- お役立ち記事
- Basics of experimental design and practical points for effective data analysis
月間77,185名の
製造業ご担当者様が閲覧しています*
*2025年2月28日現在のGoogle Analyticsのデータより
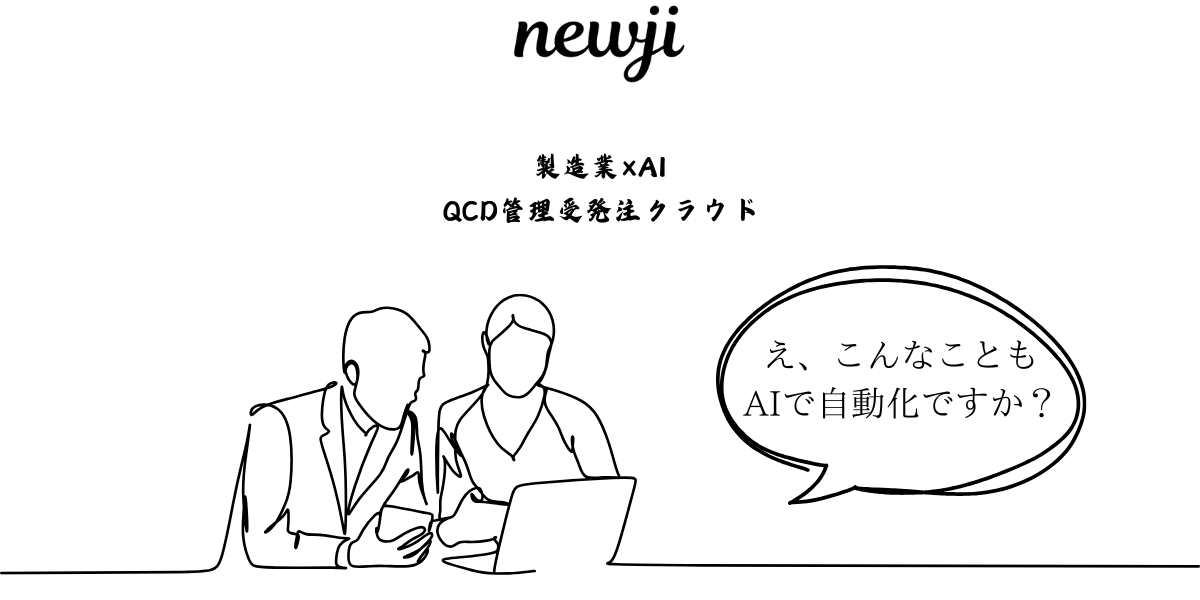
Basics of experimental design and practical points for effective data analysis
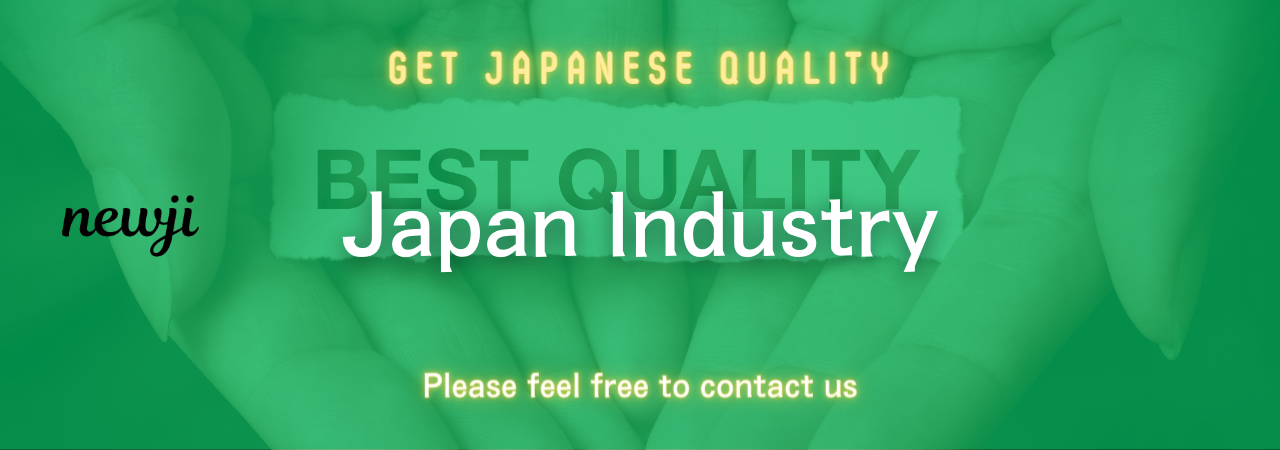
目次
Understanding Experimental Design
Experimental design is a foundational concept in both scientific research and data analysis.
At its core, experimental design is about planning how to gather data efficiently and effectively to answer specific questions.
This involves a structured approach to experimentation, ensuring that the data collected are valid and reliable.
An experiment typically involves manipulation of independent variables to observe their effect on dependent variables.
Proper experimental design helps researchers control extraneous variables, ensuring that the results are due solely to the manipulation of the independent variable.
The basics of experimental design require clear planning and execution, including defining variables, selecting participants, and deciding on procedures for data collection.
