- お役立ち記事
- Basics of materials informatics and points for using data science to deal with small amounts of data
Basics of materials informatics and points for using data science to deal with small amounts of data
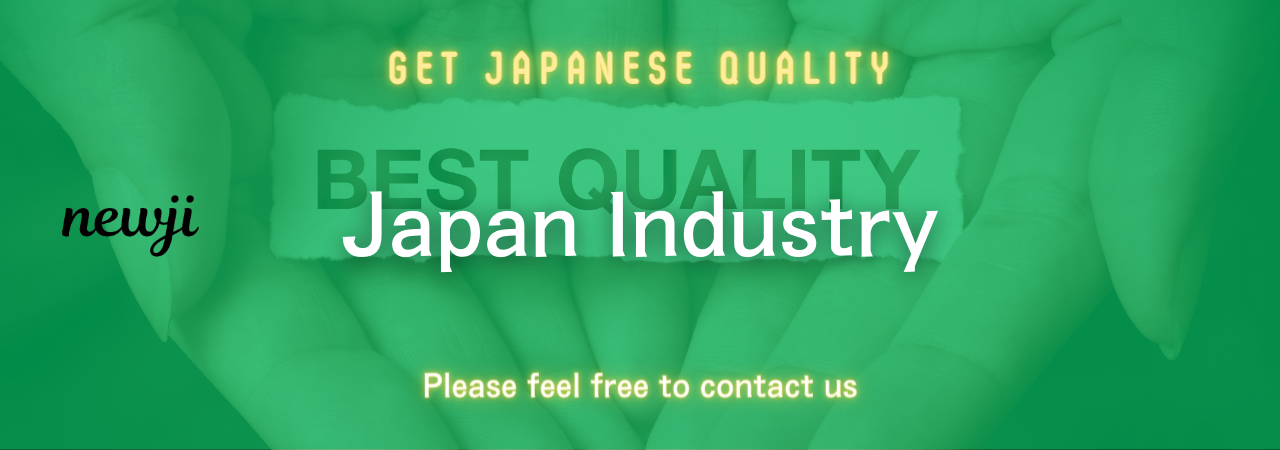
目次
Understanding Materials Informatics
Materials informatics is a rapidly growing field that combines material science with data science techniques to accelerate the discovery, design, and deployment of new materials.
At its core, it leverages computational tools and algorithms to process and analyze vast sets of material data.
The primary goal of materials informatics is to predict the properties and behaviors of materials based on their composition and structure.
By using data-driven approaches, scientists and engineers can bypass the traditional trial-and-error methods, significantly speeding up the development cycles for new materials.
Importance in Modern Material Science
In today’s fast-paced world, the demand for novel materials is at an all-time high.
From lightweight materials for aerospace applications to sustainable energy solutions, there is a constant need to innovate and improve material performance.
Materials informatics provides a strategic advantage by offering insights that might not be observable through conventional methods.
The application of machine learning in materials science enables the creation of predictive models.
These models can explore an enormous space of potential material compositions, offering suggestions for promising new materials that may meet desired criteria.
Data Science and Material Data
Data science plays a crucial role in materials informatics by providing the methods and tools needed to handle complex datasets.
In material science, data can originate from a variety of sources, such as experiments, simulations, and literature.
The quality and amount of data available are fundamental to building reliable predictive models.
Handling Small Data Sets
One of the challenges in materials informatics is dealing with small amounts of data.
Unlike fields such as image recognition or language processing, material datasets can be limited due to the high costs and complexity associated with material experimentation and characterization.
However, there are effective strategies to overcome this limitation.
When dealing with limited data, data augmentation techniques can be applied to expand the dataset.
This might include generating synthetic data through simulations or variations of existing measurements.
Furthermore, transfer learning is a powerful method where pre-trained models in related domains are adapted to the material-specific tasks.
Another critical aspect is feature engineering, where domain knowledge is applied to create meaningful features from raw data.
This process can enhance the predictive ability of models, even when starting with a small dataset.
The Role of Machine Learning
Machine learning algorithms are integral to materials informatics, providing the capability to recognize patterns and correlations in material data.
Supervised learning techniques, such as regression and classification, are commonly used to predict material properties.
Applications of Machine Learning
In materials informatics, supervised models are used to predict outcomes based on input variables.
For example, regression models can forecast the mechanical strength of materials given their composite elements.
Classification algorithms can determine the categorical property, such as whether a material is a conductor or an insulator.
Unsupervised learning methods, like clustering and dimensionality reduction, are also valuable.
These techniques help in identifying intrinsic structures in the data, categorizing materials into similar groups, and reducing the complexity of datasets while retaining essential characteristics.
Reinforcement learning, a more advanced machine learning approach, shows promise in areas such as autonomous experimentation.
Here, algorithms can iteratively design and test materials, learning from the outcomes to refine their predictions further.
Implementing a Materials Informatics Strategy
For organizations looking to incorporate materials informatics into their R&D processes, starting with a well-defined strategy is crucial.
A successful implementation relies on establishing clear objectives, whether that be improving existing materials, discovering new ones, or enhancing production efficiencies.
Setting Up for Success
Begin by addressing data collection and management.
Having a structured database where material properties and historical data are stored in an accessible format is key.
Data cleaning and preparation are equally important to ensure high-resolution input for machine learning models.
Choosing the right computational tools and software platforms is also a critical step.
There are a variety of open-source and proprietary tools available that provide powerful frameworks for combining material science with data analytics.
Furthermore, interdisciplinary collaboration should be encouraged.
Materials scientists, data scientists, and engineers must work together to foster innovation and maintain alignment with the overall goals of the project.
Conclusion
Materials informatics stands at the forefront of revolutionizing how new materials are discovered and deployed.
By effectively harnessing data science techniques, even with limited data, researchers and companies can gain valuable insights that lead to groundbreaking advancements in materials science.
As the field continues to evolve, those who integrate materials informatics into their operations stand to achieve significant competitive advantages, paving the way for innovative products and solutions that meet the complex demands of the modern world.
資料ダウンロード
QCD調達購買管理クラウド「newji」は、調達購買部門で必要なQCD管理全てを備えた、現場特化型兼クラウド型の今世紀最高の購買管理システムとなります。
ユーザー登録
調達購買業務の効率化だけでなく、システムを導入することで、コスト削減や製品・資材のステータス可視化のほか、属人化していた購買情報の共有化による内部不正防止や統制にも役立ちます。
NEWJI DX
製造業に特化したデジタルトランスフォーメーション(DX)の実現を目指す請負開発型のコンサルティングサービスです。AI、iPaaS、および先端の技術を駆使して、製造プロセスの効率化、業務効率化、チームワーク強化、コスト削減、品質向上を実現します。このサービスは、製造業の課題を深く理解し、それに対する最適なデジタルソリューションを提供することで、企業が持続的な成長とイノベーションを達成できるようサポートします。
オンライン講座
製造業、主に購買・調達部門にお勤めの方々に向けた情報を配信しております。
新任の方やベテランの方、管理職を対象とした幅広いコンテンツをご用意しております。
お問い合わせ
コストダウンが利益に直結する術だと理解していても、なかなか前に進めることができない状況。そんな時は、newjiのコストダウン自動化機能で大きく利益貢献しよう!
(Β版非公開)