- お役立ち記事
- Basics of experimental design, data analysis methods, and practical points
月間76,176名の
製造業ご担当者様が閲覧しています*
*2025年3月31日現在のGoogle Analyticsのデータより
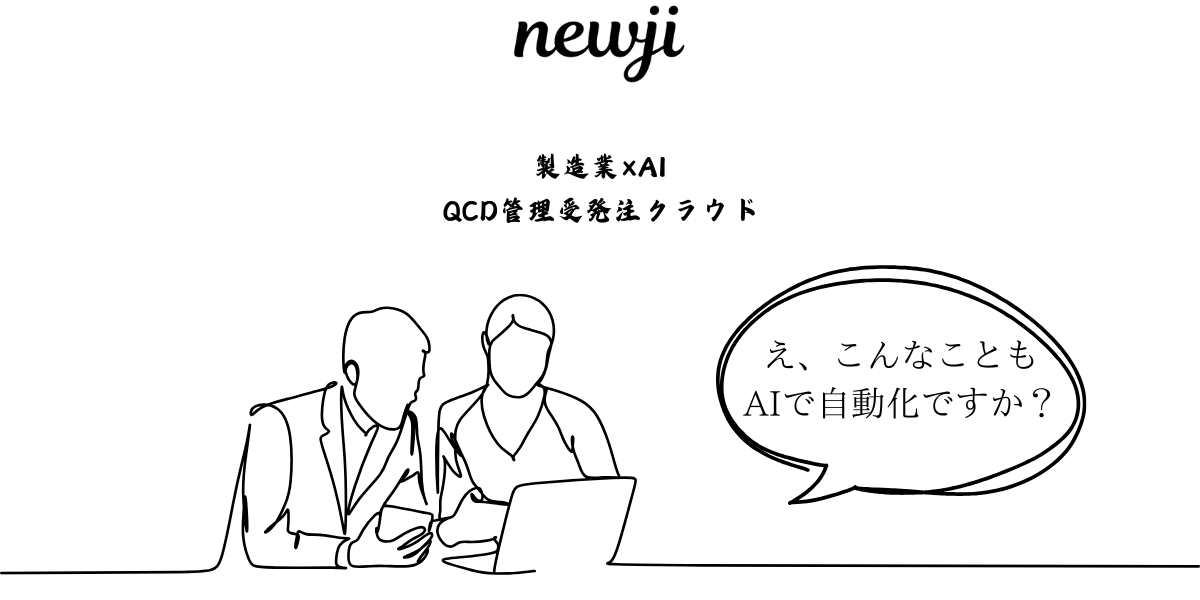
Basics of experimental design, data analysis methods, and practical points
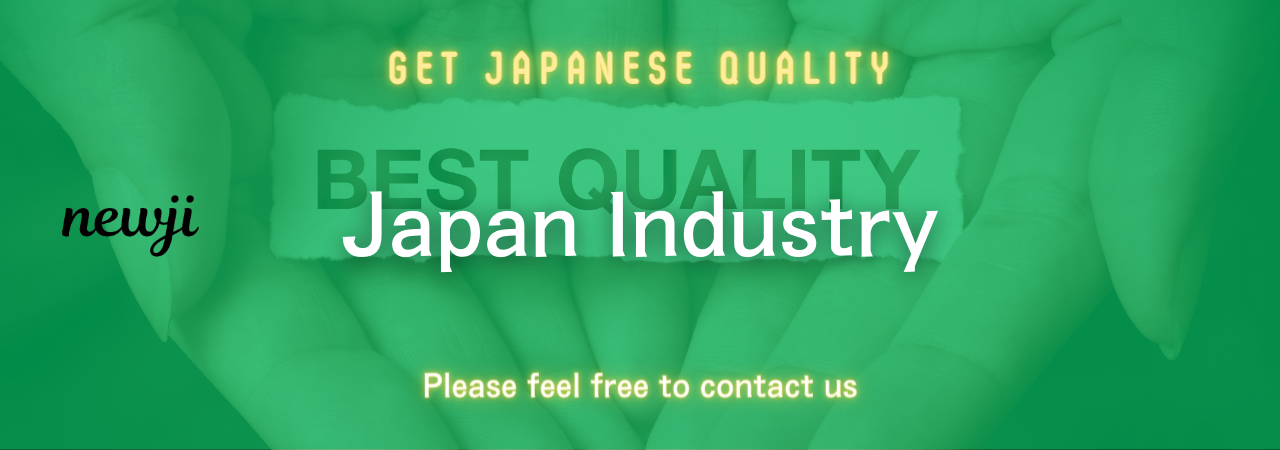
目次
Understanding Experimental Design
Experimental design is a fundamental aspect of scientific research that helps in structuring experiments to answer specific questions or test hypotheses.
