- お役立ち記事
- Basics of experimental design using response surface methodology and points for efficient practical use
月間76,176名の
製造業ご担当者様が閲覧しています*
*2025年3月31日現在のGoogle Analyticsのデータより
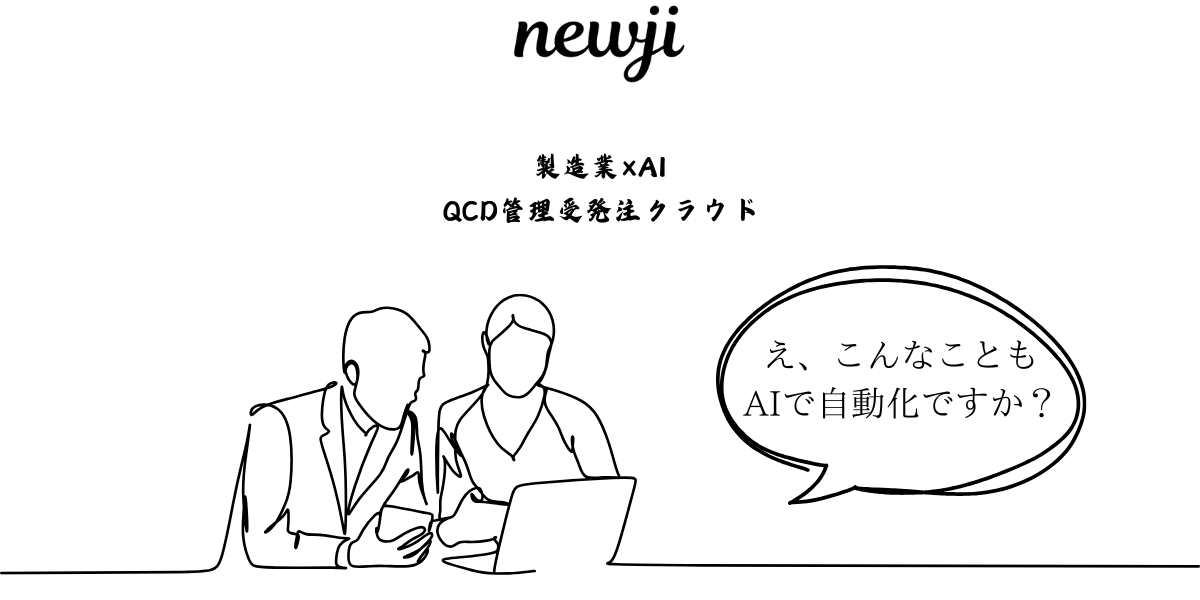
Basics of experimental design using response surface methodology and points for efficient practical use
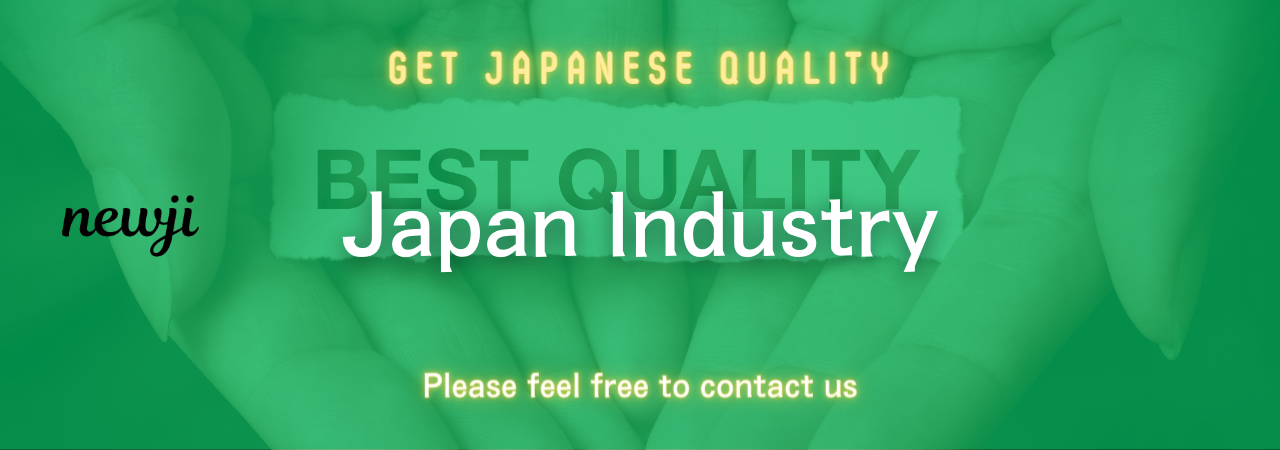
目次
Understanding Response Surface Methodology (RSM)
Response Surface Methodology (RSM) is a collection of mathematical and statistical techniques that are useful for modeling and analyzing problems where several variables influence a response of interest.
The goal of RSM is to optimize this response, which is why it is widely used in industrial applications and experimental research.
This method not only helps in finding the optimal conditions for desirable outcomes but also aids in identifying the relationship between the response and the input variables.
RSM involves the use of designed experiments to obtain data using statistical techniques.
These data are then used to generate a mathematical model—a response surface—that represents the relationship between inputs and the desired output.
