- お役立ち記事
- Basics of 3D point cloud processing technology and applications of deep learning
月間76,176名の
製造業ご担当者様が閲覧しています*
*2025年3月31日現在のGoogle Analyticsのデータより
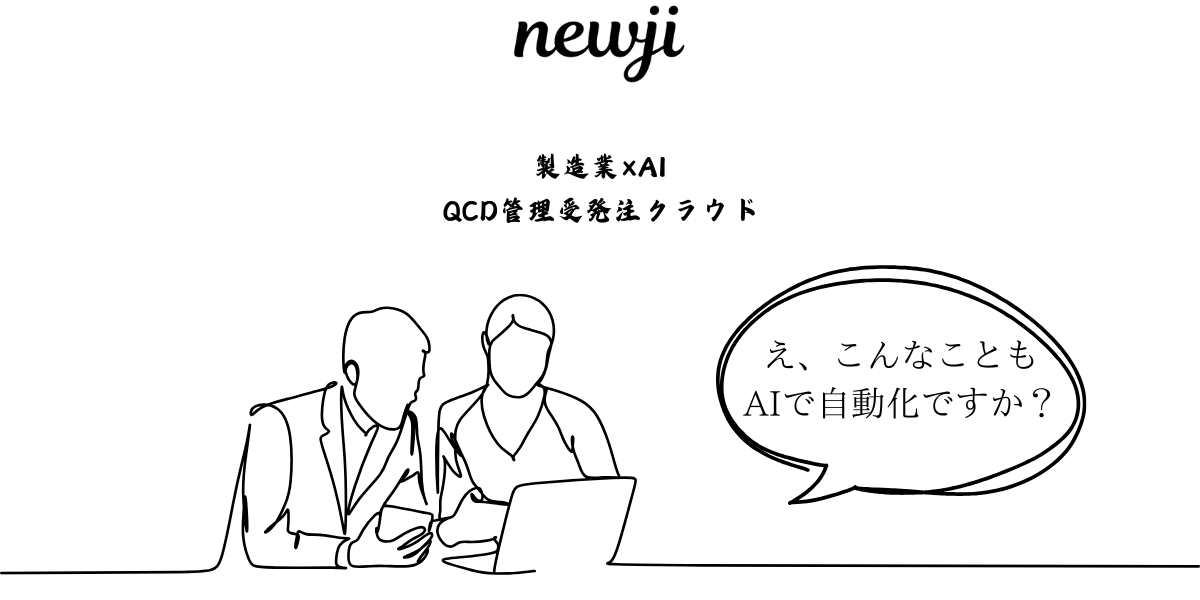
Basics of 3D point cloud processing technology and applications of deep learning
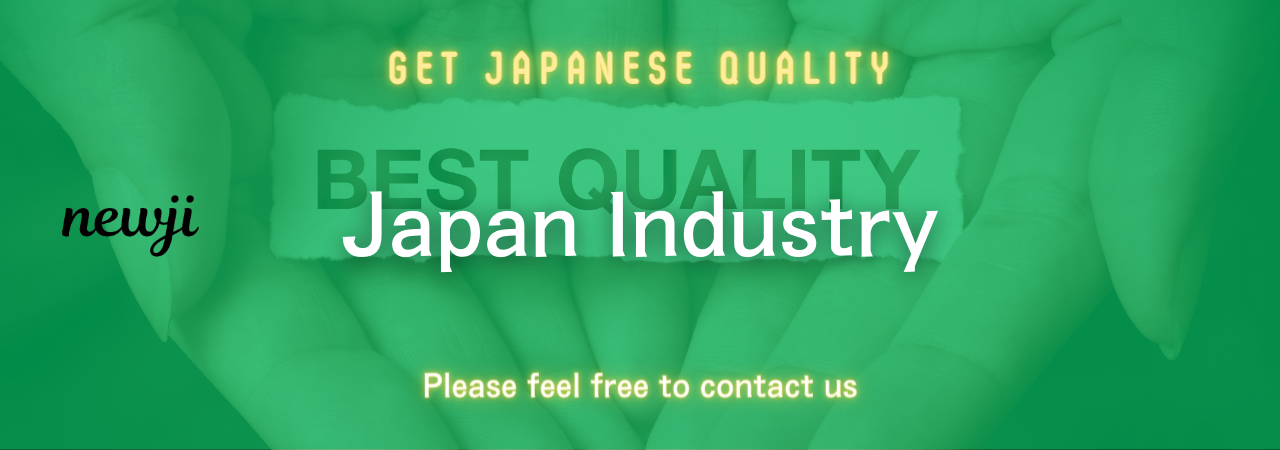
目次
Introduction to 3D Point Cloud Processing
3D point cloud processing technology has been at the forefront of various modern applications due to its ability to capture real-world scenes in a digital format.
This technology involves collecting a set of data points in space, which represent the external surface of an object or environment.
Typically generated by LiDAR sensors, photogrammetry, or structured light methods, these data sets offer detailed, high-precision information.
