- お役立ち記事
- Basics of Bayesian inference and its application to effective data analysis
Basics of Bayesian inference and its application to effective data analysis
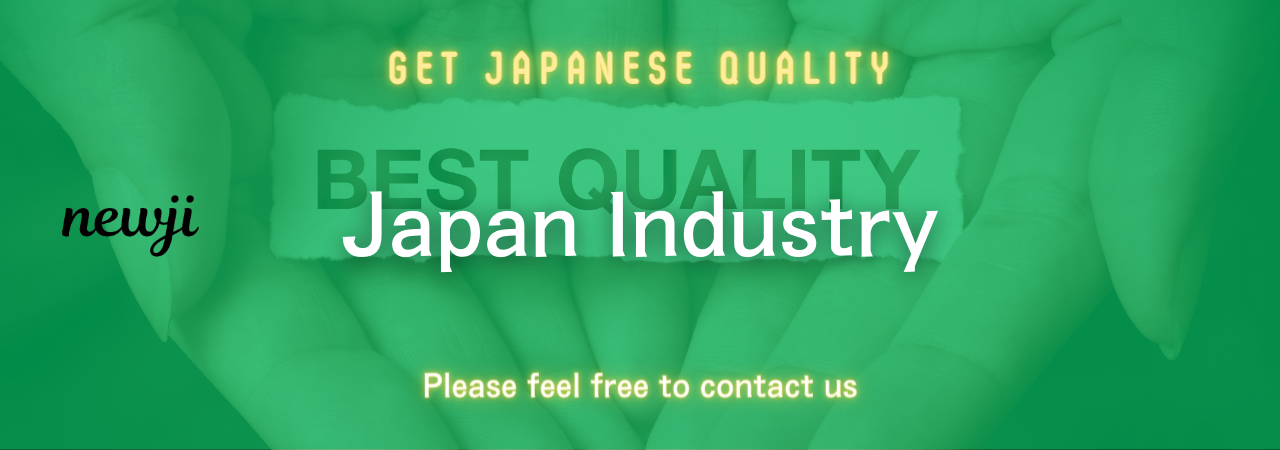
目次
Understanding Bayesian Inference
Bayesian inference is a powerful statistical method used to update the probability of a hypothesis as more evidence or information becomes available.
It is based on Bayes’ Theorem, named after the Reverend Thomas Bayes, an 18th-century statistician and theologian.
Bayes’ Theorem provides a way to revise existing predictions or beliefs in light of new evidence.
This method is particularly useful in fields where data is uncertain or incomplete, allowing for a more refined and accurate analysis.
The Bayesian approach contrasts with the traditional frequentist statistics, which rely solely on the data from the current experiment or study.
Bayesian inference combines this data with prior knowledge to make more informed predictions.
This amalgamation of prior beliefs with current data constitutes the core of Bayesian reasoning.
Bayes’ Theorem: The Foundation
At the heart of Bayesian inference lies Bayes’ Theorem.
The theorem is expressed as:
P(H|E) = [P(E|H) x P(H)] / P(E)
Here is what each element stands for:
– P(H|E): The probability of hypothesis H being true given the evidence E.
– P(E|H): The probability of observing evidence E given that hypothesis H is true.
– P(H): The prior probability of the hypothesis before observing the evidence.
– P(E): The probability of observing the evidence under all possible hypotheses.
Bayes’ Theorem enables one to update the probability estimate for a hypothesis as additional evidence is acquired.
Applications of Bayesian Inference
Bayesian inference finds applications across various fields due to its adaptability and robustness in handling uncertainty and imperfect data.
Medicine and Health
In the medical field, Bayesian methods are instrumental in diagnostic testing and clinical trials.
Healthcare professionals often deal with uncertain data and need to make crucial decisions based on incomplete information.
Bayesian inference helps in updating the likelihood of diseases based on new test results, improving diagnostic accuracy.
For example, when interpreting the result of a medical test, Bayesian inference can adjust the probability of having a disease by taking into account both the result of the test and the prior probability of the disease.