Key Components of Experimental Design
When designing an experiment, it is critical to consider several components that will ultimately impact the validity and reliability of the results.
Identifying Variables
Firstly, the experimenter must identify the independent variables, which they manipulate, and the dependent variables, which are affected by the change.
Additionally, any extraneous variables should be noted so that they can be controlled or accounted for in the analysis.
Experimental Groups and Control Groups
Next, experiments often involve an experimental group that receives the treatment and a control group that does not receive the treatment.
The control group serves as a baseline to compare against the effects observed in the experimental group.
Randomization
Randomization is a vital aspect of experimental design, where subjects are randomly assigned to either the control or experimental group.
This process minimizes the potential for bias, ensuring that any differences observed between groups are due to the treatment rather than pre-existing differences.
Replicability and Sample Size
Experiments must be replicable, meaning that they can be repeated under the same conditions.
To increase reliability, a sufficient sample size should be chosen so that the results can be generalized to a larger population.
Practical Points for Effective Data Analysis
Effective data analysis is just as critical as the experimental design itself.
Even the best-designed experiment can yield inconclusive results if the data is not accurately analyzed.
Descriptive Statistics
Descriptive statistics give a summary of the data set, providing insights into the data’s basic features.
They include measures of central tendency (mean, median, mode) and measures of variability (range, variance, standard deviation).
These statistics help the researcher understand the distribution and spread of the data, providing a snapshot of the overall trend.
Inferential Statistics
Inferential statistics allow researchers to make predictions or inferences about a larger population based on sample data.
Common tests include the t-test, ANOVA, and chi-square test, each useful for different types of data and experimental conditions.
Choosing the correct test is vital for analyzing the data accurately.
Data Visualization
Visualizing data through graphs and charts provides a clear and quick way to communicate findings.
Graphs like histograms, scatter plots, and bar charts offer unique insights and can highlight patterns that may not be immediately obvious in raw data.
Choosing the right visualization is crucial for effectively interpreting and presenting the data.
Assumptions and Validity
Every statistical test rests on certain assumptions.
Before analyzing data, it’s crucial to verify if these assumptions hold true for your dataset.
For instance, many parametric tests assume that data follows a normal distribution and that variances are equal across groups.
Similarly, the validity of the experiment should be checked to ensure that the results accurately represent what they are intended to measure.
Challenges and Considerations in Experimental Research
Despite the structured process of experimental design and data analysis, researchers often face challenges and must make important decisions.
Dealing with Bias and Confounding Variables
Bias and confounding variables can skew results.
Bias may result from the experimenter’s influence, participant selection, or measurement methods.
Strategies to mitigate these include blinding (ensuring the subjects/experimenters are unaware of key aspects of the experiment), randomization, and ensuring proper control measures are in place.
Ethical Considerations
Ethics play a crucial role in experimental research.
Ensuring participant safety and obtaining informed consent are critical.
Researchers must also be mindful of data privacy and adhere to ethical guidelines when designing and conducting experiments.
Interpreting Non-Significant Results
Not all experiments will yield significant findings.
A non-significant result does not mean the hypothesis is false; it might indicate that the study was underpowered or that there’s no effect to be observed.
Interpreting these results requires careful consideration and often leads to further investigation.
The Importance of Proper Reporting
Once an experiment concludes, and the data is analyzed, it’s essential to report findings accurately and transparently.
Writing a Clear and Comprehensive Report
A well-written report includes an introduction to the research question, the methodology (including the design and procedures), the results (both statistical analyses and visualizations), and a discussion interpreting these findings in the context of previous research.
Sharing Data and Methods
Transparency involves sharing data and methods, allowing others to verify and replicate findings.
This openness fosters trust in results and promotes further scientific inquiries.
Experimental design and effective data analysis are inseparable parts of the research process.
By understanding and applying these principles, researchers can ensure that their findings are not only credible but also contribute valuable insights into their field of study.
With careful planning, execution, and analysis, experiments can lead to breakthroughs and advancements in knowledge.
資料ダウンロード
QCD管理受発注クラウド「newji」は、受発注部門で必要なQCD管理全てを備えた、現場特化型兼クラウド型の今世紀最高の受発注管理システムとなります。
ユーザー登録
受発注業務の効率化だけでなく、システムを導入することで、コスト削減や製品・資材のステータス可視化のほか、属人化していた受発注情報の共有化による内部不正防止や統制にも役立ちます。
NEWJI DX
製造業に特化したデジタルトランスフォーメーション(DX)の実現を目指す請負開発型のコンサルティングサービスです。AI、iPaaS、および先端の技術を駆使して、製造プロセスの効率化、業務効率化、チームワーク強化、コスト削減、品質向上を実現します。このサービスは、製造業の課題を深く理解し、それに対する最適なデジタルソリューションを提供することで、企業が持続的な成長とイノベーションを達成できるようサポートします。
製造業ニュース解説
製造業、主に購買・調達部門にお勤めの方々に向けた情報を配信しております。
新任の方やベテランの方、管理職を対象とした幅広いコンテンツをご用意しております。
お問い合わせ
コストダウンが利益に直結する術だと理解していても、なかなか前に進めることができない状況。そんな時は、newjiのコストダウン自動化機能で大きく利益貢献しよう!
(β版非公開)