- お役立ち記事
- Basics and applications of compressive sensing technology and the latest technology
月間76,176名の
製造業ご担当者様が閲覧しています*
*2025年3月31日現在のGoogle Analyticsのデータより
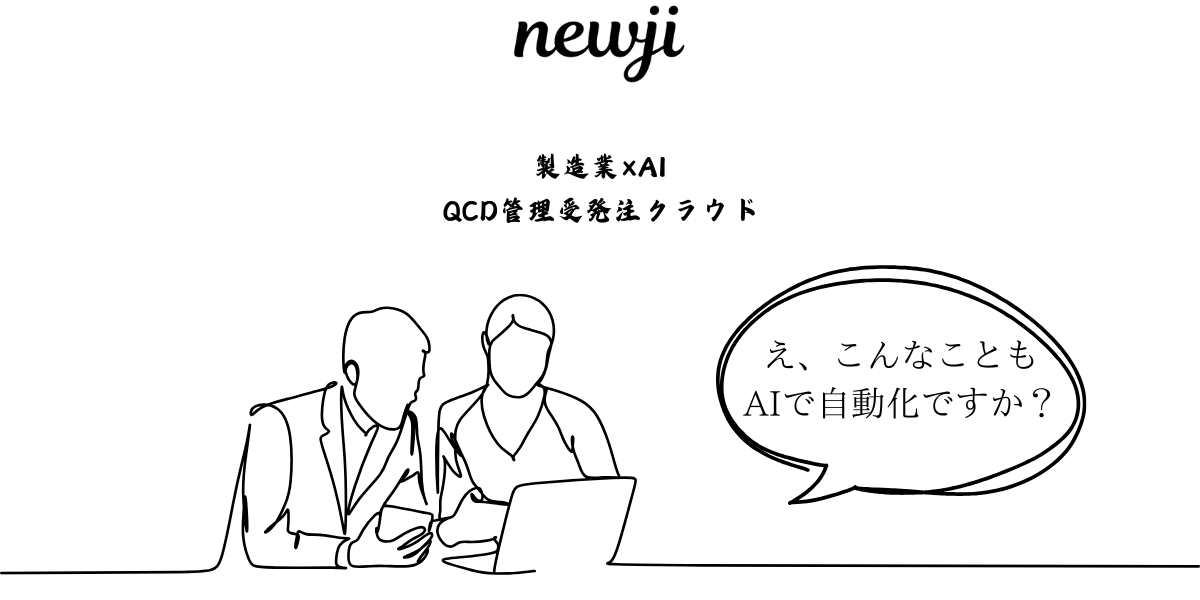
Basics and applications of compressive sensing technology and the latest technology
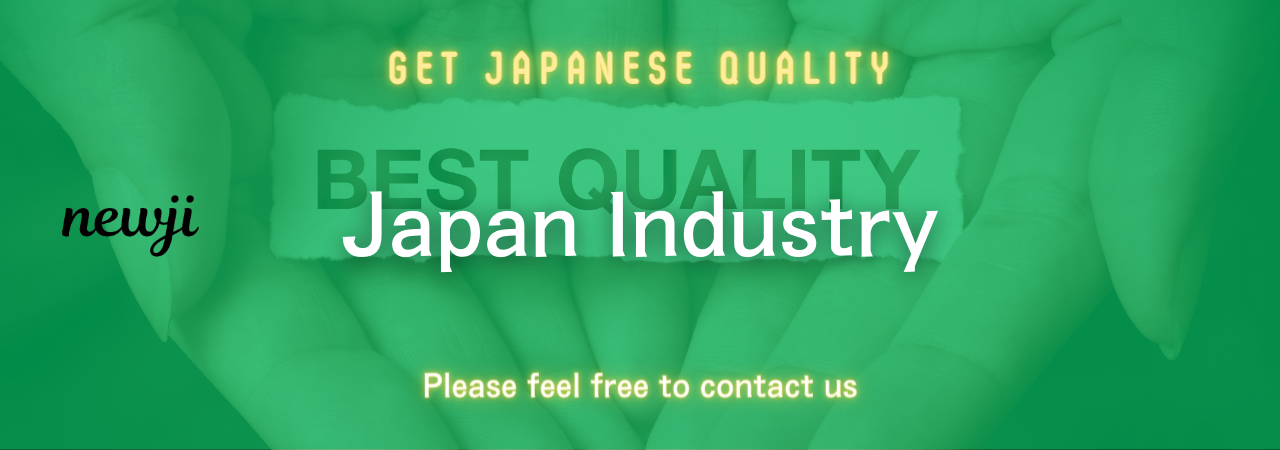
目次
Understanding Compressive Sensing Technology
Compressive sensing is a revolutionary concept in signal processing and data acquisition that allows for the reconstruction of a signal using fewer samples than traditionally required by the Nyquist-Shannon sampling theorem.
This groundbreaking approach has garnered significant attention due to its ability to efficiently acquire and process vast amounts of data, making it applicable to a wide range of fields.
The core principle of compressive sensing is that many natural signals, in certain domains, are sparse or compressible.
This means that most of the information contained in the signal can be captured with a relatively small number of non-zero coefficients when transformed into an appropriate basis or domain.