Machine Learning
In machine learning, Bayesian inference is used to build predictive models that can update themselves as new data becomes available.
This ability to incorporate prior knowledge with observed data makes Bayesian methods particularly valuable in developing algorithms that learn over time.
Bayesian networks, for example, are graphical models that use Bayes’ Theorem to efficiently compute the probabilities of different outcomes.
Economics and Finance
Economists and financial analysts use Bayesian inference to forecast economic trends or assess risks and returns.
By incorporating prior knowledge and economic indicators, Bayesian models can predict future market behaviors with a greater degree of accuracy.
They help in understanding complex financial systems where exact data may be difficult to obtain.
Advantages of Bayesian Inference
Bayesian inference offers several advantages over traditional statistical methods, making it a preferred choice in many areas of study.
Incorporating Prior Knowledge
One of the greatest strengths of Bayesian inference is its ability to incorporate prior knowledge into the analysis.
This is particularly beneficial when dealing with sparse or incomplete data.
By incorporating previous information, Bayesian analysis often provides more realistic estimates.
Flexibility and Adaptability
Bayesian models are inherently flexible and can adapt to changing information streams.
As new data becomes available, the probability estimates can be updated, ensuring that the model remains relevant and accurate over time.
Handling Uncertainty
Bayesian inference is adept at quantifying uncertainty.
Rather than providing a single point estimate, Bayesian analysis offers a probability distribution of possible outcomes.
This allows decision-makers to consider a range of possibilities and risks, leading to more informed decision-making.
Challenges and Considerations
Despite its many advantages, Bayesian inference is not without its challenges.
Complex Computations
Bayesian analysis often involves complex computations that can be resource-intensive.
This complexity increases with the size of the data and the number of parameters in the model.
Choice of Priors
Determining the appropriate prior probabilities is a crucial and sometimes contentious aspect of Bayesian inference.
The choice of prior can significantly influence the results, and improper priors may lead to misleading conclusions.
Interpretation
Interpreting the results of Bayesian analysis requires a good understanding of probability distributions and the underlying statistical concepts, which can be challenging for individuals without a statistical background.
Conclusion
Bayesian inference is a versatile and robust method for data analysis, offering a framework that integrates new information with existing knowledge.
Its ability to handle uncertainty and provide probabilistic insights makes it a valuable tool across numerous disciplines, from medicine to machine learning and finance.
While challenges such as computational complexity and the choice of priors exist, the advantages of Bayesian methods in providing comprehensive and flexible analyses make them indispensable in our data-driven world.
By adopting a Bayesian approach, analysts and researchers can make more informed decisions, ultimately leading to better outcomes.
資料ダウンロード
QCD調達購買管理クラウド「newji」は、調達購買部門で必要なQCD管理全てを備えた、現場特化型兼クラウド型の今世紀最高の購買管理システムとなります。
ユーザー登録
調達購買業務の効率化だけでなく、システムを導入することで、コスト削減や製品・資材のステータス可視化のほか、属人化していた購買情報の共有化による内部不正防止や統制にも役立ちます。
NEWJI DX
製造業に特化したデジタルトランスフォーメーション(DX)の実現を目指す請負開発型のコンサルティングサービスです。AI、iPaaS、および先端の技術を駆使して、製造プロセスの効率化、業務効率化、チームワーク強化、コスト削減、品質向上を実現します。このサービスは、製造業の課題を深く理解し、それに対する最適なデジタルソリューションを提供することで、企業が持続的な成長とイノベーションを達成できるようサポートします。
オンライン講座
製造業、主に購買・調達部門にお勤めの方々に向けた情報を配信しております。
新任の方やベテランの方、管理職を対象とした幅広いコンテンツをご用意しております。
お問い合わせ
コストダウンが利益に直結する術だと理解していても、なかなか前に進めることができない状況。そんな時は、newjiのコストダウン自動化機能で大きく利益貢献しよう!
(Β版非公開)