The analysis compiles to showcase the effects and interactions of variables on the response.
The Role of Experimental Design in RSM
In RSM, the experimental design plays a critical role in collecting accurate data to develop a reliable model.
The design of experiments (DOE) is used to systematically change the input variables, ensuring that the data collected is both exhaustive and efficient in exploring the experimental space.
Choosing the right experimental design is crucial because it influences the efficiency of the data collection process, the accuracy of the model, and the reliability of the conclusions drawn from the study.
Common experimental designs used in RSM include factorial designs, central composite designs (CCD), and Box-Behnken designs.
Each design has its applications and is suited for different scenarios depending on the complexity of the problem.
Factorial Designs
Factorial designs are useful for experiments involving two or more factors, allowing researchers to study the interaction effects between multiple variables.
In a full factorial design, experiments are performed at all possible combinations of the factor levels.
These designs are comprehensive but can become impractical with a large number of factors.
Central Composite Designs (CCD)
CCD is one of the most commonly used designs in RSM because of its efficiency and robustness.
It consists of a factorial design with added center and axial points that allow estimation of the curvature in the response surface.
CCD offers an optimal balance between the number of experimental runs and the ability to model quadratic surfaces effectively.
Box-Behnken Designs
Box-Behnken designs are another popular choice, known for their efficiency in generating a second-order model without requiring extreme factor combinations.
In these designs, experiments are done at the midpoints of edges of the process space and the center.
This makes Box-Behnken designs more economical when compared to other methods.
Steps in Conducting an RSM Experiment
Conducting an RSM experiment involves several important steps to ensure the design is efficient and the outcomes are reliable.
1. Define the Problem
The first step is to clearly define the objective of the study.
Identify the response variable to be optimized and determine the factors (independent variables) that influence this response.
2. Select the Experimental Design
Choose an appropriate experimental design that will provide the most information about the factor effects on the response while optimizing resource expenditure.
Consideration of the expected complexity of the response surface will guide this decision.
3. Collect and Analyze Data
Carry out the experiments according to the design plan.
Collect the data on the response variable for all combinations of factor levels.
This data forms the basis for model construction and further statistical analysis.
4. Develop a Mathematical Model
Use the data to develop a mathematical relationship between the independent variables and the response.
Techniques such as least squares regression are commonly used for this purpose.
The resulting model usually takes the form of a polynomial equation.
5. Perform Optimization
With the model in place, optimization techniques can predict the optimal set of conditions for the desired response.
Optimization can include examining response surfaces and contour plots.
6. Validate the Model
Validate the developed model by performing confirmation runs.
These are additional experiments using the predicted optimal conditions.
If the outcomes are close to predictions, the model is considered valid and reliable.
Efficient Practical Use of RSM
The practical use of RSM can be enhanced by considering several efficient strategies.
Use RSM Software Tools
Taking advantage of specialized software tools like Design-Expert, Minitab, or JMP can streamline the process of design, analysis, and optimization.
These tools provide templates for different experimental designs and facilitate model fitting and visualization.
Adopt Sequential Experimentation
Begin with a screening design to identify the most significant factors.
Follow this up with more detailed experiments to refine the model.
This approach is more resource-efficient than attempting a comprehensive study in one go.
Ensure Adequate Replication
Replication of experiments increases the reliability and precision of findings.
It improves the accuracy of estimating the response through averages and helps in error estimation.
Pay Attention to Resource Constraints
Resource constraints such as budget, time, and availability of materials should be considered when planning experiments.
Choose designs that maximize information gained for the resources available.
Conclusion
Response Surface Methodology is a powerful tool in experimental design, offering insights into the effects of variables and their interactions in complex systems.
By choosing the appropriate design, robust models can be developed, leading to optimal process conditions.
Efficient practical use involves a strategic approach to experimentation, taking advantage of modern tools and allowing for systematic exploration and validation of results.
Overall, RSM equips researchers and engineers with a structured method for optimizing responses in varied applications.
資料ダウンロード
QCD管理受発注クラウド「newji」は、受発注部門で必要なQCD管理全てを備えた、現場特化型兼クラウド型の今世紀最高の受発注管理システムとなります。
ユーザー登録
受発注業務の効率化だけでなく、システムを導入することで、コスト削減や製品・資材のステータス可視化のほか、属人化していた受発注情報の共有化による内部不正防止や統制にも役立ちます。
NEWJI DX
製造業に特化したデジタルトランスフォーメーション(DX)の実現を目指す請負開発型のコンサルティングサービスです。AI、iPaaS、および先端の技術を駆使して、製造プロセスの効率化、業務効率化、チームワーク強化、コスト削減、品質向上を実現します。このサービスは、製造業の課題を深く理解し、それに対する最適なデジタルソリューションを提供することで、企業が持続的な成長とイノベーションを達成できるようサポートします。
製造業ニュース解説
製造業、主に購買・調達部門にお勤めの方々に向けた情報を配信しております。
新任の方やベテランの方、管理職を対象とした幅広いコンテンツをご用意しております。
お問い合わせ
コストダウンが利益に直結する術だと理解していても、なかなか前に進めることができない状況。そんな時は、newjiのコストダウン自動化機能で大きく利益貢献しよう!
(β版非公開)