- お役立ち記事
- Basics of innovative experimental design using AI and points for effective data utilization
月間76,176名の
製造業ご担当者様が閲覧しています*
*2025年3月31日現在のGoogle Analyticsのデータより
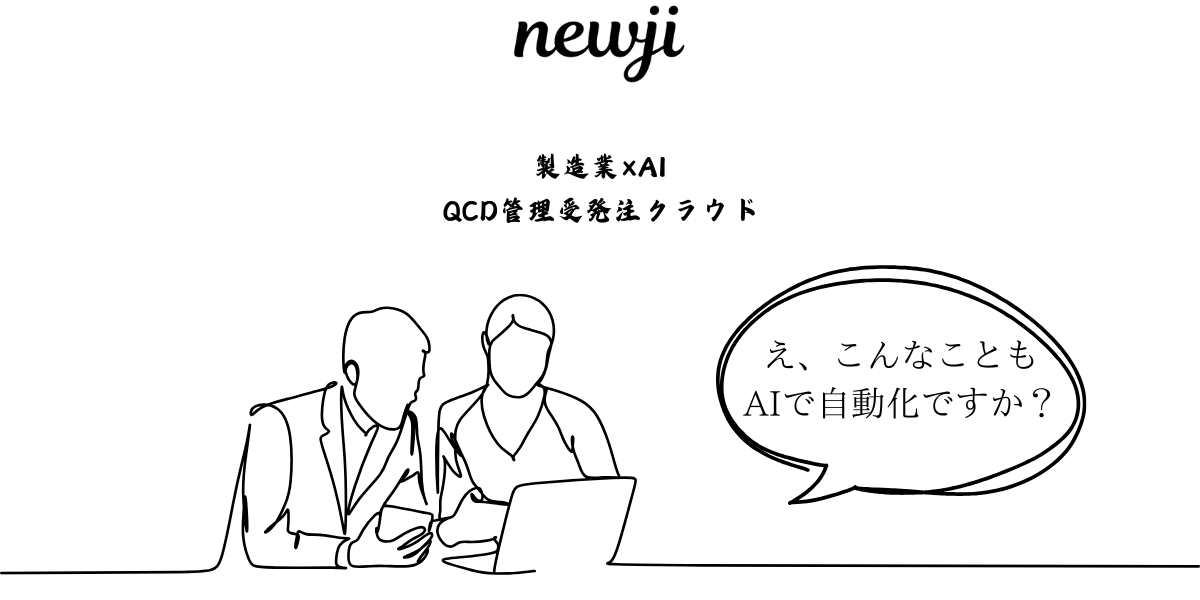
Basics of innovative experimental design using AI and points for effective data utilization
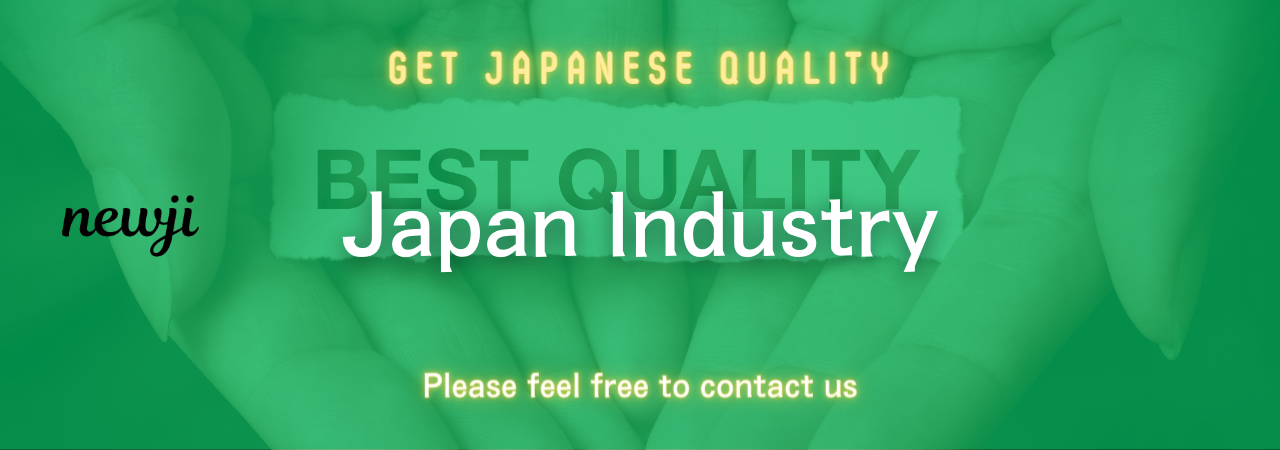
目次
Introduction to Experimental Design Using AI
Experimentation has always been a cornerstone of scientific discovery and innovation.
With the advent of Artificial Intelligence (AI), the potential to enhance experimental design has grown exponentially.
AI offers tools that not only streamline the process but also uncover patterns and insights that were previously inaccessible.
In this article, we will explore the fundamentals of using AI to innovate experimental design and effective strategies for leveraging data.
