- お役立ち記事
- Basics and practical points of sparse modeling
月間77,185名の
製造業ご担当者様が閲覧しています*
*2025年2月28日現在のGoogle Analyticsのデータより
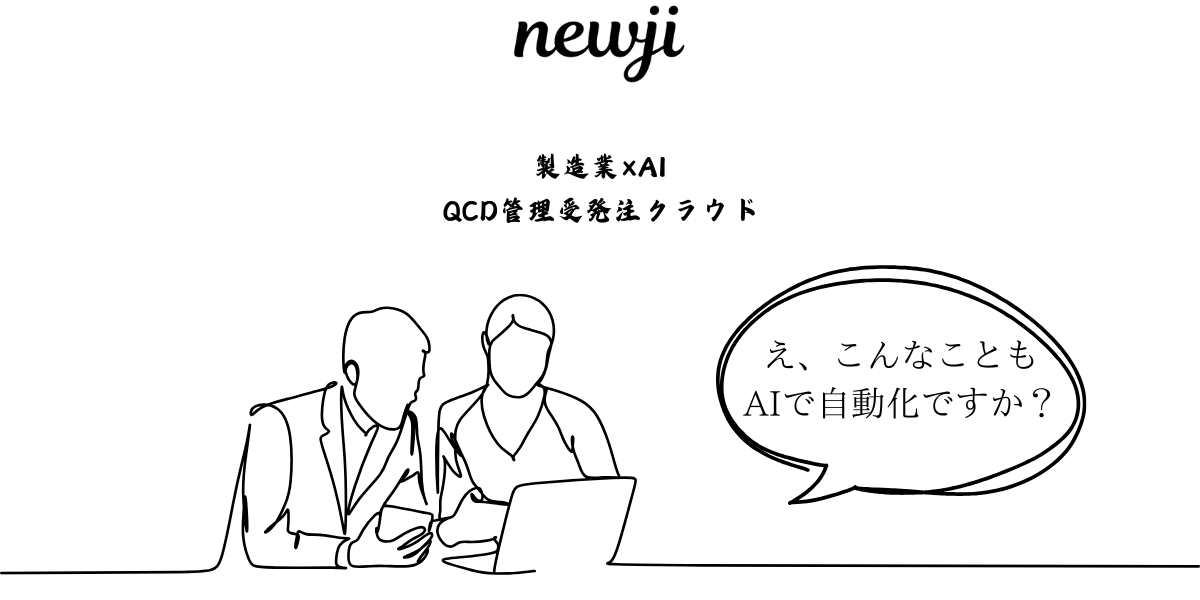
Basics and practical points of sparse modeling
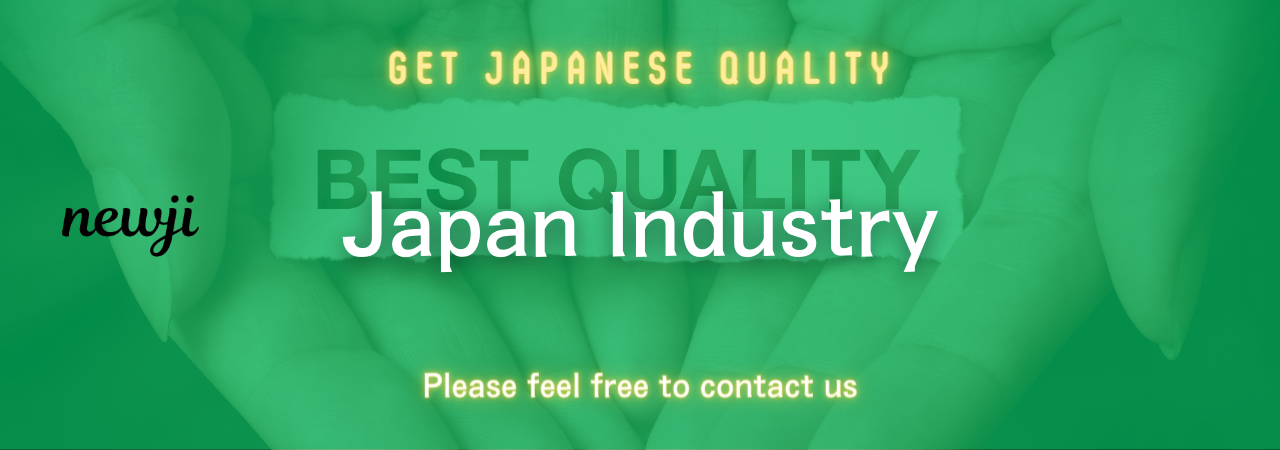
目次
Understanding Sparse Modeling
Sparse modeling is an intriguing concept within the realm of data science and machine learning.
At its core, sparse modeling is about finding a way to represent data using fewer variables than originally present.
This approach is useful in dealing with large datasets, where many of the variables may not contribute significantly to the predictive power of a model.
Sparse modeling is especially important for simplifying complex models, reducing overfitting, and improving interpretation.
By focusing on significant variables, one can create more efficient models that are both easier to understand and faster to compute.
Let’s delve into the fundamentals of sparse modeling and explore some practical applications.
