- お役立ち記事
- Basics of machine learning using Python and its application to effective prediction, estimation, and detection
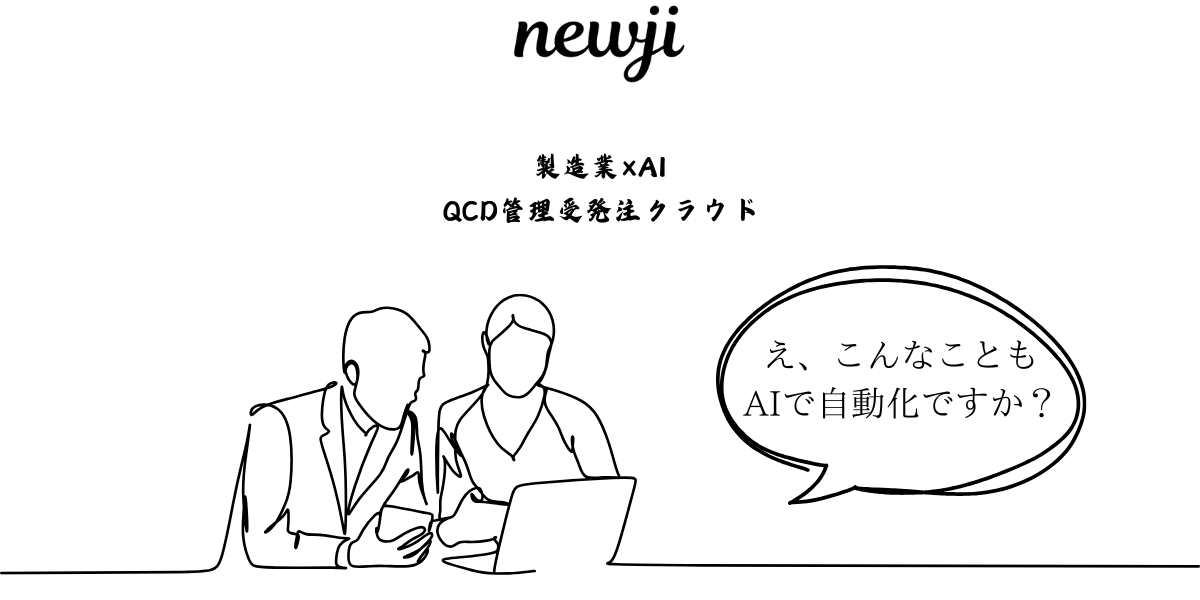
Basics of machine learning using Python and its application to effective prediction, estimation, and detection
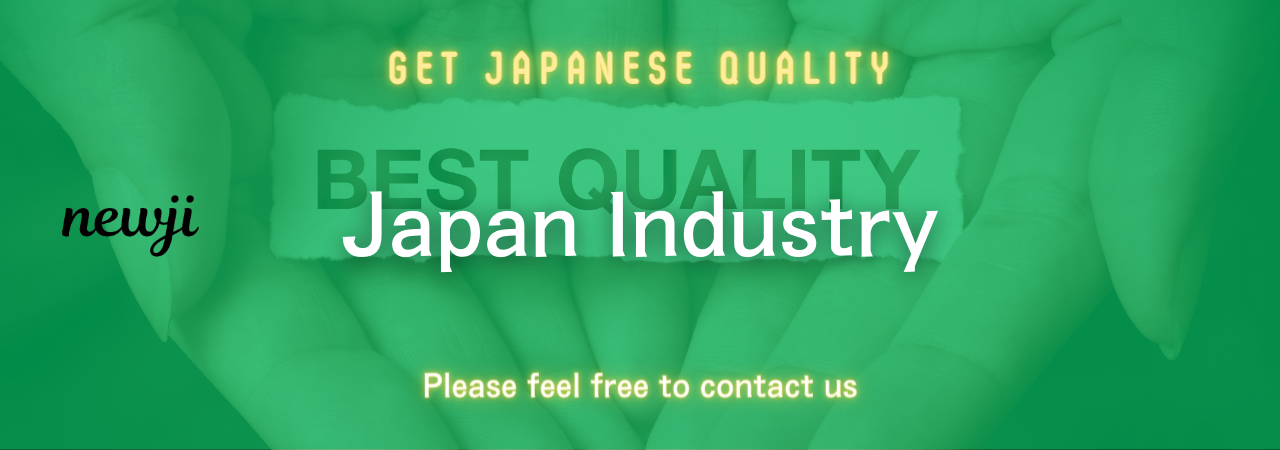
目次
Introduction to Machine Learning with Python
Machine learning has become an integral part of modern technology, assisting in the prediction, estimation, and detection of patterns in various fields.
Python, with its simplicity and vast library support, is a preferred language for implementing machine learning algorithms.
This article will guide you through the basics of machine learning using Python and illustrate how it can be applied to achieve effective results.
Understanding Machine Learning
Machine learning is a subset of artificial intelligence that involves training computer systems to learn from data and improve over time without being explicitly programmed.
It enables computers to make predictions or decisions based on past experiences represented by data.
Machine learning can be broadly categorized into three types: supervised learning, unsupervised learning, and reinforcement learning.
Supervised Learning
Supervised learning is where the model is trained on a labeled dataset, meaning each data point has an associated output or label.