Using this sparsity, compressive sensing algorithms efficiently reconstruct the original signal from a reduced number of measurements, effectively compressing the data during acquisition rather than after the data has already been collected.
Basic Concepts of Compressive Sensing
Compressive sensing relies on two key concepts: sparsity and incoherence.
A signal is sparse if it has only a few non-zero coefficients in a particular domain.
For example, an audio signal might be sparse in the frequency domain, while an image could be sparse in terms of edges or textures.
Incoherence refers to the way signals are sampled.
In compressive sensing, the sampling system encodes information incoherently, ensuring that the acquisition process captures the essential features of the signal while minimizing redundancy.
This is typically achieved by using random or pseudo-random measurement patterns, which serve to spread out the information across the entire set of samples.
Central to compressive sensing is the reconstruction process.
Algorithms such as basis pursuit, orthogonal matching pursuit, and iterative shrinkage-thresholding are employed to recover the original signal from the compressed measurements.
These algorithms aim to solve an optimization problem that finds the sparsest solution of a system of linear equations, constrained by the measured data.
Applications of Compressive Sensing
The potential applications of compressive sensing are vast and varied, spanning from medical imaging to wireless communications and beyond.
Medical Imaging
In the field of medical imaging, compressive sensing has the potential to dramatically reduce the acquisition time and radiation exposure for patients.
For instance, in Magnetic Resonance Imaging (MRI), compressive sensing can speed up the image acquisition process by collecting fewer data points without compromising the quality of the images.
This is particularly beneficial for dynamic imaging, where the physical pace of data acquisition is often a bottleneck.
Wireless Communications
In wireless communications, compressive sensing enables efficient data transmission by reducing the quantity of data that needs to be sent or stored.
For sensor networks that monitor environmental parameters over wide geographical areas, compressive sensing can significantly decrease the energy consumption by reducing the need for data transmission.
Image and Video Compression
Compressive sensing also shows promise in image and video processing.
Traditional methods of compression, such as JPEG or MPEG, apply complex algorithms after the data is fully acquired.
In contrast, compressive sensing allows for image or video data to be compressed during acquisition, leading to significant savings in storage and transmission resources.
The Latest Technology in Compressive Sensing
Recent advancements in compressive sensing technology have focused on improving the robustness and accuracy of signal reconstruction, as well as expanding its applicability to more challenging scenarios.
Deep Learning Integration
One of the most exciting developments is the integration of deep learning with compressive sensing techniques.
The use of deep neural networks has shown promise in enhancing the performance of traditional compressive sensing algorithms, particularly in terms of robustness against noise and artifacts.
These networks can learn from vast amounts of data to create powerful priors for signal reconstruction, allowing for more accurate and faster results.
Hardware Innovations
Innovations in sensor hardware have also contributed to the advancement of compressive sensing.
For instance, new sensor designs enable direct capture of compressed measurements without the need for extensive post-processing, making real-time applications more feasible.
Quantum Compressive Sensing
Another cutting-edge development is the application of compressive sensing principles within the realm of quantum computing and quantum information processing.
Quantum compressive sensing exploits the principles of quantum mechanics to further enhance data acquisition and signal processing capabilities, opening new avenues in secure communications and quantum imaging.
Challenges and Future Directions
While compressive sensing offers numerous advantages, it is not without its challenges.
One of the primary obstacles is the need for efficient algorithms that can handle the computational complexity of reconstructing signals from non-traditional measurements.
Moreover, the theoretical guarantees for signal recovery often assume ideal conditions that may not hold in practical scenarios due to noise or other real-world factors.
Nevertheless, research and development in this area continue to advance, with promising results.
Future directions include improving algorithm efficiency, expanding the application of compressive sensing to more types of data, and enhancing its robustness under adverse conditions.
In conclusion, compressive sensing represents a transformative approach to data acquisition and signal processing, offering vast improvements in efficiency and capability across numerous industries.
With ongoing advancements and a growing understanding of its potential, compressive sensing is poised to make significant impacts on technologies and applications ranging from medical diagnostics to telecommunications and beyond.
資料ダウンロード
QCD管理受発注クラウド「newji」は、受発注部門で必要なQCD管理全てを備えた、現場特化型兼クラウド型の今世紀最高の受発注管理システムとなります。
ユーザー登録
受発注業務の効率化だけでなく、システムを導入することで、コスト削減や製品・資材のステータス可視化のほか、属人化していた受発注情報の共有化による内部不正防止や統制にも役立ちます。
NEWJI DX
製造業に特化したデジタルトランスフォーメーション(DX)の実現を目指す請負開発型のコンサルティングサービスです。AI、iPaaS、および先端の技術を駆使して、製造プロセスの効率化、業務効率化、チームワーク強化、コスト削減、品質向上を実現します。このサービスは、製造業の課題を深く理解し、それに対する最適なデジタルソリューションを提供することで、企業が持続的な成長とイノベーションを達成できるようサポートします。
製造業ニュース解説
製造業、主に購買・調達部門にお勤めの方々に向けた情報を配信しております。
新任の方やベテランの方、管理職を対象とした幅広いコンテンツをご用意しております。
お問い合わせ
コストダウンが利益に直結する術だと理解していても、なかなか前に進めることができない状況。そんな時は、newjiのコストダウン自動化機能で大きく利益貢献しよう!
(β版非公開)