- お役立ち記事
- Basics of feature engineering using machine learning and application to materials DX
Basics of feature engineering using machine learning and application to materials DX
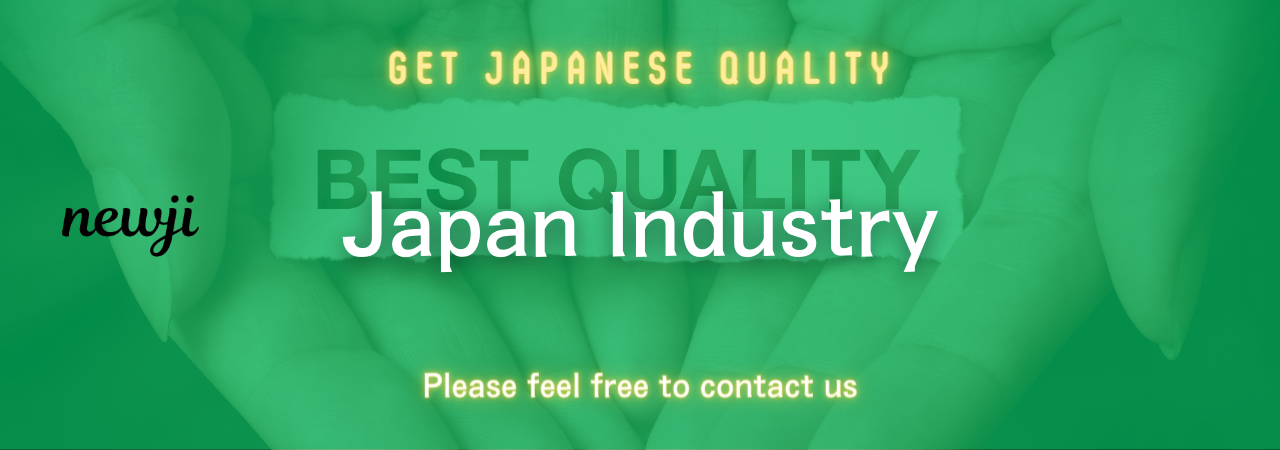
目次
Understanding Feature Engineering in Machine Learning
Feature engineering plays a crucial role in the process of developing machine learning models.
Simply put, it’s the art of selecting, modifying, and creating features to improve model performance.
Features are individual measurable properties or characteristics of data that are used to train machine learning models.
The ability to accurately engineer features can significantly impact the model’s ability to learn patterns and make predictions.
Good feature engineering can lead to enhanced model efficiency, improved accuracy, and faster convergence times during training.
In essence, it’s about providing the model with the most relevant information from the data, thus allowing it to glean insights effectively.
The Role of Feature Engineering
In machine learning, raw data often comes in a format that isn’t immediately suitable for modeling.
Feature engineering bridges the gap between raw data and meaningful information that the algorithm can learn from.
The process includes cleaning, normalization, transformation, and construction of new features.
A key aim of feature engineering is to eliminate noise in your data and ensure that features are focused on aspects relevant to the target variable.
This step is vital because irrelevant or redundant information can confuse the learning algorithm, leading to less accurate predictions.
The key to successful feature engineering is understanding the domain from which your data comes.
It requires creativity and a strong grasp of data itself, as well as an understanding of the specific machine-learning task at hand.
Common Techniques in Feature Engineering
There are numerous techniques for feature engineering, each with unique advantages depending on the data and the modeling task.
1. Feature Scaling
Feature scaling is one technique that involves adjusting the scale of features to a common level.
Methods like normalization (scaling features between 0 and 1) and standardization (transforming features to have a mean of 0 and standard deviation of 1) ensure that no single feature dominates the model due to its scale.
2. Encoding Categorical Variables
When working with categorical data, transforming these features into a numerical format is essential.
Techniques such as one-hot encoding and label encoding are used to convert categorical data into numbers the algorithm can process.
3. Feature Creation
This involves creating new features based on existing ones, aimed to enhance the learning process.
Polynomial feature creation, for instance, generates additional polynomial combinations of features, thereby capturing relationships between features.
4. Handling Missing Data
In real-world data, missing values are common.
Feature engineering tackles this through methods such as imputation, which fills in missing values using strategies like mean, median, mode, or more sophisticated techniques like predictive modeling.
5. Feature Selection
Among a plethora of available features, some will inevitably carry more significance than others.
Feature selection techniques, including wrapper methods, filter methods, and embedded methods, help in identifying and utilizing only the most relevant ones.
Application of Feature Engineering in Materials Digital Transformation (DX)
In recent years, Materials DX has emerged as an exciting area where feature engineering can significantly contribute.
Materials DX involves leveraging digital tools and data analytics to revolutionize material development and manufacturing processes.
The Relevance of Machine Learning to Materials Science
Materials science often deals with complex datasets comprised of numerous variables and interdependencies.
Leveraging machine learning in this domain can lead to groundbreaking advancements such as novel material discovery, improved material performance predictions, and optimized manufacturing processes.
Feature engineering is pivotal in this context as it aids in extracting pertinent information from raw experimental and simulation data.
Accurate feature representation can thus be translated into more reliable machine learning models for materials science.
Examples of Feature Engineering in Materials DX
1. Extracting Descriptive Features
Scientific data in materials science is usually dense and complex.
Feature engineering can distill this data into descriptive features reflecting material properties, compositions, or experimental conditions that directly influence material performance.
2. Transforming Temporal Data
Processes in material manufacturing are often time-dependent.
For instance, the curing phase of polymers or the annealing of metals is critical.
Feature engineering can transform temporal data via sequence or time-series analysis to ensure the temporal dynamics are adequately represented in models.
3. Multi-fidelity Feature Construction
Materials DX can exploit multi-fidelity data sources—from simple lab experiments to high-fidelity computer simulations.
Constructing features that effectively integrate these multi-fidelity data streams can hugely enhance model predictions and enrich insights.
Conclusion
Feature engineering remains one of the most formidable steps in the machine learning pipeline.
Its importance is evident across numerous domains, including the burgeoning field of Materials DX.
By effectively transforming and optimizing features, we enable machine learning models to learn better and predict with higher precision.
Successful feature engineering involves a delicate balance of technical prowess and creative insights, ensuring that the models built are both innovative and efficiently aligned with real-world needs.
資料ダウンロード
QCD調達購買管理クラウド「newji」は、調達購買部門で必要なQCD管理全てを備えた、現場特化型兼クラウド型の今世紀最高の購買管理システムとなります。
ユーザー登録
調達購買業務の効率化だけでなく、システムを導入することで、コスト削減や製品・資材のステータス可視化のほか、属人化していた購買情報の共有化による内部不正防止や統制にも役立ちます。
NEWJI DX
製造業に特化したデジタルトランスフォーメーション(DX)の実現を目指す請負開発型のコンサルティングサービスです。AI、iPaaS、および先端の技術を駆使して、製造プロセスの効率化、業務効率化、チームワーク強化、コスト削減、品質向上を実現します。このサービスは、製造業の課題を深く理解し、それに対する最適なデジタルソリューションを提供することで、企業が持続的な成長とイノベーションを達成できるようサポートします。
オンライン講座
製造業、主に購買・調達部門にお勤めの方々に向けた情報を配信しております。
新任の方やベテランの方、管理職を対象とした幅広いコンテンツをご用意しております。
お問い合わせ
コストダウンが利益に直結する術だと理解していても、なかなか前に進めることができない状況。そんな時は、newjiのコストダウン自動化機能で大きく利益貢献しよう!
(Β版非公開)