Why Use Sparse Modeling?
Sparse modeling is beneficial for several reasons.
First, it helps enhance model accuracy by eliminating redundant or irrelevant features.
Removing unnecessary features can streamline the data, making it easier for algorithms to uncover meaningful patterns.
Second, sparse models often result in faster computation times, saving resources and time, especially with massive datasets.
By reducing the number of variables, computations demand less memory and processing power.
Lastly, sparse models offer greater interpretability.
With fewer variables, it becomes simpler to understand the contributions of each feature, which is crucial in fields where interpretability is paramount, such as healthcare and finance.
Techniques for Sparse Modeling
There are various techniques employed in sparse modeling, each leveraging different mathematical foundations.
Some of the most common include:
Lasso Regression
Lasso Regression, or Least Absolute Shrinkage and Selection Operator, is a linear modeling method that uses L1 regularization.
It shrinks the coefficients of less significant variables to zero, effectively performing variable selection.
Lasso is particularly useful when one needs a simpler model without sacrificing performance.
Ridge Regression
Ridge Regression uses L2 regularization, adding a penalty equal to the square of the magnitude of coefficients.
While it doesn’t inherently produce a sparse model in terms of variable count, it does shrink the coefficients, giving preference to models with smaller coefficients while maintaining most variables.
Elastic Net
Elastic Net combines L1 and L2 penalties, offering the benefits of both Lasso and Ridge Regression.
It is particularly effective when working with correlated features, where Lasso tends to randomly select one variable from a set of equally predictive variables.
Elastic Net encourages group selection of correlated variables, maintaining model stability.
Principal Component Analysis (PCA)
Though traditionally not a sparse method, PCA helps in data dimensionality reduction by transforming the original data into a set of linearly uncorrelated components.
Sparse PCA incorporates an additional step to ensure that the components themselves are sparse, increasing interpretability.
Practical Applications of Sparse Modeling
Sparse modeling is not just a theoretical exercise; it has numerous practical applications across various domains.
Genomics
In genomics, datasets are expansive, with thousands of genetic markers.
Sparse modeling helps in identifying the most relevant genes linked to diseases, enabling researchers to focus on a smaller subset of markers that are most likely to yield actionable insights.
Text Analysis
Text data often contain a significant volume of terms that are not useful for modeling purposes.
Sparse modeling techniques can identify the most relevant words or phrases for tasks such as sentiment analysis or topic detection, making models more efficient and accurate.
Image Processing
For image recognition, pixel number is immense, but not all pixels carry important information.
Sparse models reduce the complexity by concentrating on key components of images, which results in quicker and more efficient processing without a severe drop in accuracy.
Challenges and Considerations
While sparse modeling offers numerous advantages, it is not without challenges.
One of the primary considerations is selecting the right level of sparsity, which involves a trade-off between the simplicity of the model and its predictive power.
Over-simplification can lead to the loss of important information, while under-simplification may not fully leverage the benefits of sparsity.
Thus, fine-tuning model parameters, such as selecting the appropriate regularization strength, is crucial.
Furthermore, some sparse modeling techniques, such as Lasso, may exhibit instability or biased estimates when dealing with datasets with high multicollinearity.
In such cases, combining methods like Elastic Net might provide a more robust solution.
Conclusion
Sparse modeling serves as a powerful tool in data science, providing a pathway to more efficient, interpretable models.
By reducing data dimensionality and focusing on essential variables, sparse models enhance computational efficiency, mitigate overfitting, and improve the overall understanding of a model’s mechanics.
With applications spanning various fields like genomics, text analysis, and image processing, the impact of sparse modeling is profound.
Future advancements and innovations in this area will likely continue to enhance the way we process and interpret large, complex datasets.
By continually refining techniques and methodologies, sparse modeling will remain a vital component in the toolkit of data scientists and machine learning practitioners.
資料ダウンロード
QCD管理受発注クラウド「newji」は、受発注部門で必要なQCD管理全てを備えた、現場特化型兼クラウド型の今世紀最高の受発注管理システムとなります。
ユーザー登録
受発注業務の効率化だけでなく、システムを導入することで、コスト削減や製品・資材のステータス可視化のほか、属人化していた受発注情報の共有化による内部不正防止や統制にも役立ちます。
NEWJI DX
製造業に特化したデジタルトランスフォーメーション(DX)の実現を目指す請負開発型のコンサルティングサービスです。AI、iPaaS、および先端の技術を駆使して、製造プロセスの効率化、業務効率化、チームワーク強化、コスト削減、品質向上を実現します。このサービスは、製造業の課題を深く理解し、それに対する最適なデジタルソリューションを提供することで、企業が持続的な成長とイノベーションを達成できるようサポートします。
製造業ニュース解説
製造業、主に購買・調達部門にお勤めの方々に向けた情報を配信しております。
新任の方やベテランの方、管理職を対象とした幅広いコンテンツをご用意しております。
お問い合わせ
コストダウンが利益に直結する術だと理解していても、なかなか前に進めることができない状況。そんな時は、newjiのコストダウン自動化機能で大きく利益貢献しよう!
(β版非公開)