Understanding Experimental Design
Experimental design is the framework that allows researchers to conduct experiments in a systematic and structured manner.
Traditional methods involve clearly defined variables and controls, which help in identifying cause and effect relationships.
However, as problems become more complex, traditional methods may fall short in managing vast amounts of data and intricate dependencies.
The Role of AI in Experimental Design
AI can transform experimental design by automating processes, enhancing decision-making, and optimizing results.
Machine learning algorithms are particularly beneficial in detecting patterns within large datasets.
These algorithms can predict outcomes and uncover relationships between variables that would be difficult for humans to discern.
By incorporating AI into experimental design, researchers can save time, reduce costs, and improve the accuracy of their findings.
AI systems can run numerous simulations quickly, allowing researchers to test multiple hypotheses and refine experimental setups dynamically.
Types of AI Used in Experimental Design
There are several types of AI technologies that can be integrated into experimental design:
– **Machine Learning (ML):** ML algorithms are used to analyze data and make predictions based on historical data.
They are particularly useful in optimizing processes and identifying potential experimental outcomes.
– **Neural Networks:** These are used to model complex patterns and relationships within data.
They mimic the human brain’s network and are incredibly powerful for image and speech recognition tasks.
– **Natural Language Processing (NLP):** This technology enables machines to understand and interpret human language.
It can be employed to process and analyze textual data, which is valuable for experiments involving large volumes of documents or literature reviews.
Benefits of AI in Experimental Design
Using AI in experimental design offers several advantages:
– **Efficiency:** AI can streamline workflows by handling repetitive tasks and large data analyses more efficiently than humans.
– **Precision:** Enhanced data analysis capabilities allow for more precise experimentation, with less human error.
– **Adaptability:** AI can adapt and refine experiment parameters in real-time, responding to data input without the need for manual intervention.
– **Innovation:** AI fosters innovation by enabling researchers to explore new variables and hypotheses that might not have been considered otherwise.
Challenges in Implementing AI for Experimental Design
While the benefits are numerous, implementing AI in experimental design comes with its challenges.
For instance, there is a steep learning curve associated with understanding and correctly applying AI technologies.
Additionally, the quality of data is crucial; AI systems require clean, well-structured data to function effectively.
Another challenge lies in integrating AI solutions with existing experimental setups and ensuring they are flexible enough to handle unforeseen changes in the experimental environment.
Effective Data Utilization in AI-Driven Experiments
Data is the lifeblood of AI-driven experimental design.
To utilize data effectively, researchers should consider the following:
Ensuring Data Quality
High-quality data is essential for the success of AI experiments.
This involves:
– **Data Cleaning:** Removing errors, inconsistencies, and duplicates from datasets to ensure accuracy.
– **Data Normalization:** Standardizing data formats for consistency, aiding analysis, and reducing bias.
Data Integration
Combining data from various sources can enrich an experiment and provide more comprehensive insights.
Researchers should aim to integrate diverse data types (e.g., quantitative, qualitative) to enhance AI’s predictive capabilities.
Iterative Data Analysis and Feedback
AI thrives on feedback loops.
Iterative data analysis allows researchers to refine their experiments continuously.
By assessing results and feeding them back into the AI system, experiments can evolve and improve over time.
Conclusion: The Future of Experimental Design with AI
The integration of AI into experimental design marks a significant evolution in how experiments are conducted.
While challenges remain, the potential for AI to enhance and innovate research methodologies is vast.
As AI technology continues to advance, we can expect even more sophisticated tools that further improve experimental accuracy, efficiency, and creativity.
Instruments that harness AI will become indispensable in the pursuit of scientific and technological breakthroughs.
By leveraging AI’s capabilities, researchers can push the boundaries of knowledge and develop solutions to complex challenges that were previously thought insurmountable.
資料ダウンロード
QCD管理受発注クラウド「newji」は、受発注部門で必要なQCD管理全てを備えた、現場特化型兼クラウド型の今世紀最高の受発注管理システムとなります。
ユーザー登録
受発注業務の効率化だけでなく、システムを導入することで、コスト削減や製品・資材のステータス可視化のほか、属人化していた受発注情報の共有化による内部不正防止や統制にも役立ちます。
NEWJI DX
製造業に特化したデジタルトランスフォーメーション(DX)の実現を目指す請負開発型のコンサルティングサービスです。AI、iPaaS、および先端の技術を駆使して、製造プロセスの効率化、業務効率化、チームワーク強化、コスト削減、品質向上を実現します。このサービスは、製造業の課題を深く理解し、それに対する最適なデジタルソリューションを提供することで、企業が持続的な成長とイノベーションを達成できるようサポートします。
製造業ニュース解説
製造業、主に購買・調達部門にお勤めの方々に向けた情報を配信しております。
新任の方やベテランの方、管理職を対象とした幅広いコンテンツをご用意しております。
お問い合わせ
コストダウンが利益に直結する術だと理解していても、なかなか前に進めることができない状況。そんな時は、newjiのコストダウン自動化機能で大きく利益貢献しよう!
(β版非公開)