- お役立ち記事
- Basics of Bayesian statistics and how to effectively utilize them for data analysis
月間76,176名の
製造業ご担当者様が閲覧しています*
*2025年3月31日現在のGoogle Analyticsのデータより
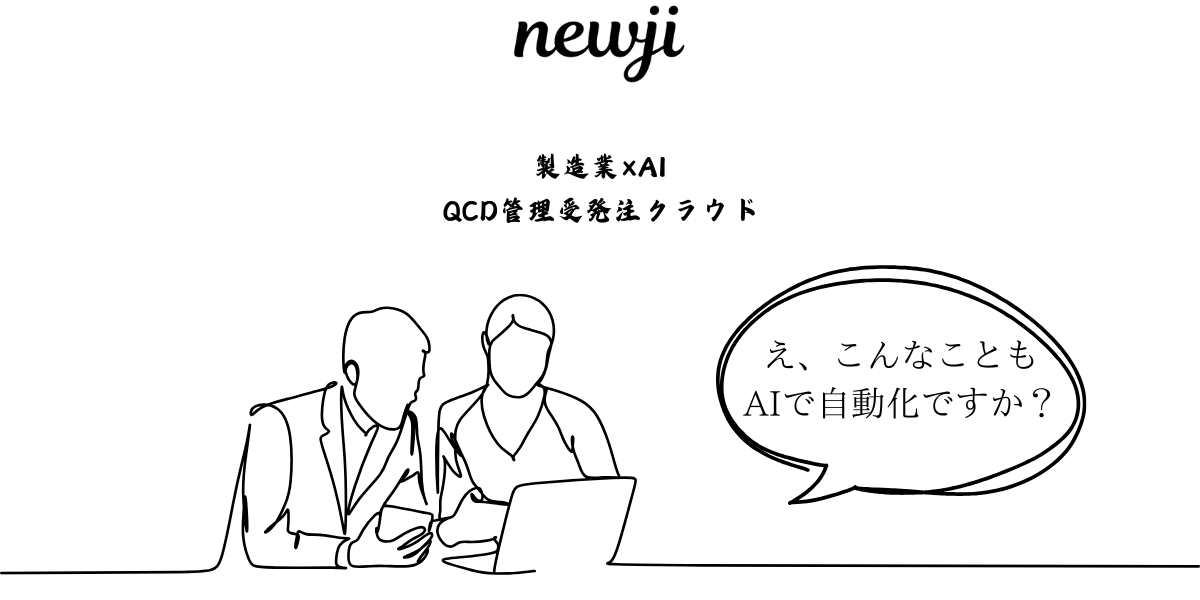
Basics of Bayesian statistics and how to effectively utilize them for data analysis
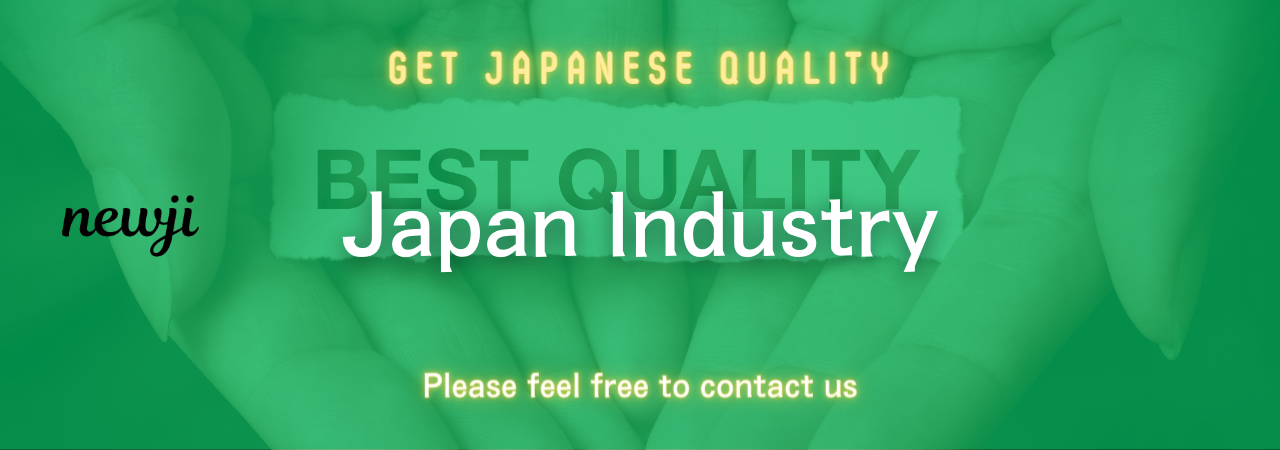
目次
Understanding Bayesian Statistics
Bayesian statistics is a field of statistical analysis that has found widespread application in various domains, primarily due to its unique approach to probability and decision-making under uncertainty.
At its core, Bayesian statistics revolves around the concept of updating our beliefs or knowledge based on new evidence or information.
This approach draws from Bayes’ Theorem, a fundamental concept named after the statistician Thomas Bayes.
To grasp the basis of Bayesian statistics, it’s crucial to understand how it differs from the frequentist approach, which is another major school of thought in statistics.
Frequentist statistics interprets probability as the long-run frequency of events.
In contrast, Bayesian statistics views probability as a measure of belief or certainty about an event.
This makes Bayesian methods more flexible and applicable to real-world scenarios where prior knowledge can be incorporated into the analysis.
The Role of Bayes’ Theorem
Bayes’ Theorem is the foundation of Bayesian statistics.
It provides a mathematical formula to update the probability of a hypothesis based on new evidence.
The theorem is expressed as:
**P(H|E) = (P(E|H) * P(H)) / P(E)**
Here’s what each part of the equation represents:
– **P(H|E)**: The posterior probability, or the probability of the hypothesis (H) given the observed evidence (E).
