- お役立ち記事
- Basics of deep learning using Python and its application to efficient data analysis
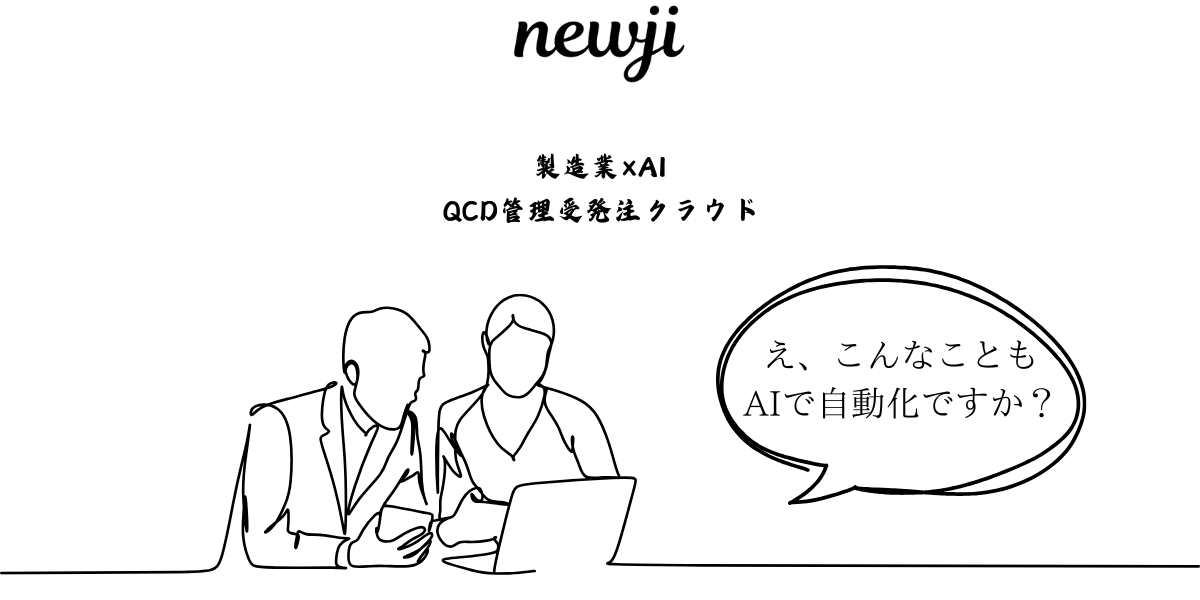
Basics of deep learning using Python and its application to efficient data analysis
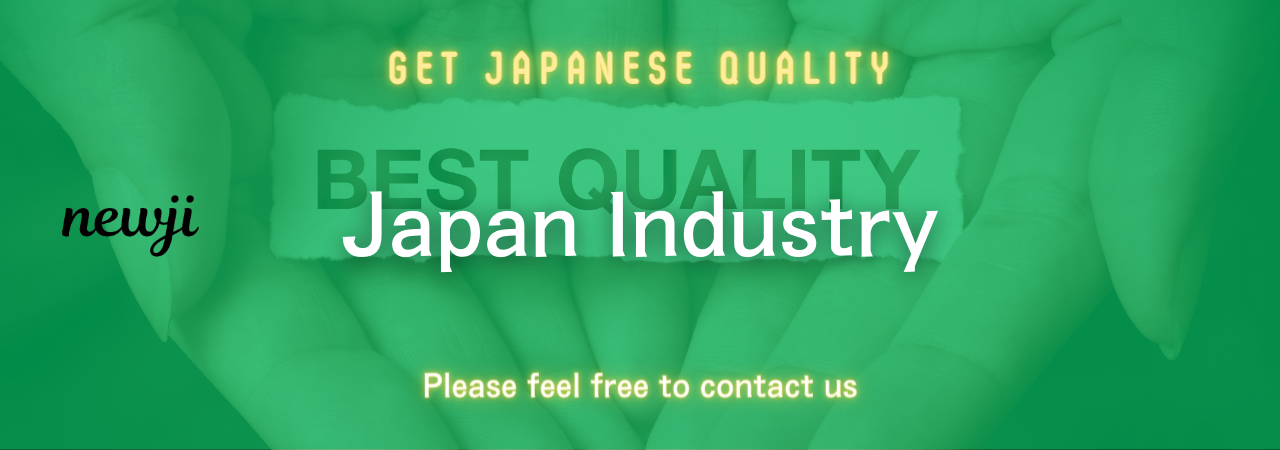
目次
Introduction to Deep Learning with Python
Deep learning is a subfield of machine learning that is attracting substantial attention due to its ability to handle vast amounts of data and solve complex problems.
Python, a versatile programming language, plays a pivotal role in implementing deep learning models.
This article will delve into the basics of deep learning, provide an overview of its components, and explore how it can be applied to data analysis efficiently.
Understanding Deep Learning
Deep learning mimics the human brain’s neural networks, composed of layers of interconnected nodes or neurons.
These networks learn patterns within data through iterative processes, improving their performance over time.
Deep learning models can handle unstructured data such as images, audio, and text, making them suitable for diverse applications.
Components of Deep Learning
Several critical components form the foundation of deep learning.
