- お役立ち記事
- Basics and latest trends of anomaly detection technology using sensing technology
月間76,176名の
製造業ご担当者様が閲覧しています*
*2025年3月31日現在のGoogle Analyticsのデータより
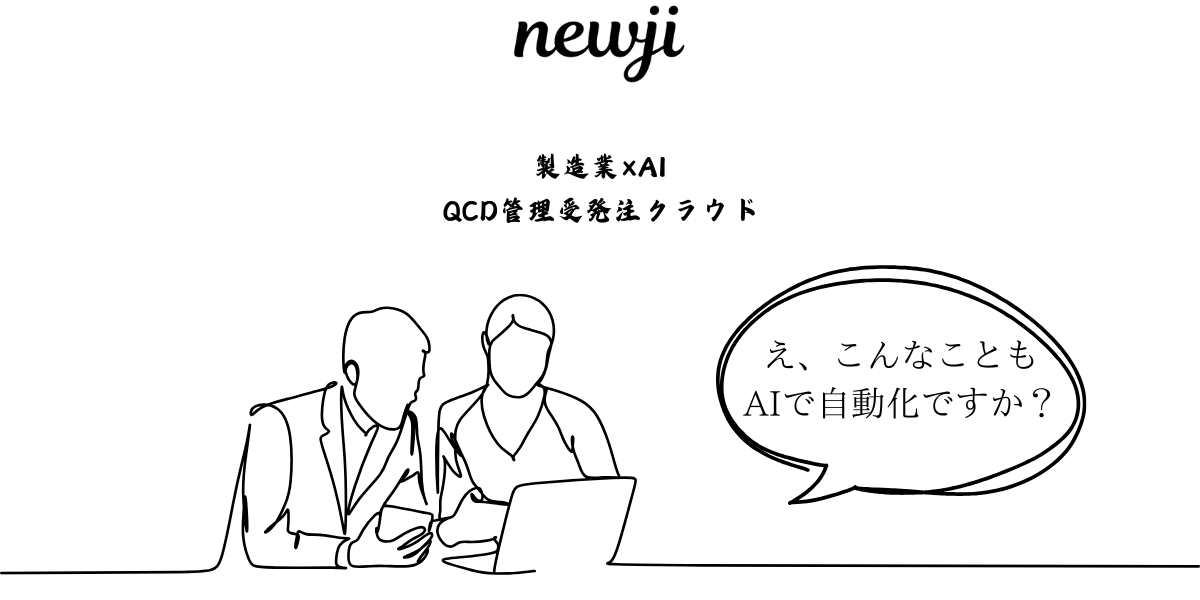
Basics and latest trends of anomaly detection technology using sensing technology
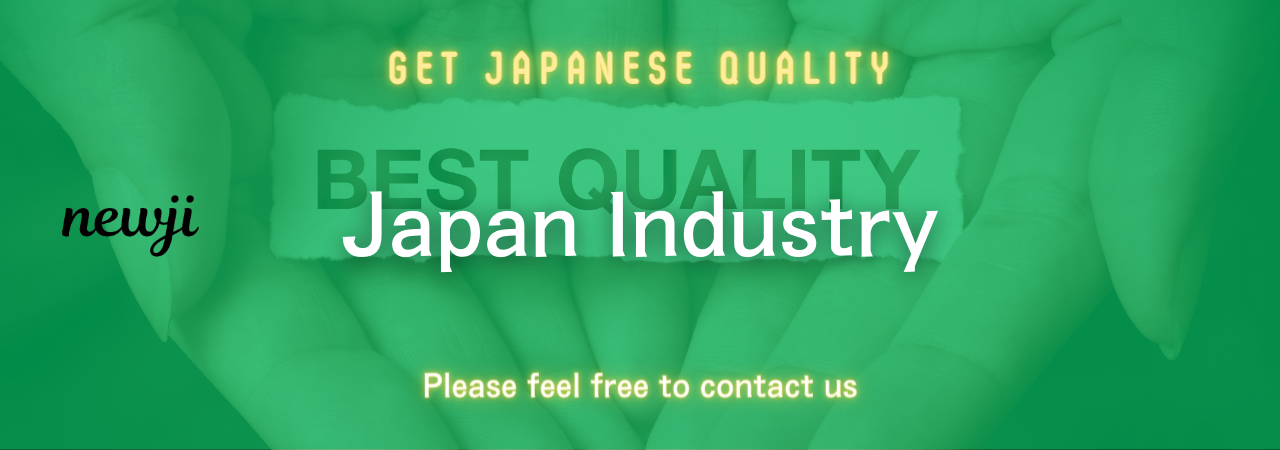
目次
Understanding Anomaly Detection
Anomaly detection is a technique used in various industries to identify unusual patterns or activities that deviate from the norm.
This process is crucial in fields such as finance, healthcare, cybersecurity, and manufacturing.
By detecting anomalies early, organizations can prevent potential issues, fraud, or system failures, ensuring a smooth operation across various sectors.
What is Sensing Technology?
Sensing technology plays a pivotal role in modern anomaly detection.
It involves the use of sensors to capture data from different environments or systems.
This data, when analyzed, helps in identifying unusual patterns or behavior.
Common types of sensors include temperature sensors, motion detectors, and pressure sensors, among others.
The data collected from these sensors is critical in monitoring systems, providing real-time insights that are vital for anomaly detection.
Types of Anomaly Detection
There are primarily three types of anomalies: point anomalies, contextual anomalies, and collective anomalies.
Understanding these can help in applying the correct techniques for detection.
