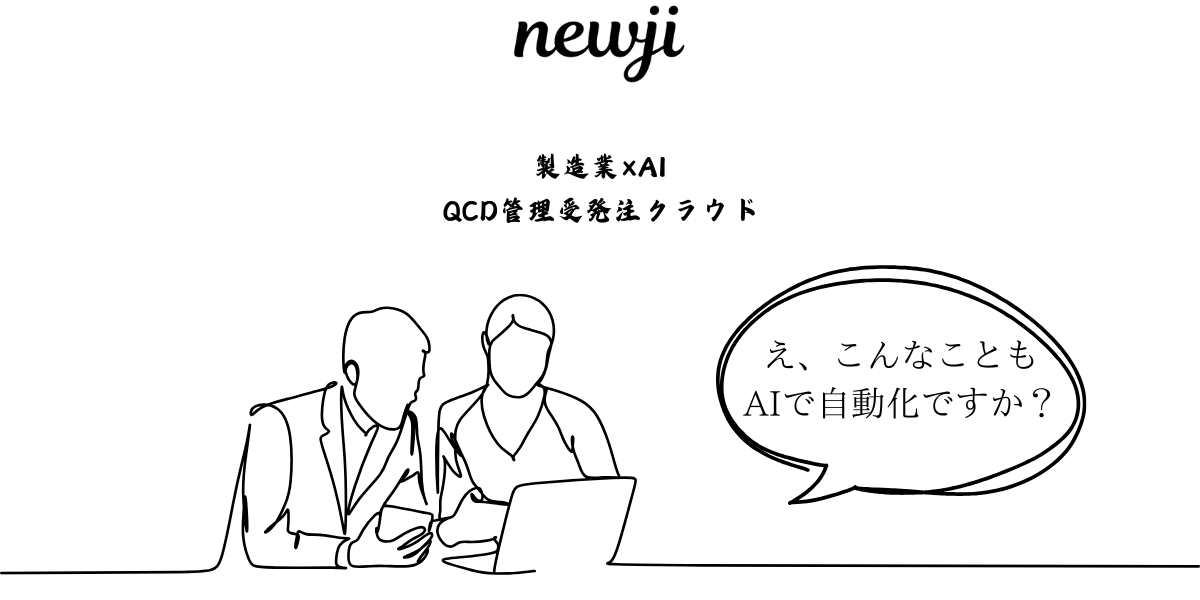
Basics and applications of Bayesian statistics that new employees in the R&D department should learn
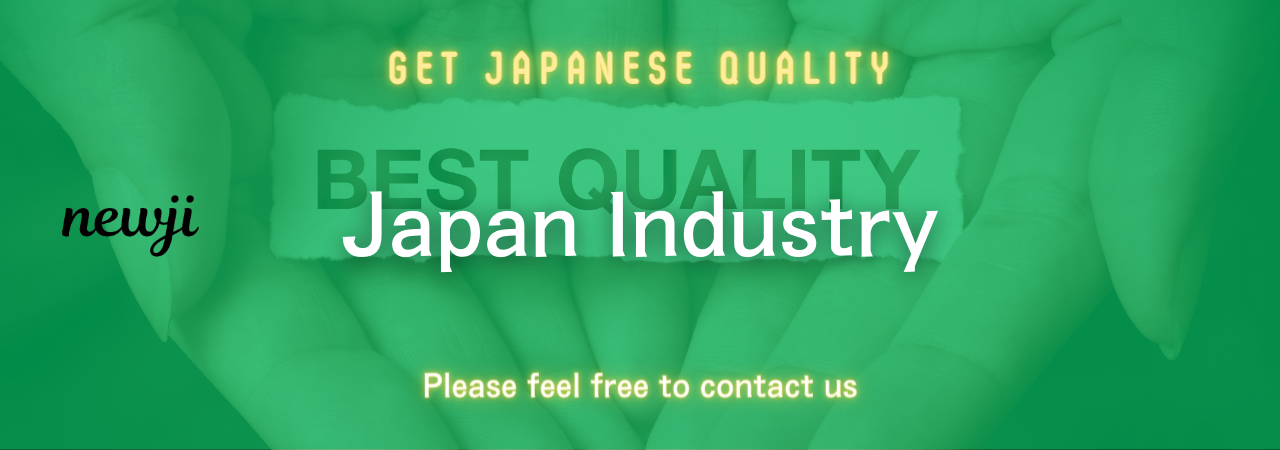
目次
Understanding Bayesian Statistics
Bayesian statistics is a branch of statistics that allows us to update the probability of a hypothesis as more evidence or information becomes available.
It’s named after Thomas Bayes, an 18th-century statistician and theologian, who introduced the concept of conditional probability.
This statistical method is fundamentally different from traditional frequentist statistics, offering a more flexible approach to data analysis.
Foundational Concepts
Before diving into applications, it’s vital to grasp the core concepts of Bayesian statistics.
First, we have the **prior probability**, which is our initial belief about a parameter before seeing the data.