Understanding these elements is essential to grasp how deep learning models function.
Neural Networks
At the core of deep learning are neural networks, which consist of input, hidden, and output layers.
The input layer receives raw data, while the hidden layers process this information through weighted connections.
The output layer generates the final results.
Activation Functions
Activation functions determine whether a neuron should be activated or not, adding non-linear properties to the model.
Common activation functions include ReLU (Rectified Linear Unit), sigmoid, and tanh, each with unique characteristics.
Backpropagation
Backpropagation is the process of updating the weights in a neural network.
This technique reduces the error rate by adjusting weights and biases during training, enabling the model to improve its predictions.
Optimization Algorithms
Optimization algorithms, such as Stochastic Gradient Descent (SGD) and Adam, are used to minimize the loss function, helping the model converge toward an optimal solution.
Python’s Role in Deep Learning
Python’s prominence in deep learning can be attributed to its simplicity and robust ecosystem of libraries.
Two of the most widely used libraries in Python for deep learning are TensorFlow and Keras.
TensorFlow
Developed by Google, TensorFlow is a powerful open-source library for building and deploying deep learning models.
It offers a comprehensive suite of tools for defining, training, and validating models, making it suitable for beginners and experts alike.
Keras
Keras is a high-level neural networks API written in Python, running on top of TensorFlow.
It simplifies the process of building complex models and provides excellent support for customization, allowing developers to focus on defining layer structures.
Building a Simple Deep Learning Model
To understand how deep learning can be applied to data analysis, let’s explore the process of building a simple model using Python.
We will use the Keras library to construct a basic neural network for a classification problem.
Data Preparation
Before building a model, we need to prepare the data.
This involves loading datasets, handling missing values, scaling features, and splitting data into training and testing sets.
Defining the Model
In Keras, we define the architecture of our model using a Sequential class.
We can add layers, specify the number of neurons, and select appropriate activation functions.
“`python
from keras.models import Sequential
from keras.layers import Dense
# Create the model
model = Sequential()
model.add(Dense(64, input_dim=input_shape, activation=’relu’))
model.add(Dense(32, activation=’relu’))
model.add(Dense(num_classes, activation=’softmax’))
“`
Compiling the Model
Compiling the model involves configuring the optimizer, setting the loss function, and defining evaluation metrics.
“`python
model.compile(optimizer=’adam’, loss=’categorical_crossentropy’, metrics=[‘accuracy’])
“`
Training the Model
Training the model involves fitting it to the training data, specifying epochs, and batch size.
During this phase, the model learns from the input data by updating its weights.
“`python
model.fit(X_train, y_train, epochs=10, batch_size=32)
“`
Evaluating the Model
After training, we evaluate the model using the testing set to gauge its performance and generalization capabilities.
“`python
loss, accuracy = model.evaluate(X_test, y_test)
print(f”Model Accuracy: {accuracy}”)
“`
Applications of Deep Learning in Data Analysis
Deep learning enhances data analysis by uncovering intricate patterns and insights.
Here are some practical applications:
Image and Video Analysis
Deep learning models excel at analyzing images and videos, enabling applications in facial recognition, object detection, and medical imaging.
Natural Language Processing (NLP)
In NLP, deep learning models process and understand human language, facilitating tasks such as sentiment analysis, language translation, and chatbot development.
Time Series Analysis
Deep learning can model temporal dependencies in time series data, making it suitable for applications like stock market prediction and anomaly detection.
Fraud Detection
By identifying patterns and anomalies in transactional data, deep learning models help detect fraudulent activities across various industries.
Conclusion
Deep learning, a powerful tool in the realm of artificial intelligence, offers significant potential for efficient data analysis.
Python’s accessibility and extensive libraries make it an ideal choice for implementing deep learning models.
By understanding the fundamentals and leveraging its capabilities, beginners and practitioners can unlock valuable insights from complex datasets.
資料ダウンロード
QCD調達購買管理クラウド「newji」は、調達購買部門で必要なQCD管理全てを備えた、現場特化型兼クラウド型の今世紀最高の購買管理システムとなります。
ユーザー登録
調達購買業務の効率化だけでなく、システムを導入することで、コスト削減や製品・資材のステータス可視化のほか、属人化していた購買情報の共有化による内部不正防止や統制にも役立ちます。
NEWJI DX
製造業に特化したデジタルトランスフォーメーション(DX)の実現を目指す請負開発型のコンサルティングサービスです。AI、iPaaS、および先端の技術を駆使して、製造プロセスの効率化、業務効率化、チームワーク強化、コスト削減、品質向上を実現します。このサービスは、製造業の課題を深く理解し、それに対する最適なデジタルソリューションを提供することで、企業が持続的な成長とイノベーションを達成できるようサポートします。
オンライン講座
製造業、主に購買・調達部門にお勤めの方々に向けた情報を配信しております。
新任の方やベテランの方、管理職を対象とした幅広いコンテンツをご用意しております。
お問い合わせ
コストダウンが利益に直結する術だと理解していても、なかなか前に進めることができない状況。そんな時は、newjiのコストダウン自動化機能で大きく利益貢献しよう!
(Β版非公開)