The rising interest in 3D point clouds can be attributed to their detailed representation of objects, which is invaluable in fields like archaeology, architecture, and urban planning.
As this technology progresses, it’s pivotal to explore the basics of processing these point clouds and understand how deep learning plays a significant role in advancing their applications.
The Basics of Point Cloud Processing
Point cloud processing starts with the acquisition of data, usually through various scanning technologies.
Once the data points are collected, they need to be preprocessed to remove noise and irrelevant details.
This step is crucial as raw data often contain inaccuracies due to environmental factors or sensor limitations.
After preprocessing, segmentation becomes necessary to identify and classify different parts of the object or scene.
Segmentation involves grouping data points into subsets that represent distinct components within the cloud.
For precise analysis, it’s essential to align and register these segments accurately, providing a coherent structure that mirrors the object or environment.
Finally, the processed point clouds can be used for reconstruction to build detailed 3D models.
This is the stage where visualization techniques render these models into a format suitable for various applications, including simulations, animations, or virtual reality experiences.
Deep Learning in Point Cloud Processing
Deep learning has revolutionized point cloud processing by enhancing the accuracy and efficiency of analyzing and interpreting these data sets.
With neural networks capable of learning intricate patterns, deep learning can streamline the segmentation, classification, and semantic understanding of point clouds.
Deep Learning Models for Point Clouds
A variety of deep learning models are specifically designed for processing point clouds.
PointNet and its variants, such as PointNet++ and DGCNN, are popular architectures that have demonstrated remarkable proficiency in handling raw point cloud data.
These models deal directly with the spatial relationships and properties inherent in point clouds, making them particularly suitable for 3D data interpretation.
By leveraging these models, deep learning enhances the capability to recognize complex patterns and features within the data.
This ability not only improves accuracy but also enables automated processes that were traditionally labor-intensive.
Key Applications of Deep Learning in Point Clouds
The integration of deep learning with point cloud processing facilitates numerous applications across various industry sectors.
Some of the prominent applications include:
1. **Autonomous Vehicles**: Deep learning aids in object detection, tracking, and classification, essential for autonomous navigation systems.
Point clouds provide comprehensive spatial awareness to ensure safe and efficient vehicular movements.
2. **Building Information Modeling (BIM)**: In the construction and architecture sectors, detailed 3D models derived from point clouds assist in designing, analyzing, and managing construction projects.
Deep learning enhances the precision in segmenting and interpreting complex building structures.
3. **Robotics**: Point cloud processing empowers robots with enhanced perception capabilities, enabling them to interact more effectively with their surroundings.
Deep learning improves a robot’s ability to understand and predict environmental changes.
4. **Healthcare**: In medical imaging and surgeries, 3D point cloud data from scans offer surgeons a detailed view of anatomical structures.
Deep learning aids in identifying patterns and anomalies, thus improving diagnostic accuracy.
Challenges and Future Perspectives
While point cloud processing has advanced significantly, challenges remain that researchers and developers must address.
Data handling and storage remain a concern due to the sheer size and complexity of 3D datasets.
Furthermore, developing standardized approaches for noise reduction and segmentation can be challenging due to the diverse nature of point clouds.
Despite these challenges, the future of point cloud processing looks promising with ongoing advancements in deep learning.
Innovations in hardware, such as improved sensors and computational tools, will further enhance the collection and processing of point clouds.
The evolution of deep learning algorithms will continue to push the boundaries of how we analyze and utilize 3D data.
Conclusion
The synergy between 3D point cloud processing technology and deep learning is driving significant innovations across numerous fields.
By understanding the basics of point cloud processing and recognizing the pivotal role of deep learning, we can unlock new potentials in technology applications.
As research and technology advance, point clouds will become an integral part of the digital landscape, offering deeper insights and smarter solutions to complex real-world challenges.
資料ダウンロード
QCD管理受発注クラウド「newji」は、受発注部門で必要なQCD管理全てを備えた、現場特化型兼クラウド型の今世紀最高の受発注管理システムとなります。
ユーザー登録
受発注業務の効率化だけでなく、システムを導入することで、コスト削減や製品・資材のステータス可視化のほか、属人化していた受発注情報の共有化による内部不正防止や統制にも役立ちます。
NEWJI DX
製造業に特化したデジタルトランスフォーメーション(DX)の実現を目指す請負開発型のコンサルティングサービスです。AI、iPaaS、および先端の技術を駆使して、製造プロセスの効率化、業務効率化、チームワーク強化、コスト削減、品質向上を実現します。このサービスは、製造業の課題を深く理解し、それに対する最適なデジタルソリューションを提供することで、企業が持続的な成長とイノベーションを達成できるようサポートします。
製造業ニュース解説
製造業、主に購買・調達部門にお勤めの方々に向けた情報を配信しております。
新任の方やベテランの方、管理職を対象とした幅広いコンテンツをご用意しております。
お問い合わせ
コストダウンが利益に直結する術だと理解していても、なかなか前に進めることができない状況。そんな時は、newjiのコストダウン自動化機能で大きく利益貢献しよう!
(β版非公開)