– **P(E|H)**: The likelihood, or the probability of observing the evidence (E) if the hypothesis (H) is true.
– **P(H)**: The prior probability, our initial belief about the hypothesis before seeing the evidence.
– **P(E)**: The marginal likelihood, the overall probability of the evidence.
By using Bayes’ Theorem, we start with a prior belief (prior probability) about a hypothesis and revise it to form a new belief (posterior probability) in light of new data.
Applications of Bayesian Statistics
Bayesian statistics has diverse applications across fields, offering a robust framework for making decisions in uncertain environments.
Here are some key areas where Bayesian methods are utilized:
Medical Diagnosis
In healthcare, Bayesian statistics is often used for diagnostic testing.
Doctors can update their assessment of a patient’s condition as new test results become available.
For example, if a medical test suggests a certain disease, Bayesian approaches would update the probability of the disease based on pre-test probabilities (such as risk factors) and the test’s characteristics.
Machine Learning
Bayesian methods are fundamental in machine learning for tasks such as classification, regression, and clustering.
In particular, Bayesian networks and Bayesian inference are employed to model complex relationships between variables and handle uncertain information effectively.
This makes it particularly useful for tasks like image recognition and natural language processing.
Financial Modeling
In finance, Bayesian techniques are used for constructing probabilistic models in portfolio management and risk assessment.
Traders and analysts utilize Bayesian inference to update the likelihood of market trends and investment outcomes based on historical data and new information.
Marketing and Customer Analysis
Bayesian statistics also play a critical role in marketing and customer behavior analysis.
By analyzing customer data through a Bayesian lens, businesses can improve personalized marketing strategies and predict customer churn, optimizing their resources in a targeted manner.
Effective Utilization of Bayesian Statistics in Data Analysis
Understanding the execution of Bayesian statistics in data analysis involves several critical steps.
These steps ensure that data scientists and researchers can leverage Bayesian concepts efficiently for insightful outcomes.
Define the Prior Distribution
Incorporating prior knowledge into your analysis is a distinctive feature of Bayesian statistics.
This first step involves meticulously defining your prior beliefs or knowledge about the hypothesis or parameters you are investigating.
The prior distribution can be subjective and is often chosen based on historical data, expert opinion, or symmetry considerations.
Establish the Likelihood
Next, it is necessary to develop a likelihood function, which models the probability of observed data given the parameters of interest.
The likelihood helps connect the model to the actual data being analyzed.
Choosing the correct likelihood function is critical, as it reflects the underlying process generating the data.
Apply Bayes’ Theorem
Using Bayes’ Theorem, you calculate the posterior distribution by combining the prior distribution and the likelihood of new data.
Mathematically, this step is achieved by integrating the prior distribution with the likelihood to provide the updated or posterior beliefs.
Compute and Interpret Posterior Distribution
The posterior distribution is the focal result of Bayesian analysis.
This distribution provides a range of plausible values for the model parameters after considering the data.
Interpreting the posterior involves understanding how the likelihood and priors combine to modify the initial beliefs in light of evidence.
Perform Bayesian Inference
Bayesian inference allows you to derive specific conclusions from the posterior distribution.
This involves generating point estimates, interval estimates, or predictive distributions needed for decision-making.
By analyzing credible intervals and hypothesis testing in the Bayesian framework, more informed decisions can be drawn from data insights.
Challenges and Considerations
While Bayesian statistics offer significant advantages in terms of flexibility and incorporation of prior knowledge, there are challenges to keep in mind.
– **Choosing the Right Prior**: Selecting an appropriate prior is subjective and can influence results significantly, especially in the case of limited data. Realizing the impact of your choice of prior can help refine and validate your analysis.
– **Computational Complexity**: Bayesian methods can be computationally intensive, especially for complex models. Utilizing modern computational tools like Markov Chain Monte Carlo (MCMC) can alleviate but not completely eliminate these difficulties.
– **Data Requirements**: In scenarios with ample data, the influence of the prior diminishes, but in small data sets, priors become crucial, requiring careful selection and testing.
By understanding these considerations, statisticians and data analysts can effectively apply Bayesian methods to derive valuable insights from complex datasets.
Recognizing the impact and potential of Bayesian statistics is essential for anyone involved in data analysis.
Incorporating Bayesian approaches equips analysts with a more comprehensive toolkit for interpreting and making predictions based on data, ultimately leading to more informed and accurate decisions across various fields.
資料ダウンロード
QCD管理受発注クラウド「newji」は、受発注部門で必要なQCD管理全てを備えた、現場特化型兼クラウド型の今世紀最高の受発注管理システムとなります。
ユーザー登録
受発注業務の効率化だけでなく、システムを導入することで、コスト削減や製品・資材のステータス可視化のほか、属人化していた受発注情報の共有化による内部不正防止や統制にも役立ちます。
NEWJI DX
製造業に特化したデジタルトランスフォーメーション(DX)の実現を目指す請負開発型のコンサルティングサービスです。AI、iPaaS、および先端の技術を駆使して、製造プロセスの効率化、業務効率化、チームワーク強化、コスト削減、品質向上を実現します。このサービスは、製造業の課題を深く理解し、それに対する最適なデジタルソリューションを提供することで、企業が持続的な成長とイノベーションを達成できるようサポートします。
製造業ニュース解説
製造業、主に購買・調達部門にお勤めの方々に向けた情報を配信しております。
新任の方やベテランの方、管理職を対象とした幅広いコンテンツをご用意しております。
お問い合わせ
コストダウンが利益に直結する術だと理解していても、なかなか前に進めることができない状況。そんな時は、newjiのコストダウン自動化機能で大きく利益貢献しよう!
(β版非公開)