Point Anomalies
Point anomalies are data points that deviate significantly from the rest of the dataset.
They are isolated instances that are rare compared to normal behavior.
For example, a sudden spike in temperature recorded by a sensor may indicate a point anomaly.
Contextual Anomalies
Contextual anomalies occur when the deviation is context-sensitive.
It usually depends on surrounding data points or the context in which they occur.
For instance, a sudden drop in stock prices might be a contextual anomaly if it occurs after a major geopolitical event.
Collective Anomalies
Collective anomalies involve a group of related data points that together deviate from the expected pattern.
These types of anomalies are often seen in network traffic, where a group of several unusual packets might indicate an unauthorized access attempt.
Methods of Anomaly Detection
Several techniques are used to detect anomalies, each with its advantages and limitations.
Statistical Methods
Statistical methods involve using statistical tests or models to identify anomalies.
These techniques assume that the data follows a specific distribution and identify any deviation from this distribution as an anomaly.
Common statistical methods include the Z-score, Grubbs’ test, and the Chi-square test.
Machine Learning Methods
Machine learning methods are gaining popularity due to their ability to handle large volumes of data and learn patterns over time.
These methods include supervised, unsupervised, and semi-supervised learning.
– **Supervised Learning:** This involves training a model using labeled data, where anomalies are already identified.
The model learns to recognize patterns and classify future data as normal or anomalous.
– **Unsupervised Learning:** Unsupervised learning doesn’t rely on labeled data.
It identifies anomalies based on patterns it recognizes in the data, making it suitable for scenarios where anomalies are not pre-labeled.
– **Semi-supervised Learning:** This method uses a small amount of labeled data to identify patterns and detect anomalies in a larger unlabeled dataset.
It’s a combination of the other two methods, leveraging their strengths.
Neural Networks
Neural networks, especially deep learning models, are increasingly being used for anomaly detection.
They can model complex patterns and recognize subtle deviations that traditional methods might miss.
Autoencoders, a type of neural network, are particularly useful.
They compress input data and attempt to reproduce it, flagging any failures in reproduction as potential anomalies.
Applications of Anomaly Detection with Sensing Technology
Anomaly detection using sensing technology has a wide array of applications across different industries.
Healthcare
In healthcare, anomaly detection helps in monitoring patients’ vital signs for any irregularities.
Sensing technology like wearable health devices can provide continuous data, alerting healthcare providers to potential medical emergencies.
Finance
In the financial sector, anomaly detection is used to identify fraudulent transactions by analyzing transaction patterns.
This helps in preventing financial losses and safeguarding consumer data.
Manufacturing
Manufacturing industries use sensing technology to monitor machinery and equipment.
Detecting anomalies early can prevent equipment breakdowns, reduce downtime, and improve operational efficiency.
Cybersecurity
Anomaly detection is crucial in cybersecurity to identify unauthorized access or breaches.
By analyzing network traffic and system logs, organizations can detect and respond to potential threats swiftly.
Latest Trends in Anomaly Detection
The field of anomaly detection is continually evolving, with several emerging trends shaping its future.
Integration of AI and IoT
The integration of Artificial Intelligence (AI) with the Internet of Things (IoT) is enhancing anomaly detection capabilities.
Smart sensors and devices collect vast amounts of data, which AI algorithms can analyze in real time for anomalies, improving accuracy and response times.
Edge Computing
Edge computing involves processing data closer to the source, such as sensors or devices, rather than relying on centralized data centers.
This approach reduces latency and allows for faster anomaly detection and response, making it ideal for time-sensitive applications.
Cloud-Based Solutions
Cloud computing offers scalable solutions for anomaly detection.
With the ability to handle large datasets and complex computations, cloud-based platforms provide the infrastructure needed for advanced anomaly detection techniques.
Enhanced Predictive Maintenance
Anomaly detection is driving advancements in predictive maintenance.
By predicting equipment failures or maintenance needs before they occur, businesses can save on costs and improve operational efficiency.
Conclusion
Anomaly detection using sensing technology is pivotal across multiple industries.
As technology evolves, so do the methods and applications of anomaly detection.
From machine learning and AI integration to edge computing and cloud solutions, the field continues to make strides, offering innovative ways to enhance operational efficiency and security.
These advancements not only help in early problem detection but also pave the way for smarter, more intuitive systems that adapt to the needs of various sectors.
資料ダウンロード
QCD管理受発注クラウド「newji」は、受発注部門で必要なQCD管理全てを備えた、現場特化型兼クラウド型の今世紀最高の受発注管理システムとなります。
ユーザー登録
受発注業務の効率化だけでなく、システムを導入することで、コスト削減や製品・資材のステータス可視化のほか、属人化していた受発注情報の共有化による内部不正防止や統制にも役立ちます。
NEWJI DX
製造業に特化したデジタルトランスフォーメーション(DX)の実現を目指す請負開発型のコンサルティングサービスです。AI、iPaaS、および先端の技術を駆使して、製造プロセスの効率化、業務効率化、チームワーク強化、コスト削減、品質向上を実現します。このサービスは、製造業の課題を深く理解し、それに対する最適なデジタルソリューションを提供することで、企業が持続的な成長とイノベーションを達成できるようサポートします。
製造業ニュース解説
製造業、主に購買・調達部門にお勤めの方々に向けた情報を配信しております。
新任の方やベテランの方、管理職を対象とした幅広いコンテンツをご用意しております。
お問い合わせ
コストダウンが利益に直結する術だと理解していても、なかなか前に進めることができない状況。そんな時は、newjiのコストダウン自動化機能で大きく利益貢献しよう!
(β版非公開)