One of the primary goals of experimental design is to ensure that the data collected are reliable and valid, so researchers can draw accurate conclusions.
Designing an experiment involves several critical steps including identifying the problem, defining the variables, and planning how to collect and analyze data efficiently.
A well-structured experimental design reduces potential biases and errors, leading to more credible and reproducible results.
There are several types of experimental designs to consider, such as completely randomized designs, randomized block designs, and factorial designs.
Each design type has its own advantages and use cases, depending on the research question and the nature of the variables involved.
Before starting an experiment, it’s crucial to understand which design best suits your needs.
Key Components of Experimental Design
Every experimental design consists of essential components, including independent and dependent variables, control groups, and random assignment.
The independent variable is the factor that is manipulated by the researcher to observe its effects.
The dependent variable is the outcome that is measured to see how it is influenced by changes in the independent variable.
Control groups are used to provide a baseline for comparison, ensuring that the effects observed in the experiment are due to the manipulation of the independent variable.
Random assignment is a process used to eliminate selection bias by randomly assigning participants or subjects to the various groups within an experiment.
This helps ensure that the groups are equivalent at the start of the experiment, making the results more reliable.
Data Analysis Methods
Once data has been collected from an experiment, data analysis is the next critical step.
Data analysis involves examining, cleaning, and modeling data to uncover useful information, draw conclusions, and support decision-making.
There are many methods of data analysis, ranging from simple descriptive statistics like means and medians to more complex inferential statistics.
Descriptive statistics provide a summary of the data, offering insights into patterns and distributions.
Inferential statistics allow researchers to draw conclusions about a population based on a sample.
This includes hypothesis testing, regression analysis, and analysis of variance (ANOVA), among other techniques.
Choosing the right method of analysis depends on the research question, the type of data collected, and the design of the experiment.
Statistical software tools such as SPSS, R, and Python are commonly used to perform data analysis and manage large datasets.
These tools can help streamline the analysis process, making it easier to interpret and communicate the results.
Practical Points in Experimental Design and Data Analysis
When conducting experiments and analyzing data, there are several practical points to keep in mind to ensure the validity and reliability of results.
Firstly, always pre-define your hypothesis and objectives clearly before starting your experiment.
This serves as a guide throughout the experimental process and helps in maintaining focus.
Always ensure that your data collection methods are consistent and systematic to minimize errors.
Double-check for any missing or erroneous data, as this can significantly impact the results of your analysis.
It’s also important to consider the sample size, as a larger sample size generally increases the reliability of the results.
However, balancing this with resource constraints is often necessary.
In terms of data analysis, make sure to choose statistical tests that are appropriate for the type of data and research question at hand.
Be transparent in your methodology, data collection, and analysis processes to facilitate reproducibility.
This means documenting your workflow and providing access to raw datasets, when possible.
Finally, consider the ethical implications of your experimental design and data analysis.
This includes obtaining informed consent if working with human subjects, and ensuring privacy and data protection measures are in place.
Conclusion
Understanding the basics of experimental design and data analysis methods is crucial for conducting effective research.
A well-crafted experimental design helps minimize biases and errors, while robust data analysis methods ensure accurate interpretation of results.
By keeping practical points in mind, researchers can enhance the validity, reliability, and reproducibility of their experiments.
The ability to critically design experiments and analyze data effectively can lead to significant scientific discoveries and informed decision-making.
資料ダウンロード
QCD管理受発注クラウド「newji」は、受発注部門で必要なQCD管理全てを備えた、現場特化型兼クラウド型の今世紀最高の受発注管理システムとなります。
ユーザー登録
受発注業務の効率化だけでなく、システムを導入することで、コスト削減や製品・資材のステータス可視化のほか、属人化していた受発注情報の共有化による内部不正防止や統制にも役立ちます。
NEWJI DX
製造業に特化したデジタルトランスフォーメーション(DX)の実現を目指す請負開発型のコンサルティングサービスです。AI、iPaaS、および先端の技術を駆使して、製造プロセスの効率化、業務効率化、チームワーク強化、コスト削減、品質向上を実現します。このサービスは、製造業の課題を深く理解し、それに対する最適なデジタルソリューションを提供することで、企業が持続的な成長とイノベーションを達成できるようサポートします。
製造業ニュース解説
製造業、主に購買・調達部門にお勤めの方々に向けた情報を配信しております。
新任の方やベテランの方、管理職を対象とした幅広いコンテンツをご用意しております。
お問い合わせ
コストダウンが利益に直結する術だと理解していても、なかなか前に進めることができない状況。そんな時は、newjiのコストダウン自動化機能で大きく利益貢献しよう!
(β版非公開)