- お役立ち記事
- Basics of irregular signal processing technology and its application to noise removal and identification combined with machine learning
Basics of irregular signal processing technology and its application to noise removal and identification combined with machine learning
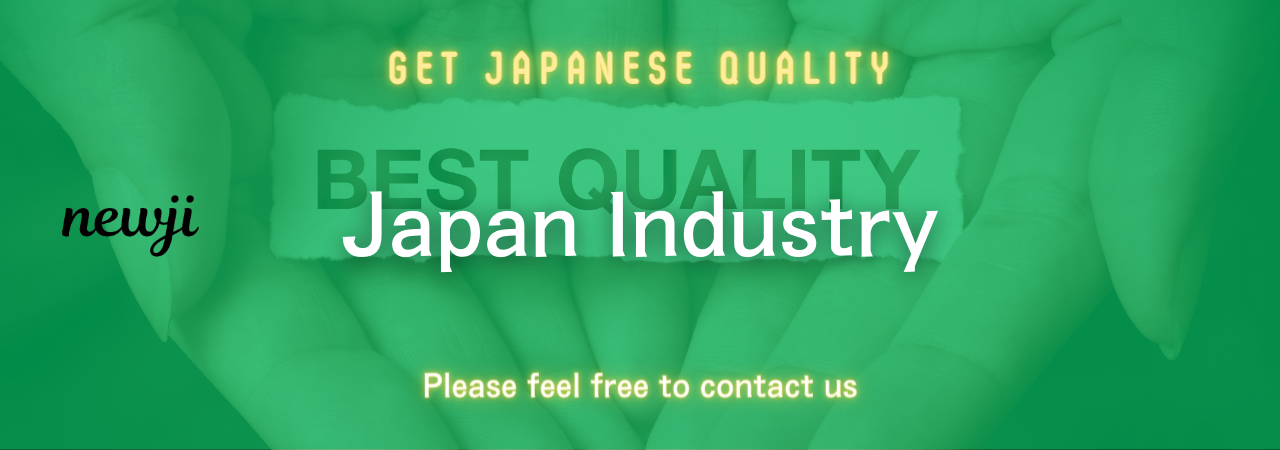
目次
Understanding Irregular Signal Processing
Irregular signal processing is a method used to manage and analyze signals that don’t conform to regular, predictable patterns.
These signals, often referred to as ‘non-stationary’, are challenging to process because their statistical properties change over time.
Understanding these signals is crucial in various fields, such as telecommunications, seismology, and medical diagnostics.
In essence, irregular signal processing involves the extraction of useful information from complex signals.
These signals can include anything from speech and audio signals to ECGs and other biosignals.
This processing is vital for identifying underlying patterns and making accurate predictions.
The Role of Machine Learning
Machine learning, a subset of artificial intelligence, has significantly enhanced the capabilities of irregular signal processing.
It offers advanced algorithms that can learn patterns and make decisions based on the data input.
Machine learning algorithms are particularly effective in identifying noise and differentiating it from the actual signal in data.
By employing techniques such as neural networks and decision trees, machine learning can automate the identification of irregular signals.
This reduces the need for manual interventions, speeds up the processing, and increases accuracy.
With machine learning, systems can adapt to new changes in signals over time, improving their performance with continuous learning.
Applications in Noise Removal
A common challenge in signal processing is noise interference.
Noise can distort the signal, making it difficult to extract valuable information.
Irregular signal processing, coupled with machine learning, can effectively remove noise from signals.
Noise removal involves filtering out unwanted components while retaining the important signal.
Techniques like spectral subtraction, Wiener filtering, and wavelet-based denoising are commonly used.
Machine learning enhances these techniques by learning from the patterns of noise and optimizing the filtering process.
For instance, in telecommunications, noise removal is essential for ensuring clear voice transmission.
In medical diagnostics, it helps in obtaining accurate readings from ECG or EEG signals by eliminating high-frequency noise.
Identification and Classification
Beyond noise removal, irregular signal processing is crucial for signal identification and classification.
Machine learning algorithms can be trained to recognize various classes of signals based on historical data.
This is particularly useful in areas like fraud detection, weather forecasting, and even stock market analysis.
For example, in fraud detection systems, machine learning algorithms analyze transaction patterns.
They learn to differentiate between normal and irregular transactions, identifying potential fraudulent activities.
Similarly, seismologists use these techniques to classify earthquake signals, distinguishing them from other seismic activities.
This classification capability is powerful, offering a proactive approach to analyzing irregular signals.
It allows industries to move from reactive to predictive models, opening new avenues for innovation.
Challenges and Future Prospects
Despite the numerous advantages, irregular signal processing combined with machine learning faces several challenges.
The process involves handling large volumes of data, which requires substantial computational power.
Additionally, developing accurate models demands high-quality training data.
Another significant challenge is the handling of real-time data.
Processing signals in real-time for applications like autonomous vehicles or real-time video analysis requires efficient algorithms and robust systems.
Looking forward, the integration of advanced technologies such as quantum computing may address some of these challenges.
Quantum computing could potentially revolutionize the speed and capabilities of processing irregular signals.
Moreover, as machine learning continues to evolve, its algorithms are expected to become more sophisticated.
This would lead to more precise noise removal, better classification, and ultimately, the extraction of more intelligence from irregular signals.
Conclusion
Irregular signal processing technology, combined with machine learning, offers a powerful tool for extracting and interpreting complex signals.
It provides solutions for noise removal, signal identification, and classification, applicable across many fields.
While challenges exist, ongoing technological advancements offer promising opportunities for enhancing these processes.
As machine learning techniques advance, they will likely introduce more robust and efficient ways to tackle irregular signal processing challenges.
This progression holds exciting prospects for industries, promising improved efficiencies and new capabilities.
資料ダウンロード
QCD調達購買管理クラウド「newji」は、調達購買部門で必要なQCD管理全てを備えた、現場特化型兼クラウド型の今世紀最高の購買管理システムとなります。
ユーザー登録
調達購買業務の効率化だけでなく、システムを導入することで、コスト削減や製品・資材のステータス可視化のほか、属人化していた購買情報の共有化による内部不正防止や統制にも役立ちます。
NEWJI DX
製造業に特化したデジタルトランスフォーメーション(DX)の実現を目指す請負開発型のコンサルティングサービスです。AI、iPaaS、および先端の技術を駆使して、製造プロセスの効率化、業務効率化、チームワーク強化、コスト削減、品質向上を実現します。このサービスは、製造業の課題を深く理解し、それに対する最適なデジタルソリューションを提供することで、企業が持続的な成長とイノベーションを達成できるようサポートします。
オンライン講座
製造業、主に購買・調達部門にお勤めの方々に向けた情報を配信しております。
新任の方やベテランの方、管理職を対象とした幅広いコンテンツをご用意しております。
お問い合わせ
コストダウンが利益に直結する術だと理解していても、なかなか前に進めることができない状況。そんな時は、newjiのコストダウン自動化機能で大きく利益貢献しよう!
(Β版非公開)