The goal is for the model to learn the mapping between inputs and outputs so that it can accurately predict the output for new, unseen data.
Common algorithms for supervised learning include Linear Regression, Decision Trees, and Support Vector Machines.
Unsupervised Learning
In unsupervised learning, the data used to train the model does not come with labels.
The objective is to find hidden patterns or intrinsic structures in the input data.
Clustering and Association are the two most popular types of unsupervised learning.
K-Means and Hierarchical clustering are examples of algorithms used in unsupervised learning.
Reinforcement Learning
Reinforcement learning involves training a model to make sequences of decisions by rewarding desired actions or behaviors.
The model learns to achieve its goal by interacting with an environment and receiving feedback in the form of rewards or penalties.
This type of learning is commonly used in robotics and gaming.
Getting Started with Python for Machine Learning
Python is the language of choice for many machine learning practitioners due to its easy-to-understand syntax and a multitude of powerful libraries.
Before diving into machine learning tasks, it is essential to have Python installed along with some key libraries like NumPy, Pandas, and Scikit-learn.
Installing Python and Libraries
To begin, download and install Python from its official website.
Once installed, you can make use of package managers like pip to install essential libraries.
For example, you can install NumPy using the command `pip install numpy`, Pandas with `pip install pandas`, and Scikit-learn with `pip install scikit-learn`.
Using NumPy and Pandas
NumPy is a library for numerical computing in Python.
It provides support for arrays, and matrices, and comes with a collection of mathematical functions.
Pandas, on the other hand, is used for data manipulation and analysis, making it easier to load, clean, and preprocess data before it is fed into a machine learning model.
Building a Simple Machine Learning Model
Let’s explore how to create a simple supervised learning model using Python and Scikit-learn.
We’ll use a Linear Regression model for a basic prediction task.
Data Preparation
First, you’ll need a dataset.
If you’re just getting started, you might consider using Scikit-learn’s built-in datasets such as the Boston housing dataset.
Load the dataset using Pandas and split it into training and testing sets.
“`python
from sklearn.datasets import load_boston
from sklearn.model_selection import train_test_split
import pandas as pd
boston = load_boston()
df = pd.DataFrame(boston.data, columns=boston.feature_names)
df[‘PRICE’] = boston.target
X = df.drop(‘PRICE’, axis=1)
y = df[‘PRICE’]
X_train, X_test, y_train, y_test = train_test_split(X, y, test_size=0.2, random_state=42)
“`
Training the Model
Define and train a Linear Regression model using the training data.
Scikit-learn makes it simple with its easy-to-use API.
“`python
from sklearn.linear_model import LinearRegression
model = LinearRegression()
model.fit(X_train, y_train)
“`
Testing the Model
Once the model is trained, evaluate its performance on the test data using metrics like Mean Absolute Error (MAE) or Mean Squared Error (MSE).
“`python
from sklearn.metrics import mean_squared_error
predictions = model.predict(X_test)
mse = mean_squared_error(y_test, predictions)
print(f’Mean Squared Error: {mse}’)
“`
Practical Applications of Machine Learning
Machine learning finds applications across diverse domains due to its predictive capabilities.
Image and Speech Recognition
Machine learning models are extensively used in facial recognition systems and voice assistants.
Convolutional Neural Networks (CNN) and Recurrent Neural Networks (RNN) are popular architectures for these tasks.
Healthcare
In healthcare, machine learning models help in diagnosis, treatment personalization, and predicting patient outcomes.
For example, models can analyze medical images like X-rays for abnormalities or predict disease progression by analyzing patient history data.
Financial Services
Machine learning aids in risk management and fraud detection in the finance sector.
Through analyzing transaction histories and learning patterns, algorithms can flag potentially fraudulent activities effectively.
Conclusion
Machine learning using Python is a powerful tool that organizations leverage to gain insights and drive innovation.
With a fundamental understanding of machine learning concepts and proficiency in Python, you can develop models for a wide array of applications.
Start with basic models like Linear Regression and gradually explore more complex algorithms as you dive deeper into this fascinating field.
資料ダウンロード
QCD調達購買管理クラウド「newji」は、調達購買部門で必要なQCD管理全てを備えた、現場特化型兼クラウド型の今世紀最高の購買管理システムとなります。
ユーザー登録
調達購買業務の効率化だけでなく、システムを導入することで、コスト削減や製品・資材のステータス可視化のほか、属人化していた購買情報の共有化による内部不正防止や統制にも役立ちます。
NEWJI DX
製造業に特化したデジタルトランスフォーメーション(DX)の実現を目指す請負開発型のコンサルティングサービスです。AI、iPaaS、および先端の技術を駆使して、製造プロセスの効率化、業務効率化、チームワーク強化、コスト削減、品質向上を実現します。このサービスは、製造業の課題を深く理解し、それに対する最適なデジタルソリューションを提供することで、企業が持続的な成長とイノベーションを達成できるようサポートします。
オンライン講座
製造業、主に購買・調達部門にお勤めの方々に向けた情報を配信しております。
新任の方やベテランの方、管理職を対象とした幅広いコンテンツをご用意しております。
お問い合わせ
コストダウンが利益に直結する術だと理解していても、なかなか前に進めることができない状況。そんな時は、newjiのコストダウン自動化機能で大きく利益貢献しよう!
(Β版非公開)