Then, as new data comes in, we calculate the **likelihood**, which measures how well the data support this parameter.
Finally, through Bayes’ Theorem, we update our beliefs, leading us to the **posterior probability**.
The formula for Bayes’ Theorem is:
Posterior = (Likelihood * Prior) / Evidence
Where **Evidence** is the total probability of observing the data under all possible hypotheses.
Bayesian vs. Frequentist Approach
Bayesian statistics differ considerably from the frequentist approach.
While the frequentist model interprets probability as a long-term frequency in repeated experiments, the Bayesian perspective treats probability as a degree of belief.
For instance, when estimating a parameter, the frequentist might use confidence intervals, while the Bayesian would provide a probability distribution representing all possible values of the parameter.
Moreover, frequentists often assume fixed effects, but Bayesians account for variability and uncertainty.
This makes Bayesian methods particularly useful when dealing with complex models or limited data.
Applications in R&D
Bayesian statistics hold immense potential for research and development departments across various industries.
Here are some key areas where new employees in R&D can leverage Bayesian methods:
Enhancing Experiment Design
Bayesian statistics can improve experiment design by incorporating prior knowledge to calculate sample sizes more efficiently.
This results in more cost-effective experiments and ensures that resources are allocated wisely.
For instance, if a company is testing a new product, Bayesian methods can help determine the necessary sample size needed to achieve a desired level of confidence in the results.
Reliable Decision Making
In decision-making scenarios, Bayesian statistics offer a structured framework to evaluate uncertainties and predict outcomes.
By updating beliefs in light of new evidence, decision-makers can make informed choices, reducing the risk of costly mistakes.
This is particularly useful when data is scarce or incomplete, a common challenge in R&D settings.
Improving Predictive Models
Bayesian methods are renowned for their ability to create robust predictive models.
In R&D, these models can be applied to forecast market trends, anticipate product failures, or optimize manufacturing processes.
The flexible nature of Bayesian models allows researchers to incorporate expert opinions and historical data, enhancing the predictive power.
Practical Tips for New Employees
For R&D department employees new to Bayesian statistics, here are some practical tips to get started:
Gain a Solid Theoretical Understanding
Before diving into applications, ensure that you have a firm grasp of Bayesian theory.
Numerous online resources, courses, and textbooks can provide valuable insights into foundational concepts.
Start with Simple Models
Begin exploring Bayesian methods with simple models to build confidence and understanding.
Tools such as Excel or online Bayesian calculators can help visualize simple Bayesian updates without complex coding.
Utilize Bayesian Software
There are sophisticated software packages available that simplify Bayesian analysis, including R, Python’s PyMC3, and Stan.
These tools can handle complex models and large datasets, making them indispensable for R&D projects.
Familiarize yourself with one or more of these tools as you progress.
Understand Assumptions and Limitations
Like any statistical method, Bayesian statistics come with assumptions and limitations.
Understand these to avoid misinterpretations.
Bayesian methods rely on prior distributions, which, if poorly chosen, can skew results.
Thus, it’s crucial to make informed choices for prior probabilities based on sound knowledge and expertise.
Collaborate and Communicate
Collaborate with your team and communicate findings effectively.
Bayesian results often involve probabilistic interpretations, so clear communication is essential for non-statistician stakeholders to understand the implications.
Conclusion
For new employees in the R&D department, mastering Bayesian statistics offers numerous benefits, from enhancing experimental design to improving predictive models and making informed decision-making.
By embracing Bayesian methods, you can bring a quantitative edge to your research endeavors, ultimately driving innovative solutions and advancements in your field.
Stay curious, keep learning, and leverage the potential of Bayesian statistics to contribute effectively to your organization’s goals.
資料ダウンロード
QCD管理受発注クラウド「newji」は、受発注部門で必要なQCD管理全てを備えた、現場特化型兼クラウド型の今世紀最高の受発注管理システムとなります。
ユーザー登録
受発注業務の効率化だけでなく、システムを導入することで、コスト削減や製品・資材のステータス可視化のほか、属人化していた受発注情報の共有化による内部不正防止や統制にも役立ちます。
NEWJI DX
製造業に特化したデジタルトランスフォーメーション(DX)の実現を目指す請負開発型のコンサルティングサービスです。AI、iPaaS、および先端の技術を駆使して、製造プロセスの効率化、業務効率化、チームワーク強化、コスト削減、品質向上を実現します。このサービスは、製造業の課題を深く理解し、それに対する最適なデジタルソリューションを提供することで、企業が持続的な成長とイノベーションを達成できるようサポートします。
製造業ニュース解説
製造業、主に購買・調達部門にお勤めの方々に向けた情報を配信しております。
新任の方やベテランの方、管理職を対象とした幅広いコンテンツをご用意しております。
お問い合わせ
コストダウンが利益に直結する術だと理解していても、なかなか前に進めることができない状況。そんな時は、newjiのコストダウン自動化機能で大きく利益貢献しよう!
(β版非公開)